Ischemic Heart Disease Selectively Modifies the Right Atrial Appendage Transcriptome
- 1Department of Pharmacology, Faculty of Medicine, University of Helsinki, Helsinki, Finland
- 2Heart and Lung Center, University of Helsinki and Helsinki University Hospital, Helsinki, Finland
- 3Department of Health Informatics, Graduate School of Informatics, Middle East Technical University (METU), Ankara, Turkey
- 4Department of Oral and Maxillofacial Diseases, Faculty of Medicine, University of Helsinki, Helsinki, Finland
- 5Research Programs Unit, University of Helsinki, Helsinki, Finland
- 6Department of Cardiothoracic Surgery, Heart Center, Tampere University Hospital, Tampere, Finland
- 7Faculty of Medicine and Health Technology, Tampere University, Tampere, Finland
- 8Department of Chemical and Biological Engineering, College of Engineering, Koc University, Istanbul, Turkey
- 9School of Medicine, Koc University, Istanbul, Turkey
Background: Although many pathological changes have been associated with ischemic heart disease (IHD), molecular-level alterations specific to the ischemic myocardium and their potential to reflect disease severity or therapeutic outcome remain unclear. Currently, diagnosis occurs relatively late and evaluating disease severity is largely based on clinical symptoms, various imaging modalities, or the determination of risk factors. This study aims to identify IHD-associated signature RNAs from the atrial myocardium and evaluate their ability to reflect disease severity or cardiac surgery outcomes.
Methods and Results: We collected right atrial appendage (RAA) biopsies from 40 patients with invasive coronary angiography (ICA)-positive IHD undergoing coronary artery bypass surgery and from 8 patients ICA-negative for IHD (non-IHD) undergoing valvular surgery. Following RNA sequencing, RAA transcriptomes were analyzed against 429 donors from the GTEx project without cardiac disease. The IHD transcriptome was characterized by repressed RNA expression in pathways for cell–cell contacts and mitochondrial dysfunction. Increased expressions of the CSRNP3, FUT10, SHD, NAV2-AS4, and hsa-mir-181 genes resulted in significance with the complexity of coronary artery obstructions or correlated with a functional cardiac benefit from bypass surgery.
Conclusions: Our results provide an atrial myocardium-focused insight into IHD signature RNAs. The specific gene expression changes characterized here, pave the way for future disease mechanism-based identification of biomarkers for early detection and treatment of IHD.
Introduction
The early diagnosis of ischemic heart disease (IHD) could facilitate the design of therapeutic strategies and, thus, improve patient prognosis (1). To this end, IHD biomarkers which specifically and sensitively correlate with disease severity are required (2). Currently, initial assessment of IHD is largely based on the patient's clinical symptoms, distinct electrocardiographic (ECG) alterations, and risk scoring. Diagnosis depends on cardiac stress testing and radiographic evaluations of coronary vessel obstructions or myocardial perfusion (3, 4). These are supplemented with laboratory investigations (5). These surrogate markers, however, do not directly reflect the myocardial structure, metabolism, or inflammation (6). We propose that the right atrial appendage (RAA), safely sampled during heart surgery, can provide direct insight into the pathophysiological myocardial changes manifesting in IHD. For the further discovery of IHD biomarkers, it is crucial to understand these changes and to utilize them for laying the rational pathology-based groundwork in molecular detail. Toward this, we collected 48 RAA biopsies from patients with or without IHD, and compared their RNA-sequenced (RNAseq) transcriptomes to those from RAA biopsies from 429 donors without heart disease available from the Genotype Tissue Expression (GTEx) project. In doing so, we establish RAA as a feasible source for the functional and metabolic characterization of cardiac ischemic pathologies, and show that specific RAA gene expressions correlate with IHD complexity and the functional benefit of cardiac surgery.
Materials and Methods
Ethics
This study was approved by the Operative Ethics Committee of the Hospital District of Helsinki and Uusimaa (DNro 286/13/03/02/2012). The study was registered to the ISRCTN registry (ISRCTN15411573). Before surgery, all patients provided their written informed consent and received treatment adhering to evidence-based clinical guidelines as routinely adopted by healthcare centers.
Patients and Samples
For this study, we recruited a total of 54 patients scheduled to undergo elective cardiac surgery between 2014 and 2015 at the Heart and Lung Center at Helsinki University Central Hospital (Helsinki, Finland) or Tampere Heart Hospital, Tampere University Hospital (Tampere, Finland). We assigned 48 patients to the RAA RNA sequencing arm and 6 patients to the RNA sequencing data validation arm. Within the RNA sequencing arm, 35 patients (35/48, 73%) underwent CABG surgery, 8 patients (8/48, 17%) underwent aortic valve surgery, and combined CABG and aortic valve surgery was performed on 5 patients (5/48, 10%). According to invasive coronary angiography (ICA), patients were categorized into IHD or non-IHD groups. The IHD group (n = 40) included patients with ICA occlusions, and contained patients who underwent CABG or combined surgery. The non-IHD group (n = 8) included patients with no ICA occlusions, and containing patients who underwent isolated valvular surgery. All 6 patients in the validation arm underwent CABG surgery and had ICA occlusions. We used RAA RNAseq transcriptomes from donors (n = 429) without heart disease from the GTEx biobank as the controls. Causes of deaths among GTEx control patients were classified according to the four-point Hardy Scale in order to exclude cases with a cardiac background. Detailed patient demographic characteristics are provided in Supplementary Table 1.
During cardiac surgery, a biopsy sample of the RAA was collected from each patient. Tissue samples were collected at the cannulation site, the insertion site of the venous line for the heart-lung machine. Dissected biopsies were briefly washed in ice-cold sterile saline, cut into 0.5 cm-thick tissue pieces, and immersed into 3 ml of RNAlater (Sigma Aldrich, Merck KGaA, Darmstadt, Germany) to inactivate RNAses and stabilize RNA. Samples were left to stabilize at +4°C for 1 day and were thereafter stored at −70°C or below until processing. The RAA tissues of the validation arm patients were collected into formalin for fixation at +4°C for 14 days, followed by storage in 70% ethanol until processing for paraffin embedding.
Total RNA was isolated from RAA biopsies via initial ice-cold tissue homogenization using a Precellys 24 tissue homogenizer equipped with the Cryolys cooling option (Bertin Technologies SAS, Montigny-le-Bretonneux, France) followed by RNA isolation using combined QIAzol/chloroform extraction and Qiagen RNeasy mini spin column purification according to the manufacturer's instructions (Qiagen, Venlo, Netherlands). The sample RNA integrity number (RIN; mean 7.95 ± 0.55; range 7.1–9.0) and total quantity (mean 3069.55 ± 1141.08 ng; range 867.8–6855.0 ng) were evaluated in the Functional Genomics Unit (FuGU, University of Helsinki, Helsinki, Finland). Isolated total RNA was stored at −70°C or below until sequencing. RNA sequencing was performed by the BGI Group (Copenhagen, Denmark). Ribosomal RNA was removed using the Ribo-ZeroTM Magnetic kit (Illumina Inc., San Diego, CA), and the samples were sequenced using the BGI LncRNA-seq protocol with Illumina HiSeq 4000 technology (100PE, BGI Group).
Collection of Patient Data
Cardiovascular disease and treatment-related clinical data were collected from electronic healthcare records. Missing values were retrieved and reanalyzed from the stored raw sequences, including those from echocardiography, electrocardiography, and angiography. Drug treatment data from the hospital databases were meticulously recorded from 6-month pre- and post-operative periods. To enable drug treatment comparisons, we used the InnoLIMS Medical software (Innovatics Oy, Helsinki, Finland) to categorize and standardize each treatment according to its classification in the Anatomical Therapeutic Chemical (ATC) classification system and by converting each daily dosing regimen to the corresponding defined daily dose (DDD, Finnish Medicines Agency, FIMEA, Helsinki, Finland and the World Health Organization Collaborating Center for Drug Statistics Methodology, Oslo, Norway). To capture patients' stable medication regimens unrelated to hospitalization, treatments were recorded at 3 weeks preoperatively and 6 months post-operatively.
Data Analysis of RNA-Sequenced Data
Fastq files were trimmed using Trimmomatic v0.32 (7) applying the following parameters: ILLUMINACLIP: < fasta with adapters>:2:30:10 LEADING:3 TRAILING:3 SLIDINGWINDOW:4:15 MINLEN:36. Trimmed reads were mapped to the reference genome (EnsEMBL gene collection v82) (8) using STAR v2.3.0 (9) with the following parameters: –genomeLoad NoSharedMemory –readFilesCommand zcat –outSAMattributes All –outSAMunmapped Within. For read quantification, we used featureCounts (10) with the following parameters: “-F” “GTF” “-t” “exon” “-g” “gene_id” “-O” “-T” “16” “-a.” Gene counts from 429 healthy tissue samples were obtained from the GTEx analysis release V8. For subsequent analyses, only genes with an average logCPM >1 were considered. We used R bioconductor's package edgeR (11) to normalize the read counts and test for differentially expressed genes. We performed upper quartile normalization on the read counts, which were fitted to a quasi-likelihood negative binomial generalized log-linear model. In addition, we performed the quasi-likelihood F-test using the glmQLFit and glmQLFtest functions. Finally, differentially expressed genes were selected applying |logFC| >2 and FDR <0.05.
The RNA sequencing data is available from the Gene Expression Omnibus database repository under the identification GSE173594, https://www.ncbi.nlm.nih.gov/geo/query/acc.cgi?acc=GSE173594.
