Abstract
Various anthropogenic activities often result in the emission of carbon dioxide (CO2), which is one of the principal components of greenhouse gases responsible for greenhouse effect. One vital strategy to mitigate the effect of the released CO2 on the environment is through sustainable utilization and conversion to value-added chemicals. This study employs the Radial Basis Function artificial neural network for modeling the prediction of thermo-catalytic CO2 oxidative coupling of methane to C2-hydrocarbons. The various architecture of the Radial Basis Function ANN was developed, trained, and tested using the non-linear relationship between the input parameters (reaction temperature, amount of CaO and MnO in the CaO-MnO/CeO2 catalysts and the CO2/CH4 ratio) and the output parameters (C2 hydrocarbon selectivity and yield). The Radial Basis Function ANN architecture with the topology of 4-20-2, representing the input layer, hidden neurons, and the output layer offers the best performance with a sum of square error (SSE) of 3.9 × 10−24 for training and 0.224 for testing. The R2 of 0.989 and 0.998 obtained for the prediction of the selectivity and the yield of the C2 hydrocarbon is an indication of the robustness of the Radial Basis Function ANN model. The sensitivity analysis revealed that the input parameters significantly influence the model output. However, the reaction temperature has the most significant influence on the model output based on the level of importance.








Similar content being viewed by others
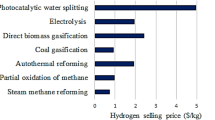
References
Bakonyi P, Peter J, Koter S et al (2020) Possibilities for the biologically-assisted utilization of CO2-rich gaseous waste streams generated during membrane technological separation of biohydrogen. J CO2 Util 36:231–243. https://doi.org/10.1016/j.jcou.2019.11.008
Yaashikaa PR, Senthil Kumar P, Varjani SJ, Saravanan A (2019) A review on photochemical, biochemical and electrochemical transformation of CO2 into value-added products. J CO2 Util 33:131–147. https://doi.org/10.1016/j.jcou.2019.05.017
Parvez AM, Afzal MT, Victor Hebb TG, Schmid M (2020) Utilization of CO2 in thermochemical conversion of biomass for enhanced product properties: a review. J CO2 Util 40:101217. https://doi.org/10.1016/j.jcou.2020.101217
Ayodele BV, Khan MR, Cheng CK (2017) Greenhouse gases mitigation by CO2 reforming of methane to hydrogen-rich syngas using praseodymium oxide supported cobalt catalyst. Clean Techn Environ Policy 19:795–807. https://doi.org/10.1007/s10098-016-1267-z
Skytt T, Nielsen SN, Jonsson B-G (2020) Global warming potential and absolute global temperature change potential from carbon dioxide and methane fluxes as indicators of regional sustainability – a case study of Jämtland, Sweden. Ecol Indic 110:105831. https://doi.org/10.1016/j.ecolind.2019.105831
Khan AA, Tahir M (2019) Recent advancements in engineering approach towards design of photo-reactors for selective photocatalytic CO2 reduction to renewable fuels. J CO2 Util 29:205–239. https://doi.org/10.1016/j.jcou.2018.12.008
Din IU, Shaharun MS, Alotaibi MA et al (2019) Recent developments on heterogeneous catalytic CO2 reduction to methanol. J CO2 Util 34:20–33. https://doi.org/10.1016/j.jcou.2019.05.036
Zhang Z, Zhang L, Hülsey MJ, Yan N (2019) Zirconia phase effect in Pd/ZrO2 catalyzed CO2 hydrogenation into formate. Mol Catal 475:110461. https://doi.org/10.1016/j.mcat.2019.110461
Ye R-P, Li Q, Gong W, Wang T, Razink JJ, Lin L, Qin YY, Zhou Z, Adidharma H, Tang J, Russell AG (2020) High-performance of nanostructured Ni/CeO2 catalyst on CO2 methanation. Appl Catal B Environ 268:118474. https://doi.org/10.1016/j.apcatb.2019.118474
