Abstract
The emerging technology of autonomous vehicles has been widely recognized as a promising urban mobility solution in the future. This paper considers the integration of autonomous vehicles into bus transit systems and proposes a modeling framework to determine the optimal bus fleet size and its assignment onto multiple bus lines in a bus service network considering uncertain demand. The mixed-integer stochastic programming approach is applied to formulate the problem. We apply the sample average approximation (SAA) method to solve the formulated stochastic programming problem. To tackle the nonconvexity of the SAA problem, we first present a reformulation method that transforms the problem into a mixed-integer conic quadratic program (MICQP), which can be solved to its global optimal solution by using some existing solution methods. However, this MICQP based approach can only handle the small-size problems. For the cases with large problem size, we apply the approach of quadratic transform with linear alternating algorithm, which allows for efficient solution to large-scale instances with up to thousands of scenarios in a reasonable computational time. Numerical results demonstrate the benefits of introducing autonomous buses as they are flexible to be assigned across different bus service lines, especially when demand uncertainty is more significant. The introduction of autonomous buses would enable further reduction of the required fleets and total cost. The model formulation and solution methods proposed in this study can be used to provide bus transit operators with operational guidance on including autonomous buses into bus services, especially on the autonomous and conventional bus fleets composition and allocation.






Similar content being viewed by others
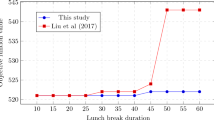
References
Abe, R.: Introducing autonomous buses and taxis: quantifying the potential benefits in japanese transportation systems. Transp. Res. Part A Policy Pract. 126, 94–113 (2019)
Aldaihani, M.M., Quadrifoglio, L., Dessouky, M.M., Hall, R.: Network design for a grid hybrid transit service. Transp. Res. Part A Policy Pract. 38(7), 511–530 (2004)
Alizadeh, F., Goldfarb, D.: Second-order cone programming. Math. Program. 95(1), 3–51 (2003)
An, K., Lo, H.K.: Two-phase stochastic program for transit network design under demand uncertainty. Transp. Res. Part B Methodol. 84, 157–181 (2016)
Beaujon, G.J., Turnquist, M.A.: A model for fleet sizing and vehicle allocation. Transp. Sci. 25(1), 19–45 (1991)
Ben-Tal, A., Nemirovski, A.: Lectures on Modern Convex Optimization: Analysis, Algorithms, and Engineering Applications, vol. 2. Siam, Peninsula (2001)
Benson, H.: On the global optimization of sums of linear fractional functions over a convex set. J. Optim. Theory Appl. 121(1), 19–39 (2004)
Bergqvist, O., Åstrand, M.: Bus line optimisation using autonomous minibuses (2017). https://www.divaportal.org/smash/get/diva2:1120402/FULLTEXT01.pdf
CAN News: Singapore: More Bus Drivers Needed in Next Five Years. https://www.channelnewsasia.com/news/singapore (2017). Accessed 26 June 2019
Ceder, A.: Estimation of fleet size for variable bus schedules. Transp. Res. Rec. 1903(1), 2–10 (2005)
Chen, P.W., Nie, Y.M.: Optimal design of demand adaptive paired-line hybrid transit: case of radial route structure. Transp. Res. part E Logist. Transp. Rev. 110, 71–89 (2018)
Chen, A., Zhou, Z., Chootinan, P., Ryu, S., Yang, C., Wong, S.: Transport network design problem under uncertainty: a review and new developments. Transp. Rev. 31(6), 743–768 (2011)
Chen, J., Liu, Z., Zhu, S., Wang, W.: Design of limited-stop bus service with capacity constraint and stochastic travel time. Transp. Res. part E Logist. Transp. Rev. 83, 1–15 (2015)