Functional Enrichment Analysis
We carried out functional enrichment analyses using IPA software (Qiagen). Enriched pathways were selected using FDR <0.05 for differentially expressed genes from ischemic, non-ischemic, and healthy tissue samples. For the miRNA targets, we selected enriched pathways applying p < 0.05. The functional annotation of genes significantly downregulated or upregulated across surgery benefit groups was extracted separately from the diseases, and the functions tab for IPA, enriched diseases, and functions were selected by excluding cancer-related results and applying FDR <0.05.
mIRNA Targets
miRNA targets were identified using Targetscan 7.2 (12). Targetscan calculates context++ score for the complementary site of miRNA by summing contributions from 14 features. Some of the features are site type, 3′ UTR length, and the target site abundance. The total context++ score is calculated for a representative transcript by summing the context++ scores for the sites to the representative miRNA. Many of the targets for a specific miRNA are false positives in the Targetscan, requiring a cutoff. Thus, targets were extracted using a cutoff total context++ score <-0.8. We found that with a less stringent cutoff, the number of targets increased greatly. This specific cutoff was selected to extract high confidence targets.
Quantitative and Qualitative Patient Categorizations
In order to quantitatively evaluate the complexity of coronary artery obstructions, preoperative SYNTAX scores were calculated for all patients. Patients were classified into three groups based on their SYNTAX score values using cut-offs from the US/European guidelines [low ≤ 22 (n = 34), intermediate 23–32 (n = 10), and high score >33 (n = 4)] (13, 14). Moreover, information on occlusion of proximal RCA was also collected in order to perform subanalysis correlating proximal RCA occlusion and gene expression of RAA. Major adverse cardiovascular and cerebrovascular events (MACCE) relied on a four-point MACCE score: death due to a cardiovascular reason, non-fatal stroke, non-fatal myocardial infarction, and hospitalization due to heart failure (HF). MACCE and mortality rates were recorded from a 5-year postoperative follow-up.
Surgery benefit was assessed by calculating the preoperative and post-operative ejection fraction (EF). Preoperative EF was evaluated within 1 month before surgery, and post-operative EF was determined during a 3-month control visit. An EF change was calculated (postoperative EF – preoperative EF), and patients were divided into two groups according to the result: (a) good benefit group (postoperative EF >10% better than preoperative EF) and (b) poor benefit group (postoperative EF reduced >10% compared to preoperative EF).
Validation
The six formalin-fixed AA samples for validation were paraffin-embedded at the Tissue Preparation and Histochemistry Unit (Faculty of Medicine, University of Helsinki, Finland). Immunohistochemistry for FUT10 and CSRNP3 was performed using standardized protocols at BioSiteHisto Ltd (Tampere, Finland) and the primary antibodies validated in the Human Protein Atlas project (www.proteinatlas.org, rabbit anti-human CSRNP3 HPA017905 used here at dilution 1:75, and rabbit anti-human FUT10 HPA053970 used here at dilution 1:50; both from Sigma Aldrich, Merck KGaA, Darmstadt, Germany). Staining was cross-validated against in-house human tissue control sections. The immunostained RAA tissue sections were scanned with the 3d-HISTECH Pannoramic MIDI II imaging system using a x20 objective at 0.23 × 0.23 μm/pixel resolution (3DHISTECH Ltd., Budapest, Hungary). For analysis, serial non-overlapping images at × 20 magnification covering each section were captured with the Pannoramic Viewer software (3DHISTEC Ltd.). The analysis of the captured images was carried out using the FiJI ImageJ software (15). The image analysis macros are available upon request from the corresponding author. Briefly, for each image, after background subtraction, the color deconvolution algorithm to hematoxylin (H) and diaminobenzidine (DAB) channels was utilized. The DAB channel image was thresholded to the stain using the automated default method based on the IsoData algorithm, and the stain intensity was measured. For nuclear counting, hematoxylin-positive nuclei (representing the total amount of nuclei in the image) were counted from the H channel using the particle-counting algorithm and compared with the thresholded positively immunostained nuclei (stained nuclei) counted from the DAB channel. The results of the densitometric image analysis of the serial images for each sample were first averaged, and these single values were then used for the further combined analysis of results.
Statistical Analyses
Differentially expressed genes between phenotypic ischemic and non-ischemic patient groups were identified by performing permutation tests using gene TPMs (Transcripts per Million). Results from the permutation test were corrected using the Benjamin-Hochberg FDR correction (FDR <0.05). Correlation analyses relied on Pearson's correlation comparing blood test parameters and gene TPMs, for which we applied p < 0.05. Genes associated with the SYNTAX score groups were identified through a one-way ANOVA (p < 0.05) using the TPM values for genes that were differentially expressed compared to GTEx. For the clinical data, statistical differences in continuous variables were calculated using the Kruskal-Wallis test (three groups) and the Mann-Whitney U-test (two groups). Statistical differences in categorical variables were calculated using Fisher's exact test. We considered p < 0.01 to denote statistical significance, and all p-values were two-tailed. Clinical data were analyzed using SPSS (IBM's SPSS Statistics for Windows, version 25.0, IBM Corp., Armonk, NY).
Results
Patients in the GTEx and non-IHD groups were younger (mean age 56.26 years ± 10.74 and 59.75 ± 12.47, respectively) than IHD patients (mean age 68.20 ± 8.28) (Figure 1A). While the mean SYNTAX score was 0 in the non-IHD group, in the IHD group it reached 18.29 ± 8.95. In addition, diabetes occurred more frequently in the IHD group. Aortic cross-clamp times and cardiopulmonary bypass (CPB) times were relatively longer in the non-IHD group.
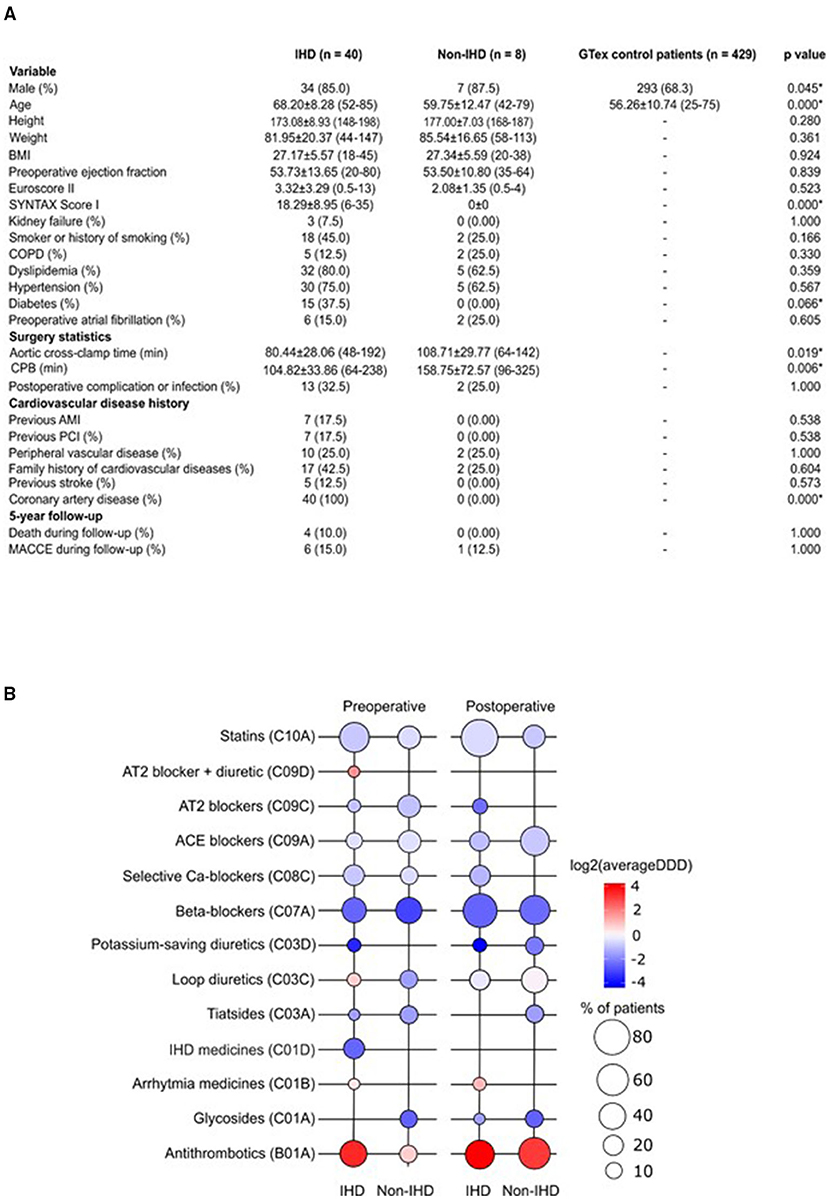
Figure 1. (A) Demographic characteristics of the study population. AMI, acute myocardial infarction; BMI, body mass index; COPD, chronic obstructive pulmonary disease; CPB, cardiopulmonary bypass; MACCE, major adverse cardiovascular and cerebrovascular event; PCI, percutaneous coronary intervention. (B) Pre- and post-operative medication use in the study groups. Color key representing the total use of medication (as log2 average DDD); bubble plot representing the percentage of patients on medication indicated in study groups. DDD, defined daily dose.
Preoperative medication in the IHD group reflected ischemic disease, particularly the use of organic nitrates; no organic nitrate use was recorded among non-IHD patients (Figure 1B). Post-operatively, the dyspnea- and chest pain symptom-alleviating effects of CABG surgery were reflected as an extinct use of organic nitrates in IHD patients. Drug treatments were overall more uniform following surgery. Postoperatively, statin treatment was more common among IHD patients (p = 0.006). We detected differences in postoperative complications or infections when comparing groups. In total, three IHD patients, but no non-IHD patients, were treated with antiarrhythmic medication during the preoperative and postoperative periods. Overall, ACE inhibitors and antithrombotics were used more during the post-operative period.