Wang Z, Cao XM, Zhu J, Hu P (2014) Activity and coke formation of nickel and nickel carbide in dry reforming: a deactivation scheme from density functional theory. J Catal 311:469–480. https://doi.org/10.1016/j.jcat.2013.12.015
Shin SA, Noh YS, Hong GH, Park JI, Song HT, Lee KY, Moon DJ (2018) Dry reforming of methane over Ni/ZrO2-Al2O3 catalysts: effect of preparation methods. J Taiwan Inst Chem Eng 90:25–32. https://doi.org/10.1016/j.jtice.2017.11.032
Ayodele BV, Khan MR, Cheng CK (2015) Syngas production from CO2 reforming of methane over ceria supported cobalt catalyst: effects of reactants partial pressure. J Nat Gas Sci Eng 27:1016–1023. https://doi.org/10.1016/j.jngse.2015.09.049
Ayodele BV, Khan MR, Lam SS, Cheng CK (2016) Production of CO-rich hydrogen from methane dry reforming over lanthania-supported cobalt catalyst: kinetic and mechanistic studies. Int J Hydrog Energy 41:4603–4615. https://doi.org/10.1016/j.ijhydene.2016.01.091
Mesrar F, Kacimi M, Liotta LF, Puleo F, Ziyad M (2018) Syngas production from dry reforming of methane over ni/perlite catalysts: effect of zirconia and ceria impregnation. Int J Hydrog Energy 43:17142–17155. https://doi.org/10.1016/j.ijhydene.2018.07.104
Li N, Li Y, Jiang R, Zhou J, Liu M (2019) Photocatalytic coupling of methane and CO2 into C2-hydrocarbons over Zn doped g-C3N4 catalysts. Appl Surf Sci 498:143861. https://doi.org/10.1016/j.apsusc.2019.143861
Ma Y, Harding JD, Tu X (2019) Catalyst-free low temperature conversion of n-dodecane for co-generation of COx−free hydrogen and C2 hydrocarbons using a gliding arc plasma. Int J Hydrog Energy 44:26158–26168. https://doi.org/10.1016/j.ijhydene.2019.08.067
Sundaram KM, Shreehan MM, Olszewski EF (2001) Ethylene. In: Kirk-Othmer encyclopedia of chemical technology. American Cancer Society
Istadi I, Amin NAS (2006) Optimization of process parameters and catalyst compositions in carbon dioxide oxidative coupling of methane over CaO-MnO/CeO2 catalyst using response surface methodology. Fuel Process Technol 87:449–459. https://doi.org/10.1016/j.fuproc.2005.11.004
Istadi I, Amin NAS (2006) Hybrid artificial neural network—genetic algorithm technique for modeling and optimization of plasma reactor hybrid artificial neural network—genetic algorithm technique for modeling and optimization of plasma reactor. Ind Eng Chem Res 45:6655–6664. https://doi.org/10.1021/ie060562c
Koschwitz D, Frisch J, van Treeck C (2018) Data-driven heating and cooling load predictions for non-residential buildings based on support vector machine regression and NARX recurrent neural network: a comparative study on district scale. Energy 165:134–142. https://doi.org/10.1016/j.energy.2018.09.068
Alsaffar MA, Ayodele BV, Mustapa SI (2019) Scavenging carbon deposition on alumina supported cobalt catalyst during renewable hydrogen-rich syngas production by methane dry reforming using artificial intelligence modeling technique. J Clean Prod 119168. https://doi.org/10.1016/j.jclepro.2019.119168
Ayodele BV, Alsaffar MA, Mustapa SI, Vo DN (2020) Back-propagation neural networks modeling of photocatalytic degradation of organic pollutants using TiO2 -based photocatalysts. J Chem Technol Biotechnol 95(10):2739–2749. https://doi.org/10.1002/jctb.6407
Al-Haiqi O, Nour AH, Ayodele BV, Bargaa R (2020) Bayesian regularization-trained multi-layer perceptron neural network predictive modelling of phenol degradation using ZnO/Fe2O3 photocatalyst. J Phys Conf Ser 1529:052058. https://doi.org/10.1088/1742-6596/1529/5/052058
Li H, Zhang Z, Liu Z (2017) Application of artificial neural networks for catalysis: a review. Catalysts 7:306. https://doi.org/10.3390/catal7100306
Ehsani MR, Bateni H, Razi Parchikolaei G (2013) Modeling of oxidative coupling of methane over Mn/Na2Wo4/SiO2 catalyst using artificial neural network. Iran J Chem Chem Eng 32:107–114