Clark, C.E.: The greatest of a finite set of random variables. Oper. Res. 9(2), 145–162 (1961)
Council, A.T.: National guidelines for transport system management in Australia. Backgr. Mater. 5, Volume 4: Urban Transport, pp. 179–211 (2006)
Dandapat, S., Maitra, B.: An approach for identifying optimal service for rural bus routes. Case Stud. Transp. Policy 3(3), 287–294 (2015)
Dong, X., DiScenna, M., Guerra, E.: Transit user perceptions of driverless buses. Transportation 46(1), 35–50 (2019)
Geetha, S., Nair, K.: A variation of the assignment problem. Eur. J. Oper. Res. 68(3), 422–426 (1993)
Gentile, G., Noekel, K.: Modelling Public Transport Passenger Flows in the Era of Intelligent Transport Systems. Springer, Gewerbestrasse (2016)
Huang, Z., Ren, G., Liu, H.: Optimizing bus frequencies under uncertain demand: case study of the transit network in a developing city. Math. Prob. Eng. (2013). https://doi.org/10.1155/2013/375084
Jansson, J.O.: A simple bus line model for optimisation of service frequency and bus size. J. Transp. Econ. Policy 14(1), 53–80 (1980)
Jara-Díaz, S., Fielbaum, A., Gschwender, A.: Optimal fleet size, frequencies and vehicle capacities considering peak and off-peak periods in public transport. Transp. Res. Part A Policy Pract. 106, 65–74 (2017)
Jiménez, F., Román, A.: Urban bus fleet-to-route assignment for pollutant emissions minimization. Transp. Res. Part E Logist. Transp. Rev. 85, 120–131 (2016)
Ker, K., Kuo, H., Schondeld, M.: Optimal mixed bus fleet for urban operations. Transp. Res. Rec. 1503, 39–48 (1995)
Kim, M., Schonfeld, P.: Conventional, flexible, and variable-type bus services. J. Transp. Eng. 138(3), 263–273 (2012)
Kim, M.E., Schonfeld, P.: Integrating bus services with mixed fleets. Transp. Res. Part B Methodol. 55, 227–244 (2013)
Kim, M.E., Schonfeld, P.: Integration of conventional and flexible bus services with timed transfers. Transp. Res. Part B Methodol. 68, 76–97 (2014)
Kleywegt, A.J., Shapiro, A., Homem-de Mello, T.: The sample average approximation method for stochastic discrete optimization. SIAM J. Optim. 12(2), 479–502 (2002)
Li, X., Quadrifoglio, L.: Optimal zone design for feeder transit services. Transp. Res. Rec. 2111(1), 100–108 (2009)
Li, X., Quadrifoglio, L.: Feeder transit services: choosing between fixed and demand responsive policy. Transp. Res. Part C Emerg. Technol. 18(5), 770–780 (2010)
Li, Z.-C., Lam, W.H., Sumalee, A.: Modeling impact of transit operator fleet size under various market regimes with uncertainty in network. Transp. Res. Rec. 2063(1), 18–27 (2008)
Li, Z.-C., Lam, W.H., Wong, S.: On the allocation of new lines in a competitive transit network with uncertain demand and scale economies. J. Adv. Transp. 45(4), 233–251 (2011)
Li, Z.-C., Lam, W.H., Wong, S.: Optimization of number of operators and allocation of new lines in an oligopolistic transit market. Netw. Spat. Econ. 12(1), 1–20 (2012a)
Li, Z.-C., Lam, W.H., Wong, S., Sumalee, A.: Environmentally sustainable toll design for congested road networks with uncertain demand. Int. J. Sustain. Transp. 6(3), 127–155 (2012b)
Lin, Y.H., Wang, Y., He, D., Lee, L.H.: Last-mile delivery: Optimal locker location under multinomial logit choice model. Transp. Res. Part E Logist. Transp. Rev. 142, 102059 (2020)
Liu, H., Wang, D.Z.: Global optimization method for network design problem with stochastic user equilibrium. Transp. Res. Part B Methodol. 72, 20–39 (2015)
Liu, Z., Yan, Y., Qu, X., Zhang, Y.: Bus stop-skipping scheme with random travel time. Transp. Res. Part C Emerg. Technol. 35, 46–56 (2013)
Long, Y., Lee, L.H., Chew, E.P.: The sample average approximation method for empty container repositioning with uncertainties. Eur. J. Oper. Res. 222(1), 65–75 (2012)