DEGs Between IHD and Non-IHD Compared to GTEx Database
A comparison of the gene expression profiles of the IHD group and GTEx controls yielded 672 differentially expressed genes (DEGs), 360 of which were upregulated and 312 downregulated (Figure 2A). Sirtuin signaling, epithelial adherens junction signaling, and serine peptidase inhibitor Kazal type 1 (SPINK1) were significantly upregulated in the IHD group. In contrast, agrin interactions and oxidative phosphorylation were significantly downregulated (Figure 2D). In the non-IHD vs. GTEx comparison, we identified 342 upregulated and 416 downregulated DEGs (Figure 2B). DEGs correlated with inflammatory and immunological processes (Figure 2D). The natriuretic peptide B (NPPB) coding gene was differentially expressed in both the IHD (logFC −2.65, p < 0.001) and non-IHD (logFC −4.08, p = 0.001) groups vs. the GTex group.
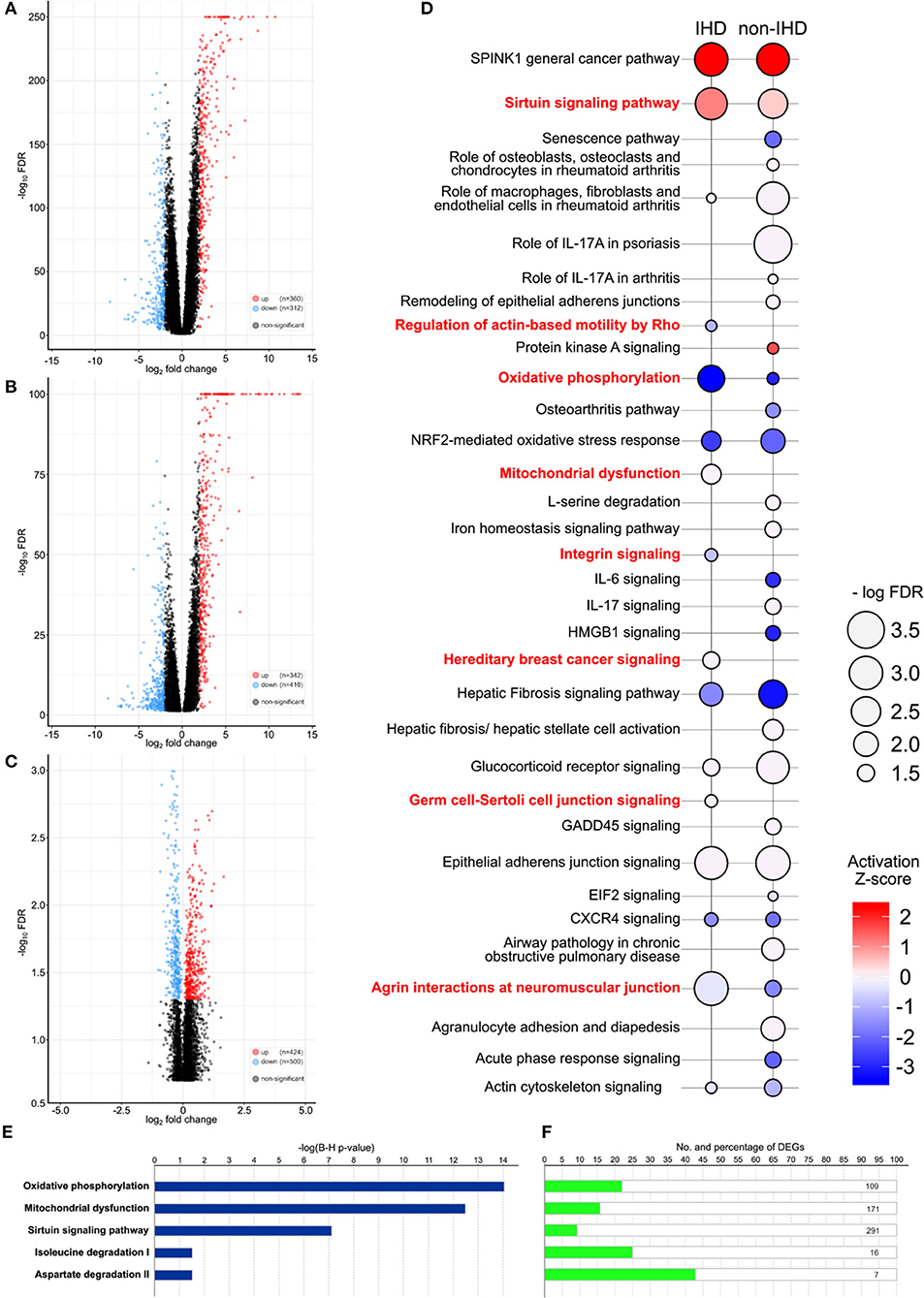
Figure 2. (A–C) Volcano plots providing an overview and the number of differentially expressed genes (DEGs). IHD vs. GTEx patients (A), non-IHD vs. GTEx patients (B), and IHD vs. non-IHD patients (C). (D) DEG-related pathways between IHD and non-IHD vs. GTEx comparison. Major differences are highlighted in red, with the color key showing the activation Z-score. The size of a balloon represents the –log(FDR) value. (E) P-values for the top five enriched pathways in IHD vs. non-IHD group comparisons. (F) Number of DEGs in the top five enriched pathways IHD vs. non-IHD group comparisons.
Differences Between IHD and Non-IHD Groups
Comparison of IHD and non-IHD samples resulted in 424 significantly upregulated and 500 significantly downregulated genes (Figure 2C). DEGs were enriched in oxidative phosphorylation, mitochondrial dysfunction, sirtuin signaling, isoleucine degradation, and aspartate degradation (Figures 2E,F). The two latter pathways strengthen previous associations identified for branched-chain amino acids and aspartate as metabolic contributors to IHD (16, 17). As such, our results directly situate myocardial changes in these amino acid pathways as the culprit in IHD.
The list of differentially expressed miRNAs, significant downstream pathways, and functionally annotated targets of these miRNAs from comparison of IHD and non-IHD is shown in Figures 3A,B. We found a total of 13 differently expressed miRNAs between groups (Figure 3A). The target genes of these miRNAs are significantly enriched in cardiac β-adrenergic signaling, role of NFAT in cardiac hypertrophy, androgen signaling, and complement system pathways. A three-layer subnetwork of interactions between miRNA, their differentially expressed target genes, and their functions appears in Figure 3C. Most of the miRNA targets in this subnetwork are downregulated. These genes play a role in muscle cell death, metabolizing pyruvic acid, fibroblast cell line proliferation, cell invasion, synthesizing ATP, and degranulating neutrophils.
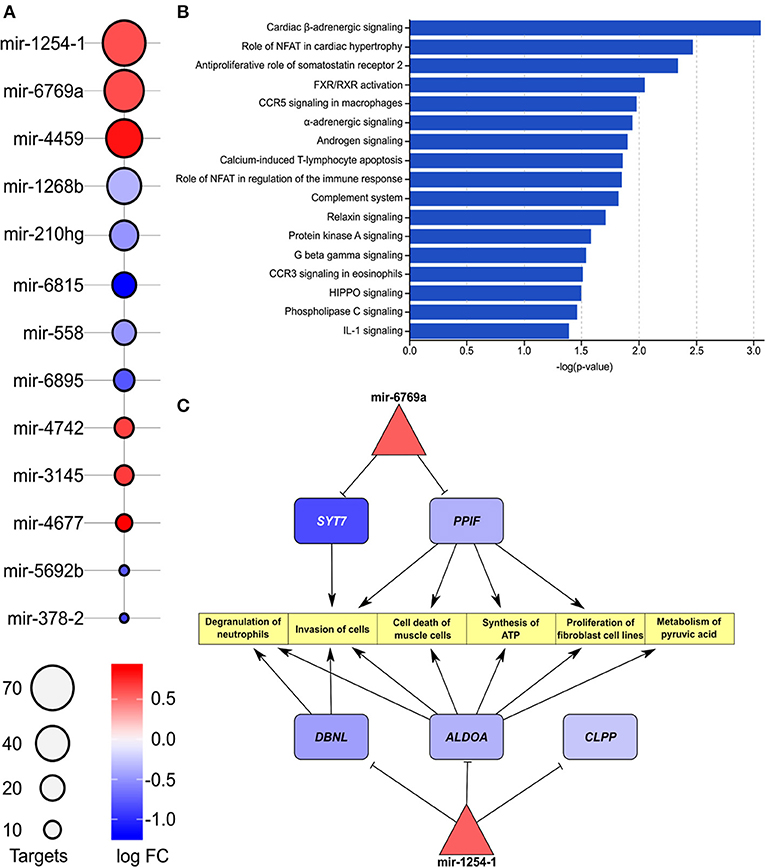
Figure 3. (A) List of enriched miRNAs in IHD vs. non-IHD patients. Color key, log FC; bubble plot, number of targets. (B) List of pathways related to enriched miRNAs. The bars express –log(p-value). (C) Differentially expressed downstream target genes and pathways related to hsa-mir-1254-1 and hsa-mir-6769a.
Clinical Associations to Preoperative Condition
Ejection Fraction
We further divided IHD patients into two groups based on a high (≥55%) or low (<55%) preoperative EF value and clustered the genes accordingly (Figure 4A). Protocadherin gamma family members (PCDHGs) exhibited higher expression in the low preoperative EF group (EF <55%). The correlation between PCDHGs and preoperative laboratory tests appear in Figure 4B. PCDHGA1 and PCDHGA12 positively correlated with preoperative NT-proBNP levels (Pearson R = 0.6, p = 0.04), while all PCDHGs negatively correlated with preoperative EF values (Figure 4B). In addition, Figure 4C displays selected PCDHGs and their expressions based on the preoperative EF groups. Each selected PCDHG is highly expressed in patients in the low EF group compared to the high EF group. These results further strengthen the association of the PCDHG gene cluster with cardiac dysfunction (18).
