Analytical and Bioanalytical Chemistry ( IF 3.8 ) Pub Date : 2018-02-24 , DOI: 10.1007/s00216-018-0947-4 Yitao Li , Mengci Li , Wei Jia , Yan Ni , Tianlu Chen
It is well recognized that physiological and environmental factors such as race, age, gender, and diurnal cycles often have a definite influence on metabolic results that statistically manifests as confounding variables. Currently, removal or controlling of confounding effects relies heavily on experimental design. There are no available data processing techniques focusing on the compensation of their effects. We therefore proposed a new method, Metabolic confounding effect elimination (MCEE), to remove the influence of specified confounding factors and make the data more accurate. The method consists of three steps: metabolites grouping, confounder-related metabolites selection, and metabolites modification. Its effectiveness and advantages were evaluated comprehensively by several simulated models and real datasets, and were compared with two typical methods, the principal component analysis (PCA)- and the direct orthogonal signal correction (DOSC)-based methods. MCEE is simple, effective, and safe, and is independent of sample number, association degree, and missing value. Hence, it may serve as a good complement to existing metabolomics data preprocessing methods and aid in better understanding the metabolic and biological status of interest.
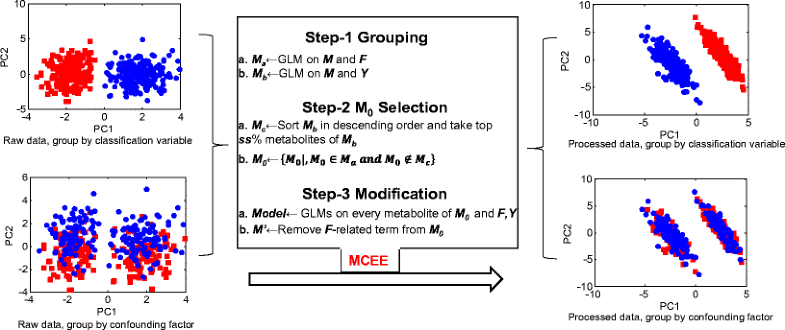
Algorithm flow and demo performance of MCEE
中文翻译:

MCEE:消除代谢混杂效应的数据预处理方法
众所周知,生理和环境因素,例如种族,年龄,性别和昼夜周期,通常对代谢结果有确定的影响,统计学上表现为混杂变量。当前,消除或控制混杂效应在很大程度上取决于实验设计。没有可用的数据处理技术来关注其效果的补偿。因此,我们提出了一种新方法,即代谢混杂效应消除(MCEE),以消除指定混杂因子的影响,并使数据更准确。该方法包括三个步骤:代谢物分组,与混杂物有关的代谢物选择和代谢物修饰。通过几个模拟模型和真实数据集对它的有效性和优势进行了全面评估,并与两种典型方法进行了比较:基于主成分分析(PCA)的方法和基于直接正交信号校正(DOSC)的方法。MCEE简单,有效和安全,并且与样本数,关联度和缺失值无关。因此,它可以作为现有代谢组学数据预处理方法的良好补充,并有助于更好地了解目标代谢和生物学状态。
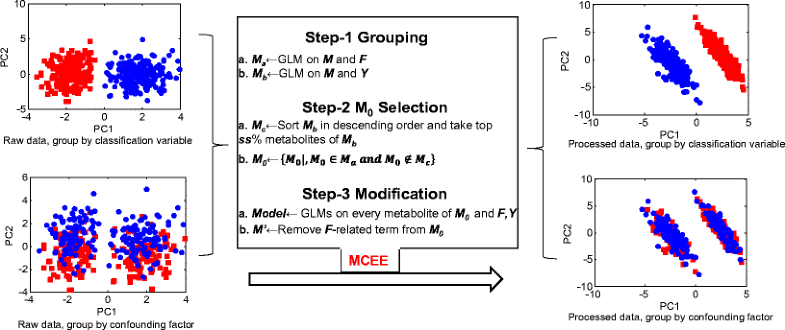
MCEE的算法流程和演示性能