Ehsani MR, Bateni H, Parchikolaei GR (2012) Modeling the oxidative coupling of methane using artificial neural network and optimizing of its operational conditions using genetic algorithm. Korean J Chem Eng 29:855–861. https://doi.org/10.1007/s11814-011-0250-y
Huang K, Zhan XL, Chen FQ, Lü DW (2003) Catalyst design for methane oxidative coupling by using artificial neural network and hybrid genetic algorithm. Chem Eng Sci 58:81–87. https://doi.org/10.1016/S0009-2509(02)00432-3
Istadi I, Amin NAS (2006) Hybrid artificial neural network-genetic algorithm technique for modeling and optimization of plasma reactor. Ind Eng Chem Res 45:6655–6664. https://doi.org/10.1021/ie060562c
Hossain MA, Ayodele BV, Cheng CK, Khan MR (2016) Artificial neural network modeling of hydrogen-rich syngas production from methane dry reforming over novel Ni/CaFe2O4 catalysts. Int J Hydrog Energy 41:11119–11130. https://doi.org/10.1016/j.ijhydene.2016.04.034
Hossain MS, Ong ZC, Ismail Z, Khoo SY (2017) A comparative study of vibrational response based impact force localization and quantification using radial basis function network and multilayer perceptron. Expert Syst Appl 85:87–98. https://doi.org/10.1016/j.eswa.2017.05.027
Garson GD (1991) Comparison of neural network analysis of social science data. Soc Sci Comput Rev 9:399–434
Sunphorka S, Chalermsinsuwan B, Piumsomboon P (2017) Application of artificial neural network for kinetic parameters prediction of biomass oxidation from biomass properties. J Energy Inst 90:51–61. https://doi.org/10.1016/j.joei.2015.10.007
Chen D, Lødeng R, Anundskås A et al (2001) Deactivation during carbon dioxide reforming of methane over Ni catalyst: microkinetic analysis. Chem Eng Sci 56:1371–1379. https://doi.org/10.1016/S0009-2509(00)00360-2
Ginsburg JM, Piña J, El Solh T, De Lasa HI (2005) Coke formation over a nickel catalyst under methane dry reforming conditions: thermodynamic and kinetic models. Ind Eng Chem Res 44:4846–4854. https://doi.org/10.1021/ie0496333
Abdolahi F, Mortazavi Y, Khodadadi A et al (2005) Modeling of methane oxidative coupling under periodic operation by neural network. Chem Eng Technol 28:581–586. https://doi.org/10.1002/ceat.200407041
Han H-G, Qiao J-F, Chen Q-L (2012) Model predictive control of dissolved oxygen concentration based on a self-organizing RBF neural network. Control Eng Pract 20:465–476. https://doi.org/10.1016/j.conengprac.2012.01.001
Alsaffar MA, Ayodele BV, Mustapa SI (2020) Scavenging carbon deposition on alumina supported cobalt catalyst during renewable hydrogen-rich syngas production by methane dry reforming using artificial intelligence modeling technique. J Clean Prod 247:119168. https://doi.org/10.1016/j.jclepro.2019.119168
Hashemi Fath A, Madanifar F, Abbasi M (2020) Implementation of multilayer perceptron (MLP) and radial basis function (RBF) neural networks to predict solution gas-oil ratio of crude oil systems. Petroleum 6:80–91. https://doi.org/10.1016/j.petlm.2018.12.002
Crowley JN, CampuzanoJost P, Moortgat GK (1996) Temperature dependent rate constants for the gas-phase reaction between OH and CH3OCl. J Phys Chem 100:3601–3606
Author information
Authors and Affiliations
Corresponding author
Additional information
Publisher's Note
Springer Nature remains neutral with regard to jurisdictional claims in published maps and institutional affiliations.
Rights and permissions
About this article
Cite this article
Ayodele, B.V., Mustapa, S.I., Witoon, T. et al. Radial Basis Function Neural Network Model Prediction of Thermo-catalytic Carbon Dioxide Oxidative Coupling of Methane to C2-hydrocarbon. Top Catal 64, 328–337 (2021). https://doi.org/10.1007/s11244-020-01401-0
Accepted:
Published:
Issue Date:
DOI: https://doi.org/10.1007/s11244-020-01401-0