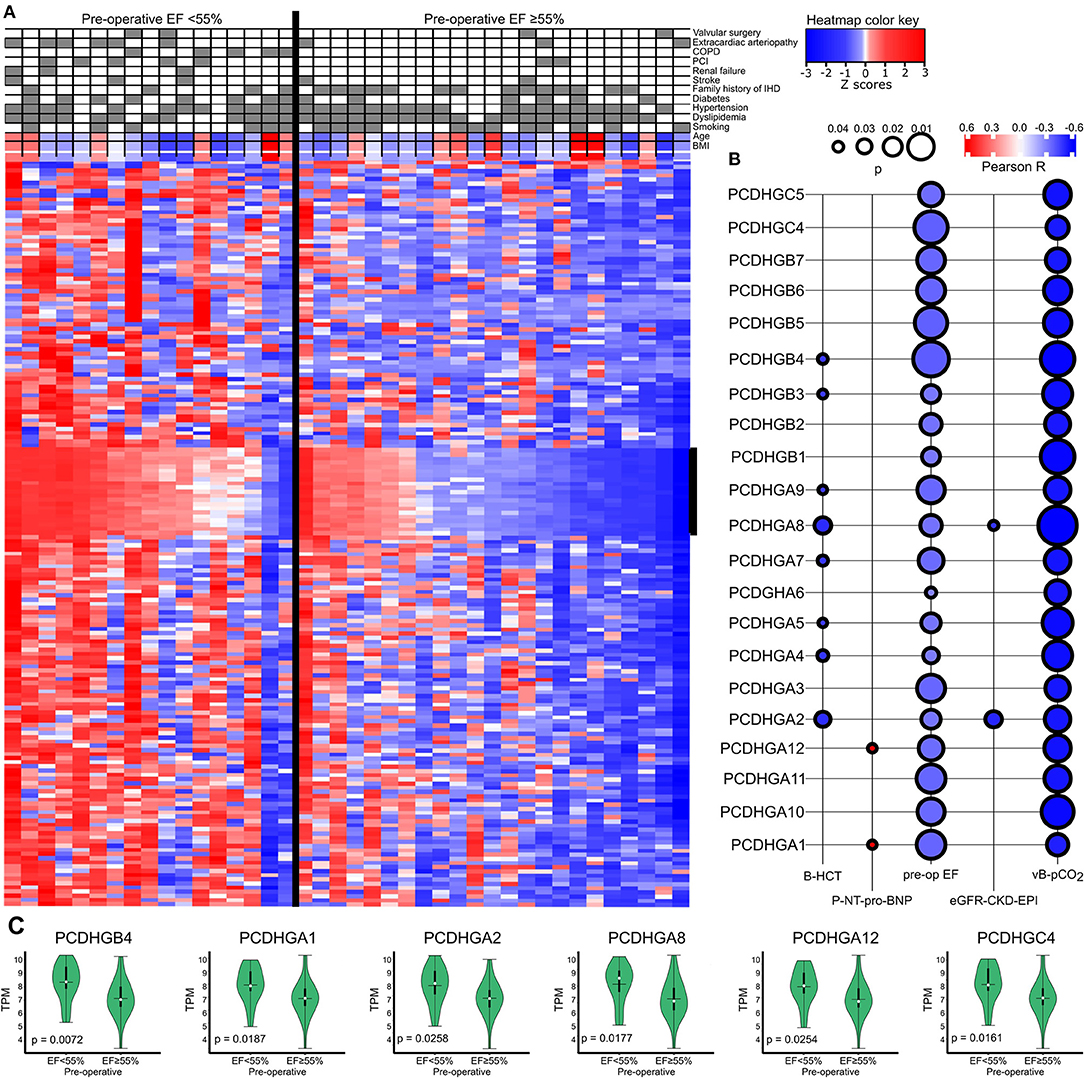
Figure 4. (A) Heatmap showing a general overview of DEGs comparing the categorized EF groups in IHD patients. The heatmap color key expresses the Z-scores. (B) Correlations between PCDHGs and preoperative EF and preoperative laboratory values. The size of the bubble indicates the p-value and the color key indicates the Pearson R. (C) Correlations between preoperative EF and PCDHGs. EF, ejection fraction; PCDHG, protocadherin.
SYNTAX Score and Laboratory Measurements
We categorized the patients into three groups according to low, intermediate, or high SYNTAX scores reflecting the level of coronary artery obstruction complexity. All IHD patients except one had obstructions also in the right coronary artery (RCA). Total of 30 patients had occlusion in the proximal RCA, 7 patients in the mid RCA and 2 in the distal RCA. We identified 11 DEGs across the groups (ANOVA test, Figure 5A). Among them, CSRNP3 (Cysteine and Serine Rich Nuclear Protein 3) and FUT10 (Fucosyltransferase 10) genes were common in DEGs in the IHD vs. non-IHD groups (ANOVA p = 0.045 and p = 0.043; FDR 0.036 and 0.030, respectively). Although the differential expression of COX5A (Cytochrome C Oxidase Subunit 5A) and ACSBG1 (Acyl-CoA Synthetase Bubblegum Family Member 1) suggested IHD selectivity, with their expressions significantly correlating with the SYNTAX scores (ANOVA p = 0.012 and p = 0.030; FDR 0.060 and 0.085, respectively), this difference did not emerge in the DEG comparison between the IHD compared with non-IHD groups.
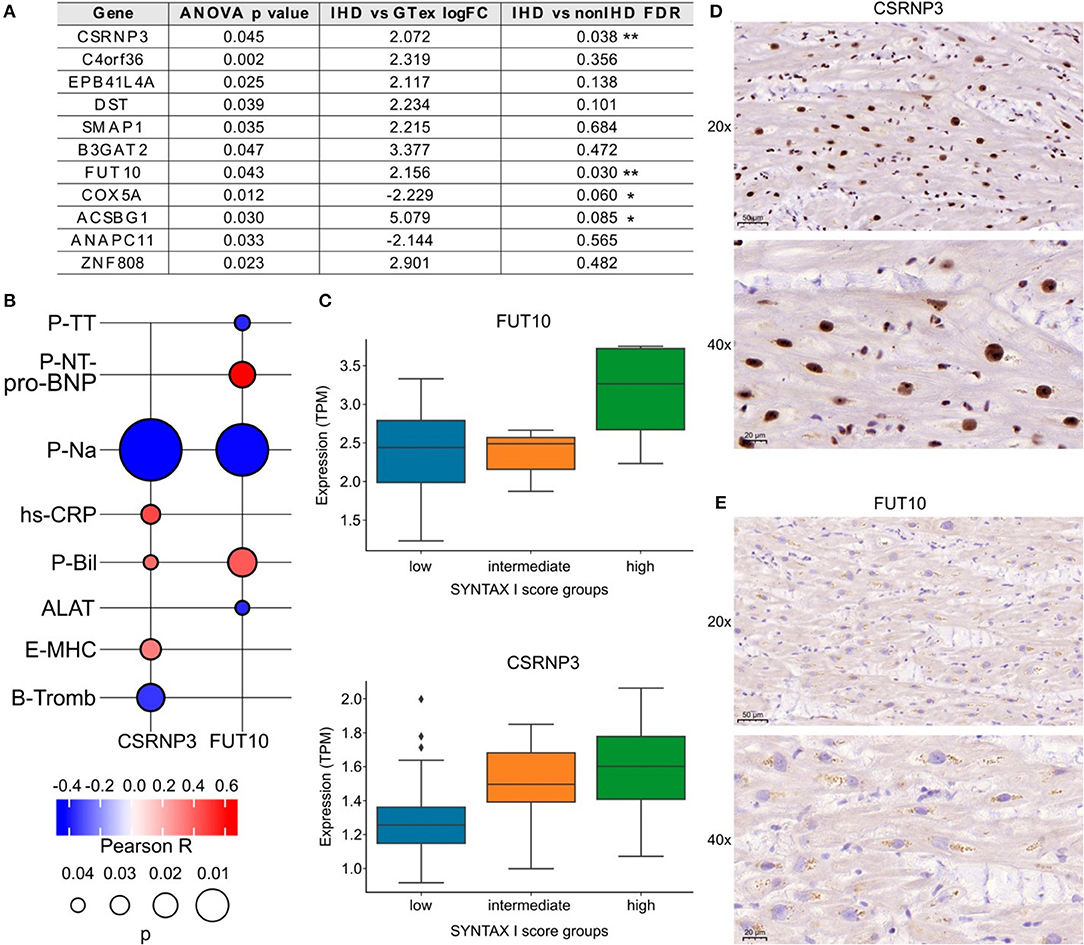
Figure 5. (A) List of genes associated with SYNTAX score I. (B) FUT10 and CSRNP3 correlations with preoperative laboratory tests. The color key illustrates the Pearson R and the size of the bubble indicates the p-value. (C) FUT10 and CSRNP3 expression in the SYNTAX score groups. (D,E) Representative CSRNP3 and FUT10 stainings from the validation group.
The associations between FUT10 and CSRNP3 and the preoperative laboratory values appear in Figure 5B. FUT10 positively correlated with preoperative NT-proBNP levels (Pearson R = 0.6; p = 0.03), whereas CSRNP3 positively correlated with the blood CRP levels (Pearson R = 0.4; p = 0.03). The expressions of CSRNP3 and FUT10 were higher in the high SYNTAX score category (Figure 5C), suggesting that lower CSRNP3 and FUT10 expression levels reflect milder or less complex coronary artery disease. Figures 5D,E provide representative immunohistochemistry images of the validation arm RAA samples with primary antibodies against the FUT10 and CSRNP3 proteins. The results from the immunohistochemistry analysis and box plots showing the percentage of area staining for CSRNP3 and FUT10 compared to the total sample area appear in Supplementary Figure 1. We identified no statistically significant differences although FUT10 protein expression presented with an increased tendency in the high SYNTAX score group. Further analysis of the FUT10 transcripts revealed the highest expression for the FUT10-201 transcript (median TPM >2.5) in our study population (Supplementary Figure 2) suggesting differential regulation of alternate transcription. Results from RCA-occlusion subanalysis are presented in Supplementary Figure 3.
Molecular Associations With Surgery Benefit
IHD patients were divided into groups based on a positive or negative benefit from surgery using the difference between pre- and post-operative EF values. Positive or negative benefit was assigned if the magnitude of the EF difference was 10% or more. Comparing the positive- and negative-benefit groups revealed a total of 271 DEGs. The clinical parameters among patients appear in the upper panel of Figure 6. Despite the clinical heterogeneity across patients, we identified 271 DEGs, among which 166 genes were downregulated and 105 genes were upregulated in the negative-benefit group compared to the positive-benefit group. The pathways associated with a surgical benefit (Figure 6) emerged from a functional enrichment analysis. The degradation of connective tissue (FDR = 0.031), cell migration (FDR = 0.034), and cell movement (FDR = 0.034) were upregulated in the negative-benefit group. The pathways related to an enlargement of the heart (FDR = 0.013), cardiomyopathy in the heart ventricle (FDR = 0.019), and the organization of mitochondria (FDR = 0.019) were upregulated in the positive-benefit group. Cardiac status-related demographics of benefit groups are presented in Supplementary Figure 4. Preoperative EF and first postoperative day CK-MBm were higher in the negative benefit group (Supplementary Figure 4A). No clinical observations of perioperative myocardial infarctions were documented. The magnitude of the EF change was significantly higher in the positive benefit group (Supplementary Figure 4). Linearly correlated genes with an EF change appear in Figure 7. The top three highest logFC genes comparing surgery benefit classes were mir-181b (Pearson R = 0.362, p = 0.014), NAV2-AS4 (Pearson R = −0.327, p = 0.028), and SHD (Pearson R = −0.297, p = 0.047).
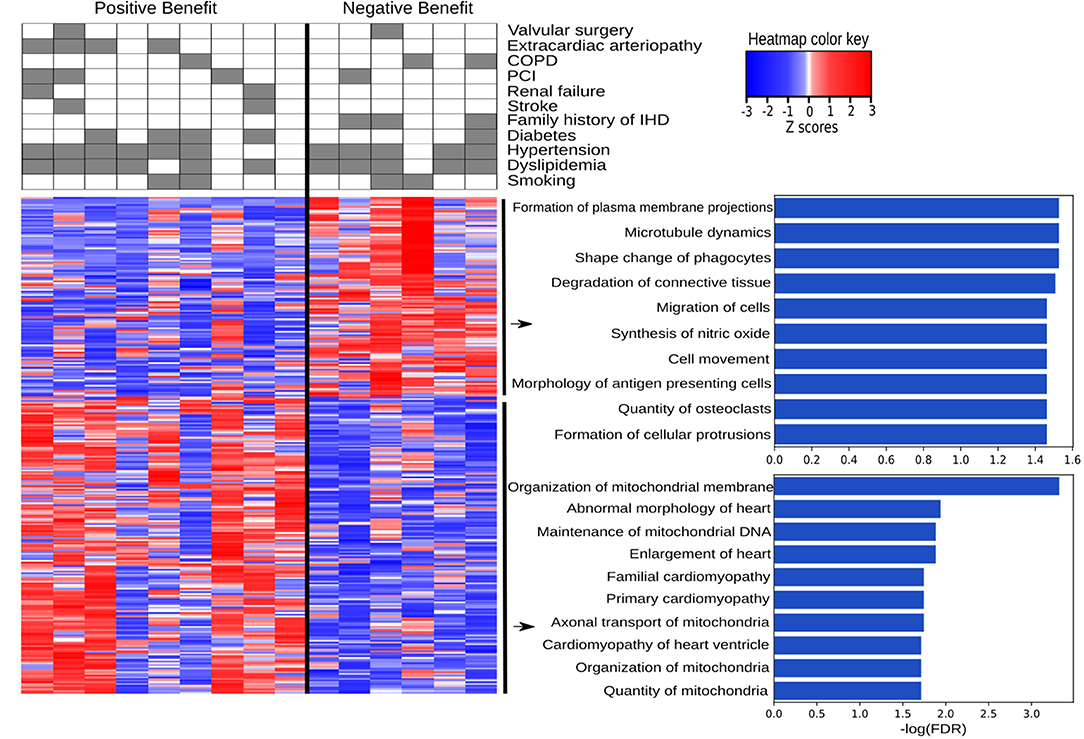
Figure 6. Pathways associated with a positive and negative benefit from surgery according to EF change. The heatmap provides a visual overview of the differences between two groups in terms of DEGs and clinically associated diseases, with color key representing the Z-score, and the top ten up and down regulated pathways are listed with their log(FDR) values.
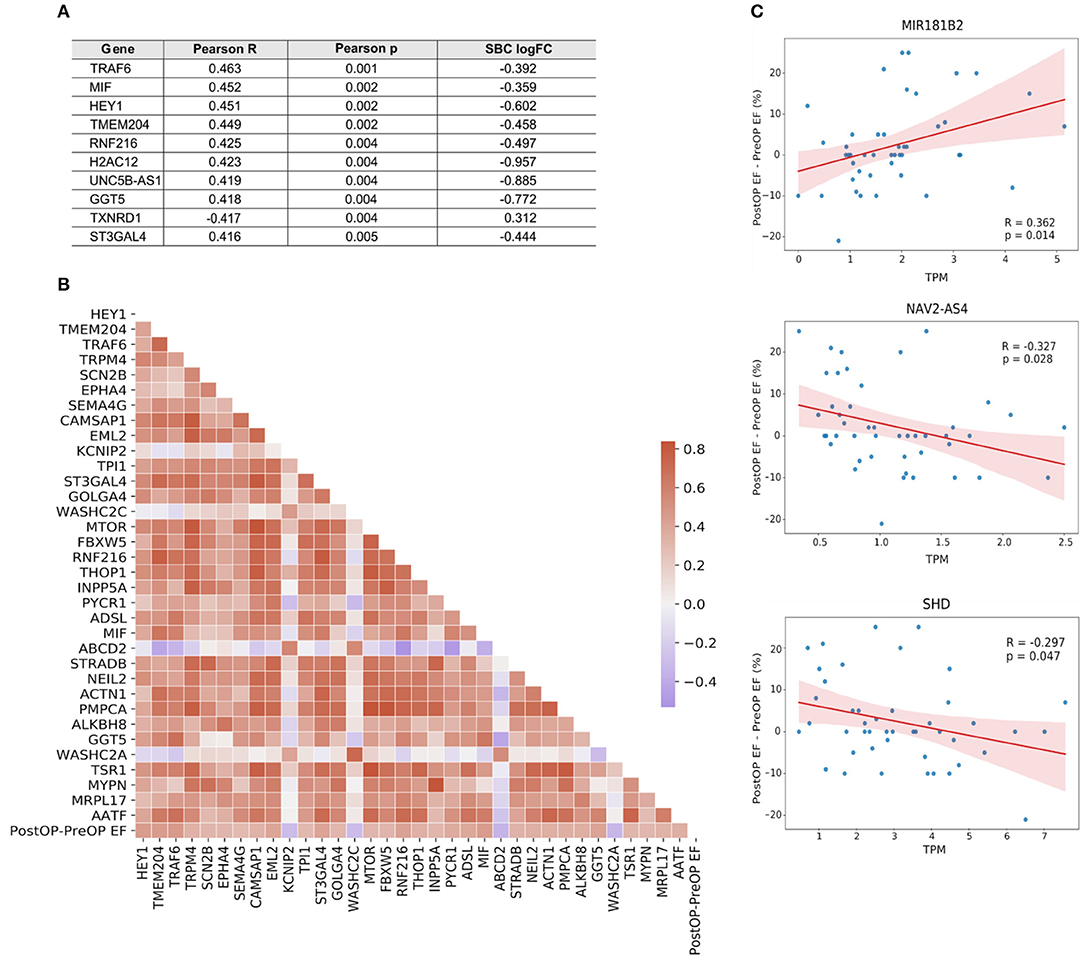
Figure 7. (A) Top 10 genes linearly correlated with the change in EF following surgery. (B) Correlation heatmap of the genes mapped to a GO biological process. Color key, correlation coefficient. (C) Correlation plots of the top three genes with the highest |logFC| between surgery benefit classes.
Discussion
In this study, we present analysis of an IHD-selective RAA transcriptome and its relations to disease severity and outcome from cardiac surgery. In addition, identification of known IHD-associated pathways including changes in energy metabolism, cell structure, and surface proteins in the heart under pathological stress (16–21) further validated the applicability of the RAA as a surrogate source of information for enhancing our tissue-level understanding of human IHD.
We utilized the SYNTAX score, calculated based on coronary angiography, as a parameter for the complexity of coronary artery obstructions (22). Using ANOVA test, we detected significant results in gene expression between the SYNTAX scores and two IHD-associated DEGs. FUT10, or fucosyltransferase, catalyzes the transfer of a fucose residue (23), promotes adipocyte proliferation, and inhibits cell differentiation (24). Furthermore, it is a regulator of cell proliferation, particularly in stem cell self-renewal and in the maintenance of stem cell populations (25). In addition, FUTs are hallmarks of M1 inflammatory macrophages, and their expression, including that of FUT10, increases in inflammatory lesions (26). Recently, the post-translational role of FUTs in the glycosylation of immunoglobulins has garnered increasing interest. FUT10 may serve as a possible target for therapeutic intervention (27). Interestingly, one protein-coding transcript (FUT10-201) incorporates a signal peptide, to potentially produce a secreted protein (28). FUT10-201 transcript emerged as the most highly expressed in our sequencing analysis among sampled patients. This transcript and its coded (secreted) protein represent interesting candidates as tissue-level and/or circulating biomarkers for IHD. The expression of FUT10 resulted significant with SYNTAX scores and correlated with preoperative NT-proBNP levels strengthening the correlation between increasing fucosylation and decreasing cardiac function in IHD.
CSRNP3 gene expression resulted significant with the SYNTAX scores and correlated with preoperative CRP levels, indicating its potential to serve as an IHD-specific marker. CSRNP3 is expressed in the nucleus, carries transcription factor activity (29), and links to apoptosis (30). Recently, CSRNP3 was identified as one of eight overlapping genes between CD90pos MSCs (31) and the atherosclerosis associated gene expression dataset of aorta and fat tissue, STARNET (32). Our study further strengthens the association between CSRNP3 and IHD/CAD, providing additional evidence of its cardiac tissue IHD-specific expression. Subanalysis correlating gene expression with proximal RCA occlusion strengthened the discovery of HEY1—a transcriptional suppressor and an atrial NOTCH, TGFbeta, and HIF1 pathway target gene—as an indicator of cardiovascular functional reserve in myocardial ischemia.
Upregulated mir-1254, mir-6769, and mir-4459 and their downstream target genes in IHD RAA showcase an interregulating network related to inflammation and immune regulation, cardiac hypertrophy, and adrenergic signaling.
The hsa-mir-1254 and its target gene DBNL regulate cell proliferation and immune responses (33–35), and increased expression of DBNL has been reported in experimental dilated cardiomyopathy (36). We found an IHD-associated downregulation of two other hsa-mir-1254 target genes, ALDOA (aldolase) and CLPP (caseinolytic mitochondrial matrix peptidase proteolytic subunit). In hypertrophic cardiomyopathy, reduced tissue expression of aldolase (37) and increased plasma concentrations of secreted aldolase in cardiomyocyte-derived exosomes have been reported (38, 39). In our data, reduced ALDOA expression in RAA was associated with IHD, suggesting a similar tissue-level regulation to that observed in hypertrophic myocardium, possibly indicating a common metabolic dysregulation in these diseases. Reduced expression of CLPP, as found here to be IHD-associated, has also been reported in knockout mice to decrease formation of cardiac mitochondrial respiratory supercomplexes, T-cell activation, and increased production of mediators of inflammation and immunity contributing to inflammatory tissue injury (40, 41). Furthermore, reduced cardiac CLPP expression may contribute to mitochondrial dysfunction and tissue injury in IHD, but its actions in the heart seem to depend on the disease background and require further elucidation (42). The RAA IHD-associated miRNA hsa-mir-6769a regulates the expression of synaptotagmin-7 (SYN7). Syn7 knock-out mice present with increased cardiac inflammation and deposition of collagen (43) suggesting that increased hsa-mir-6769a may drive myocardial fibrosis, thus contributing to fibrosis in RAA. Another IHD-associated RAA miRNA found in our study, hsa-mir-4459, has been reported to suppress inflammatory reactivity and autophagy of vascular endothelial cells through inhibition of its proinflammatory target gene LARP-1 (44) thereby possibly also contributing to regulation of the inflammatory tissue response in RAA under IHD.
We found IHD-associated reduced expression of the insulin-like growth factor-1 receptor (IGR-1R) signaling effector (45) hsa-mir-6815 in the RAA samples. Interestingly, it has been shown that macrophages deficient of IGR-1R accelerate atherosclerosis and increase atherosclerotic plaque vulnerability (46) and that the hsa-mir-6815 response is activated under hypoxia and coagulation (47). Taken together with our results, these findings suggest that these RAA miRNAs can contribute to the tissue level pathological process observed in IHD.
Our results suggest that IHD is associated with an increased expression of transcriptional repressors, implying a complex modulation of metabolic inflammation or macrophage polarization through transcription factor activity and putative aggravation through suppressing inflammatory repressors (48). For example, KLF10 and KLF11 are tightly linked to vascular inflammation, but also carry an anti-inflammatory role in experimental disease models and in in vitro studies (49). Our results associated KLF10 and KLF11 with IHD, thereby indicating a further complexity to the transcriptional regulator networks in the multimodal pathology of IHD. This agrees with our findings of IHD-associated increases in the expression of the inflammation-associated transcriptional activator RUNX1 (50) together with the transcription regulator MYEF2 shown to modify RUNX1 function and target gene selectivity (51). Thus, it seems feasible to assume a more elaborate and environmentally sensitive mechanism for these factors in complex metabolic diseases such as IHD (52).
We then analyzed the RAA DEGs that were associated with the patients' functional cardiac benefit from surgery. Despite the complexity of categorical EF use, we were able to discover DEGs that were related to absolute EF change and correlated with surgery benefit without EF categorization. One such DEG, SHD, encodes for an SH2 homology domain-containing protein SHD, which interacts with key signaling phosphoprotein receptors such as c-MET (53). Also the cis-natural antisense transcript NAV2-AS4 significantly correlated with a benefit from surgery. NAV2 expresses in the heart (54), but its role has remained unspecified. It has, however, been shown to regulate cell adhesion and neurite outgrowth (55). Hsa-mir-181 expression levels significantly negatively correlated with cardiac surgery benefit. Changes in mir-181 expression have been associated with the early stages of heart failure (56), regulating thrombin-induced endothelial cell activation and dysfunction, arterial thrombosis pathology, and promoting atherosclerosis (57). Moreover, diminished mir-181b levels have correlated with the ability to remodel the extracellular matrix affecting vascular stiffness via TGF-β signaling (58), while circulating mir-181b levels have been associated with diabetic cardiomyopathy (59). Due to mir-181's ability to reflect cardiac status, its expression represents a potential novel marker for predicting cardiac surgery benefit. As associated with a functional benefit from surgery, the expression of these DEGs can serve as an indicator for the patient's cardiac functional recuperation reserve.
This study provides a rationale for the utilization of RAA tissue RNA profiling in the search of potential IHD biomarkers. We meticulously collected available clinical, imaging, and laboratory data from electronic healthcare records as well as documented patient drug treatments at a high resolution. All analyses progressed according to clinical guidelines for patient treatment and, thus, any study-guided analysis bias can be eliminated. The classification of medications according to their ATC class and the standardization of doses using DDD conversions relying on the InnoLIMS Medical software platform enabled us to compare drug treatments at various time points and intervals. We also performed an initial immunohistochemical validation of results for early preliminary confirmation of our results.
Our study also has several limitations. The total number of patients in the study and the relatively low number of non-IHD patients limit our analytical power. Another limitation lies in the retrospective collection of clinical data from healthcare databases, possibly leading to incomplete coverage and interobserver variability in the clinical measurements. Lastly, immunohistochemical validations were performed on a limited number of patient samples, with varying levels of IHD according to the SYNTAX scores. Non-IHD patient samples or samples from donors without cardiac disease were not used. Thus, validation on a larger cohort is necessary. Our recently initiated IHD-EPITRAN project (ClinicalTrials.gov identifier NCT04533282) will address this limitation (60).
Based on their differential expression in the RAA tissue of patients with or without IHD, we present here a specific set of IHD-associated RNAs expressing significantly differently when compared to coronary artery disease complexity, and correlating with CRP, NT-proBNP, and surgery outcomes. Specifically, FUT10 and CSRNP3 represent lucrative candidates as IHD biomarkers.
Data Availability Statement
The RNA sequencing data is available from the Gene Expression Omnibus database repository under the identification GSE173594, https://www.ncbi.nlm.nih.gov/geo/query/acc.cgi?acc=GSE173594.
Ethics Statement
The studies involving human participants were reviewed and approved by the Operative Ethics Committee of the Hospital District of Helsinki and Uusimaa. The patients/participants provided their written informed consent to participate in this study.
Author Contributions
MKu, AV, AH, EK, and JL: conceptualization. MKa, AH, EK, AV, ML, AN, and JL: methodology. SM, AN, KT, ML, TN, JL, TO, MKa, AE, and NT: investigation. SM, EK, NT, and AE: visualization and writing—original draft. EK, AV, AH, MKu, and NT: funding acquisition and project administration. EK, AV, AH, and NT: supervision. SM, AE, EK, NT, ML, TN, KT, TO, MKa, AV, JL, MKu, AH, and NT: writing—review and editing. All authors have reviewed and approved the manuscript.
Funding
This study was funded by the Finnish Medical Foundation (SM), the Aarne Koskelo Foundation (SM), the Finnish Foundation for Cardiovascular Research (ML), Finnish government subsidies for medical research block grants (EVO, AH TYH2014207 and MK TYH2015311), the Finnish Funding Agency for Technology and Innovation (EK 3137/31/2013), UNESCO-L'Oréal National Fellowship for Women in Science (NT), UNESCO-L'Oréal International Rising Talent Fellowship (NT), and TUBA-GEBIP (NT).
Conflict of Interest
The authors declare that the research was conducted in the absence of any commercial or financial relationships that could be construed as a potential conflict of interest.
Publisher's Note
All claims expressed in this article are solely those of the authors and do not necessarily represent those of their affiliated organizations, or those of the publisher, the editors and the reviewers. Any product that may be evaluated in this article, or claim that may be made by its manufacturer, is not guaranteed or endorsed by the publisher.
Acknowledgments
The authors are extremely grateful for the expert help and assistance provided by research nurse Liisa Blubaum-Innanen, research nurse Kati Helleharju, research technician Lahja Eurajoki, and research technician Ulla Kiiski. We gratefully acknowledge the Tissue Preparation and Histochemistry Unit (Faculty of Medicine, University of Helsinki, Finland) for help with tissue processing, and BioSiteHisto Ltd (Tampere, Finland) and MSc Johanna Lappeteläinen for performing the immunohistochemistry experiments.
Supplementary Material
The Supplementary Material for this article can be found online at: https://www.frontiersin.org/articles/10.3389/fcvm.2021.728198/full#supplementary-material
References
1. Gupta R, Yusuf S. Challenges in management and prevention of ischemic heart disease in low socioeconomic status people in LLMICs. BMC Med. (2019) 17:209. doi: 10.1186/s12916-019-1454-y
2. Dhingra R, Vasan RS. Biomarkers in cardiovascular disease: statistical assessment and section on key novel heart failure biomarkers. Trends Cardiovasc Med. (2017) 27:123–33. doi: 10.1016/j.tcm.2016.07.005
3. Libby P, Buring JE, Badimon L, Hansson GK, Deanfield J, Bittencourt MS, et al. Atherosclerosis. Nat Rev Dis Prim. (2019) 5:56. doi: 10.1038/s41572-019-0106-z
4. de Roos A, Nikolaou K. CT and MRI in suspected ischemic heart disease. In: Hodler J, Kubik-Huch RA, von Schulthess GK, editors. Diseases of the Chest, Breast, Heart and Vessels 2019-2022: Diagnostic and Interventional Imaging. Cham: IDKD Springer Series (2019). p. 179-87. doi: 10.1007/978-3-030-11149-6_15
5. Huang Y, Gulshan K, Nguyen T, Wu Y. Biomarkers of cardiovascular disease. Dis Mark. (2017) 2017:8208609. doi: 10.1155/2017/8208609
6. Richards AM. Future biomarkers in cardiology: my favourites. Eur Heart J Suppl. (2018) 20:G37–44. doi: 10.1093/eurheartj/suy023
7. Bolger AM, Lohse M, Usadel B. Trimmomatic: a flexible trimmer for Illumina sequence data. Bioinformatics. (2014) 30:2114–20. doi: 10.1093/bioinformatics/btu170
8. Flicek P, Amode MR, Barrell D, Beal K, Billis K, Brent S, et al. Ensembl 2014. Nucleic Acids Res. (2014) 42:D749–55. doi: 10.1093/nar/gkt1196
9. Dobin A, Davis CA, Schlesinger F, Drenkow J, Zaleski C, Jha S, et al. STAR: ultrafast universal RNA-seq aligner. Bioinformatics. (2013) 29:15–21. doi: 10.1093/bioinformatics/bts635
10. Liao Y, Smyth GK, Shi W. featureCounts: an efficient general purpose program for assigning sequence reads to genomic features. Bioinformatics. (2014) 30:923–30. doi: 10.1201/b16589
11. Robinson MD, McCarthy DJ, Smyth GK. edgeR: a Bioconductor package for differential expression analysis of digital gene expression data. Bioinformatics. (2010) 26:139–40. doi: 10.1093/bioinformatics/btp616
12. Agarwal V, Bell GW, Nam JW, Bartel DP. Predicting effective microRNA target sites in mammalian mRNAs. Elife. (2015) 4:e05005. doi: 10.7554/eLife.05005.028
13. Windecker S, Kolh P, Alfonso F, Collet JP, Cremer J, Falk V, et al. 2014 ESC/EACTS guidelines on myocardial revascularization: The Task Force on Myocardial Revascularization of the European Society of Cardiology (ESC) and the European Association for Cardio-Thoracic Surgery (EACTS) developed with the special contribution of the European Association of Percutaneous Cardiovascular Interventions (EAPCI). Eur Heart J. (2014) 35:2541–619. doi: 10.1093/eurheartj/ehu278
14. Amsterdam EA, Wenger NK, Brindis RG, Casey DE Jr., Ganiats TG, Holmes DR Jr., et al. 2014 AHA/ACC guideline for the management of patients with non-ST-elevation acute coronary syndromes: a report of the American College of Cardiology/American Heart Association Task Force on Practice Guidelines. J Am Coll Cardiol. (2014) 64:e139–228. doi: 10.1016/j.jacc.2014.09.016
15. Schindelin J, Arganda-Carreras I, Frise E, Kaynig V, Longair M, Pietzsch T, et al. Fiji: an open-source platform for biological-image analysis. Nat Methods. (2012) 9:676–82. doi: 10.1038/nmeth.2019
16. Chistiakov DA, Shkurat TP, Melnichenko AA, Grechko AV, Orekhov AN. The role of mitochondrial dysfunction in cardiovascular disease: a brief review. Ann Med. (2018) 50:121–7. doi: 10.1080/07853890.2017.1417631
17. Zhao JV, Kwok MK, Schooling CM. Effect of glutamate and aspartate on ischaemic heart disease, blood pressure, and diabetes: a Mendelian randomisation study. Lancet. (2018) 392:S23. doi: 10.1016/S0140-6736(18)32652-7
18. Ortega A, Gil-Cayuela C, Tarazon E, Garcia-Manzanares M, Montero JA, Cinca J, et al. New cell adhesion molecules in human ischemic cardiomyopathy. PCDHGA3 implications in decreased stroke volume and ventricular dysfunction. PLoS ONE. (2016) 11:e0160168. doi: 10.1371/journal.pone.0160168
19. Simon T, Taleb S, Danchin N, Laurans L, Rousseau B, Cattan S, et al. Circulating levels of interleukin-17 and cardiovascular outcomes in patients with acute myocardial infarction. Eur Heart J. (2012) 34:570–7. doi: 10.1093/eurheartj/ehs263
20. Kim N, Lee S, Lee J-R, Kwak Y-L, Jun J-H, Shim J-K. Prognostic role of serum high mobility group box 1 concentration in cardiac surgery. Sci Rep. (2020) 10:6293. doi: 10.1038/s41598-020-63051-2
21. Hardt SE, Sadoshima J. Negative regulators of cardiac hypertrophy. Cardiovasc Res. (2004) 63:500–9. doi: 10.1016/j.cardiores.2004.03.015
22. Head SJ, Farooq V, Serruys PW, Kappetein AP. The SYNTAX score and its clinical implications. Heart. (2014) 100:169–77. doi: 10.1136/heartjnl-2012-302482
23. Li J, Hsu HC, Mountz JD, Allen JG. Unmasking fucosylation: from cell adhesion to immune system regulation and diseases. Cell Chem Biol. (2018) 25:499–512. doi: 10.1016/j.chembiol.2018.02.005
24. Jiang R, Li H, Yang J, Shen X, Song C, Yang Z, et al. circRNA profiling reveals an abundant circFUT10 that promotes adipocyte proliferation and inhibits adipocyte differentiation via sponging let-7. Mol Ther Nucleic Acids. (2020) 20:491–501. doi: 10.1016/j.omtn.2020.03.011
25. Kumar A, Torii T, Ishino Y, Muraoka D, Yoshimura T, Togayachi A, et al. The Lewis X-related α1,3-fucosyltransferase, Fut10, is required for the maintenance of stem cell populations. J Biol Chem. (2013) 288:28859–68. doi: 10.1074/jbc.M113.469403
26. Li J, Hsu HC, Ding Y, Li H, Wu Q, Yang P, et al. Inhibition of fucosylation reshapes inflammatory macrophages and suppresses type II collagen-induced arthritis. Arthritis Rheumatol. (2014) 66:2368–79. doi: 10.1002/art.38711
27. Markina YV, Gerasimova EV, Markin AM, Glanz VY, Wu WK, Sobenin IA, et al. Sialylated immunoglobulins for the treatment of immuno-inflammatory diseases. Int J Mol Sci. (2020) 21:5472. doi: 10.3390/ijms21155472
28. Uhlén M, Fagerberg L, Hallström BM, Lindskog C, Oksvold P, Mardinoglu A, et al. Tissue-based map of the human proteome. Science. (2015) 347:1260419. doi: 10.1126/science.1260419
29. Gingras S, Pelletier S, Boyd K, Ihle JN. Characterization of a family of novel cysteine- serine-rich nuclear proteins (CSRNP). PLoS ONE. (2007) 2:e808. doi: 10.1371/journal.pone.0000808
30. Yamada K, Akiyama N, Yamada S, Tanaka H, Saito S, Hiraoka M, et al. Taip2 is a novel cell death-related gene expressed in the brain during development. Biochem Biophys Res Commun. (2008) 369:426–31. doi: 10.1016/j.bbrc.2008.02.041
31. Michelis KC, Nomura-Kitabayashi A, Lecce L, Franzén O, Koplev S, Xu Y, et al. CD90 identifies adventitial mesenchymal progenitor cells in adult human medium- and large-sized arteries. Stem Cell Rep. (2018) 11:242–57. doi: 10.1016/j.stemcr.2018.06.001
32. Franzén O, Ermel R, Cohain A, Akers NK, Di Narzo A, Talukdar HA, et al. Cardiometabolic risk loci share downstream cis- and trans-gene regulation across tissues and diseases. Science. (2016) 353:827–30. doi: 10.1126/science.aad6970
33. Jiang M, Shi L, Yang C, Ge Y, Lin L, Fan H, et al. miR-1254 inhibits cell proliferation, migration, and invasion by down-regulating Smurf1 in gastric cancer. Cell Death Dis. (2019) 10:32. doi: 10.1038/s41419-018-1262-x
34. Han J, Shui JW, Zhang X, Zheng B, Han S, Tan TH. HIP-55 is important for T-cell proliferation, cytokine production, and immune responses. Mol Cell Biol. (2005) 25:6869–78. doi: 10.1128/MCB.25.16.6869-6878.2005
35. Liu N, Xing R, Yang C, Tian A, Lv Z, Sun N, et al. HIP-55/DBNL-dependent regulation of adrenergic receptor mediates the ERK1/2 proliferative pathway. Mol Biosyst. (2014) 10:1932–9. doi: 10.1039/c3mb70525k
36. Isserlin R, Merico D, Alikhani-Koupaei R, Gramolini A, Bader GD, Emili A. Pathway analysis of dilated cardiomyopathy using global proteomic profiling and enrichment maps. Proteomics. (2010) 10:1316–27. doi: 10.1002/pmic.200900412
37. Coats CJ, Heywood WE, Virasami A, Ashrafi N, Syrris P, Dos Remedios C, et al. Proteomic analysis of the myocardium in hypertrophic obstructive cardiomyopathy. Circ Genom Precis Med. (2018) 11:e001974. doi: 10.1161/CIRCGEN.117.001974
38. Malik ZA, Kott KS, Poe AJ, Kuo T, Chen L, Ferrara KW, et al. Cardiac myocyte exosomes: stability, HSP60, and proteomics. Am J Physiol Heart Circ Physiol. (2013) 304:H954–65. doi: 10.1152/ajpheart.00835.2012
39. Captur G, Heywood WE, Coats C, Rosmini S, Patel V, Lopes LR, et al. Identification of a multiplex biomarker panel for hypertrophic cardiomyopathy using quantitative proteomics and machine learning. Mol Cell Proteomics. (2020) 19:114–27. doi: 10.1074/mcp.RA119.001586
40. Zhang G, Wang X, Gillette TG, Deng Y, Wang ZV. Unfolded protein response as a therapeutic target in cardiovascular disease. Curr Top Med Chem. (2019) 19:1902–17. doi: 10.2174/1568026619666190521093049
41. Gispert S, Parganlija D, Klinkenberg M, Dröse S, Wittig I, Mittelbronn M, et al. Loss of mitochondrial peptidase Clpp leads to infertility, hearing loss plus growth retardation via accumulation of CLPX, mtDNA and inflammatory factors. Hum Mol Genet. (2013) 22:4871–87. doi: 10.1093/hmg/ddt338
42. Seiferling D, Szczepanowska K, Becker C, Senft K, Hermans S, Maiti P, et al. Loss of CLPP alleviates mitochondrial cardiomyopathy without affecting the mammalian UPRmt. EMBO Rep. (2016) 17:953–64. doi: 10.15252/embr.201642077
43. Chakrabarti S, Kobayashi KS, Flavell RA, Marks CB, Miyake K, Liston DR, et al. Impaired membrane resealing and autoimmune myositis in synaptotagmin VII-deficient mice. J Cell Biol. (2003) 162:543–9. doi: 10.1083/jcb.200305131
44. Huang S, Lu W, Ge D, Meng N, Li Y, Su L, et al. A new microRNA signal pathway regulated by long noncoding RNA TGFB2-OT1 in autophagy and inflammation of vascular endothelial cells. Autophagy. (2015) 11:2172–83. doi: 10.1080/15548627.2015.1106663
45. Ma W, Kang Y, Ning L, Tan J, Wang H, Ying Y. Identification of microRNAs involved in gefitinib resistance of non-small-cell lung cancer through the insulin-like growth factor receptor 1 signaling pathway. Exp Ther Med. (2017) 14:2853–62. doi: 10.3892/etm.2017.4847
46. Higashi Y, Sukhanov S, Shai SY, Danchuk S, Tang R, Snarski P, et al. Insulin-like growth factor-1 receptor deficiency in macrophages accelerates atherosclerosis and induces an unstable plaque phenotype in apolipoprotein E-deficient mice. Circulation. (2016) 133:2263–78. doi: 10.1161/CIRCULATIONAHA.116.021805
47. Hembrom AA, Srivastava S, Garg I, Kumar B. Identification of regulatory microRNAs for hypoxia induced coagulation mechanism by in-silico analysis. bioRxiv [Preprint]. (2020). doi: 10.1101/2020.06.26.173112
48. Neele AE, de Winther MPJ. Repressing the repressor: Ezh2 mediates macrophage activation. J Exp Med. (2018) 215:1269–71. doi: 10.1084/jem.20180479
49. Sweet DR, Fan L, Hsieh PN, Jain MK. Krüppel-like factors in vascular inflammation: mechanistic insights and therapeutic potential. Front Cardiovasc Med. (2018) 5:6. doi: 10.3389/fcvm.2018.00006
50. Luo MC, Zhou SY, Feng DY, Xiao J, Li WY, Xu CD, et al. Runt-related transcription factor 1 (RUNX1) binds to p50 in macrophages and enhances TLR4-triggered inflammation and septic shock. J Biol Chem. (2016) 291:22011–20. doi: 10.1074/jbc.M116.715953
51. van Riel B, Pakozdi T, Brouwer R, Monteiro R, Tuladhar K, Franke V, et al. A novel complex, RUNX1-MYEF2, represses hematopoietic genes in erythroid cells. Mol Cell Biol. (2012) 32:3814–22. doi: 10.1128/MCB.05938-11
52. Lee TI, Young RA. Transcriptional regulation and its misregulation in disease. Cell. (2013) 152:1237–51. doi: 10.1016/j.cell.2013.02.014
53. Leung KK, Hause RJ Jr., Barkinge JL, Ciaccio MF, Chuu CP, Jones RB. Enhanced prediction of Src homology 2 (SH2) domain binding potentials using a fluorescence polarization-derived c-Met, c-Kit, ErbB, and androgen receptor interactome. Mol Cell Proteomics. (2014) 13:1705–23. doi: 10.1074/mcp.M113.034876
54. Maes T, Barceló A, Buesa C. Neuron navigator: a human gene family with homology to unc-53, a cell guidance gene from Caenorhabditis elegans. Genomics. (2002) 80:21–30. doi: 10.1006/geno.2002.6799
55. Muley PD, McNeill EM, Marzinke MA, Knobel KM, Barr MM, Clagett-Dame M. The atRA-responsive gene neuron navigator 2 functions in neurite outgrowth and axonal elongation. Dev Neurobiol. (2008) 68:1441–53. doi: 10.1002/dneu.20670
56. Macgregor-Das AM, Das S. A microRNA's journey to the center of the mitochondria. Am J Physiol Heart Circ Physiol. (2018) 315:H206–15. doi: 10.1152/ajpheart.00714.2017
57. Wang Z, Li C, Sun X, Li Z, Li J, Wang L, et al. Hypermethylation of miR-181b in monocytes is associated with coronary artery disease and promotes M1 polarized phenotype via PIAS1-KLF4 axis. Cardiovasc Diagnosis Ther. (2020) 10:738–51. doi: 10.21037/cdt-20-407
58. Hori D, Dunkerly-Eyring B, Nomura Y, Biswas D, Steppan J, Henao-Mejia J, et al. miR-181b regulates vascular stiffness age dependently in part by regulating TGF-β signaling. PLoS ONE. (2017) 12:e0174108. doi: 10.1371/journal.pone.0174108
59. Copier CU, León L, Fernández M, Contador D, Calligaris SD. Circulating miR-19b and miR-181b are potential biomarkers for diabetic cardiomyopathy. Sci Rep. (2017) 7:13514. doi: 10.1038/s41598-017-13875-2
60. Sikorski V, Karjalainen P, Blokhina D, Oksaharju K, Khan J, Katayama S, et al. Epitranscriptomics of ischemic heart disease-the IHD-EPITRAN study design and objectives. Int J Mol Sci. (2021) 22. Available online at: www.ihd-epitran.com
Keywords: biomarkers, ischemia, chronic ischemic heart disease, cardiovascular surgery, atrial appendage, differentially expressed genes
Citation: Mulari S, Eskin A, Lampinen M, Nummi A, Nieminen T, Teittinen K, Ojala T, Kankainen M, Vento A, Laurikka J, Kupari M, Harjula A, Tuncbag N and Kankuri E (2021) Ischemic Heart Disease Selectively Modifies the Right Atrial Appendage Transcriptome. Front. Cardiovasc. Med. 8:728198. doi: 10.3389/fcvm.2021.728198
Received: 21 June 2021; Accepted: 01 November 2021;
Published: 02 December 2021.
Edited by:
Christian Schulte, University Heart and Vascular Center Hamburg (UHZ), GermanyReviewed by:
Judith Sluimer, Maastricht University, NetherlandsChen Gao, UCLA Department of Anesthesiology & Perioperative Medicine, United States
David Lemar Simpson, Wentworth Institute of Technology, United States
Copyright © 2021 Mulari, Eskin, Lampinen, Nummi, Nieminen, Teittinen, Ojala, Kankainen, Vento, Laurikka, Kupari, Harjula, Tuncbag and Kankuri. This is an open-access article distributed under the terms of the Creative Commons Attribution License (CC BY). The use, distribution or reproduction in other forums is permitted, provided the original author(s) and the copyright owner(s) are credited and that the original publication in this journal is cited, in accordance with accepted academic practice. No use, distribution or reproduction is permitted which does not comply with these terms.
*Correspondence: Esko Kankuri, esko.kankuri@helsinki.fi
†These authors have contributed equally to this work