Luathep, P., Sumalee, A., Lam, W.H., Li, Z.-C., Lo, H.K.: Global optimization method for mixed transportation network design problem: a mixed-integer linear programming approach. Transp. Res. Part B Methodol. 45(5), 808–827 (2011)
Nayan, A., Wang, D.Z.: Optimal bus transit route packaging in a privatized contracting regime. Transp. Res. Part A Policy Pract. 97, 146–157 (2017)
Ongel, A., Loewer, E., Roemer, F., Sethuraman, G., Chang, F., Lienkamp, M.: Economic assessment of autonomous electric microtransit vehicles. Sustainability 11(3), 648 (2019)
Patil, G.R., Ukkusuri, S.V.: Sample average approximation technique for flexible network design problem. J. Comput. Civ. Eng. 25(3), 254–262 (2011)
Pinto, H.K., Hyland, M.F., Mahmassani, H.S., Verbas, I.Ö.: Joint design of multimodal transit networks and shared autonomous mobility fleets. Transp. Res. Part C Emerg. Technol. 111, 255–293 (2020)
Salonen, A.O.: Passenger’s subjective traffic safety, in-vehicle security and emergency management in the driverless shuttle bus in Finland. Transp. Policy 61, 106–110 (2018)
Salzborn, F.J.: Optimum bus scheduling. Transp. Sci. 6(2), 137–148 (1972)
Shapiro, A., Philpott, A.: A tutorial on stochastic programming. www2.isye.gatech.edu/ashapiro/publications.html, 17 (2007)
Shen, K., Yu, W.: Fractional programming for communication systems–part i: power control and beamforming. IEEE Trans. Signal Process. 66(10), 2616–2630 (2018)
Shen, Y., Zhang, H., Zhao, J.: Integrating shared autonomous vehicle in public transportation system: a supply-side simulation of the first-mile service in Singapore. Transp. Res. Part A Policy Pract. 113, 125–136 (2018)
Silman, L.A., Barzily, Z., Passy, U.: Planning the route system for urban buses. Comput. Oper. Res. 1(2), 201–211 (1974)
Szeto, W.Y., Jiang, Y.: Hybrid artificial bee colony algorithm for transit network design. Transp. Res. Rec. 2284(1), 47–56 (2012)
Szeto, W.Y., Jiang, Y.: Transit route and frequency design: Bi-level modeling and hybrid artificial bee colony algorithm approach. Transp. Res. Part B Methodol. 67, 235–263 (2014)
Szeto, W.Y., Wu, Y.: A simultaneous bus route design and frequency setting problem for tin Shui Wai, Hong Kong. Eur. J. Oper. Res. 209(2), 141–155 (2011)
Tirachini, A., Antoniou, C.: The economics of automated public transport: effects on operator cost, travel time, fare and subsidy. Econ. Transp. 21, 100151 (2020)
Wang, D.Z., Lo, H.K.: Multi-fleet ferry service network design with passenger preferences for differential services. Transp. Res. Part B Methodol. 42(9), 798–822 (2008)
Wang, D.Z., Lo, H.K.: Global optimum of the linearized network design problem with equilibrium flows. Transp. Res. Part B Methodol. 44(4), 482–492 (2010)
Wang, D.Z., Liu, H., Szeto, W.: A novel discrete network design problem formulation and its global optimization solution algorithm. Transp. Res. Part E Logis. Transp. Rev. 79, 213–230 (2015)
Wang, D.Z., Nayan, A., Szeto, W.: Optimal bus service design with limited stop services in a travel corridor. Transp. Res. Part E Logis. Transp. Rev. 111, 70–86 (2018)
Wen, J., Chen, Y.X., Nassir, N., Zhao, J.: Transit-oriented autonomous vehicle operation with integrated demand-supply interaction. Transp. Res. Part C Emerg. Technol. 97, 216–234 (2018)
Wien, J.: An assessment of the willingness to choose a self-driving bus for an urban trip: a public transport user’s perspective. http://resolver.tudelft.nl/uuid:8064cc17-dc0e-4c0c-9a9c-6efca8564d94 (2019)
Winter, K., Wien, J., Molin, E., Cats, O., Morsink, P., van Arem, B.: Taking the self-driving bus: a passenger choice experiment. In: 2019 6th International Conference on Models and Technologies for Intelligent Transportation Systems (MT-ITS), pp 1–8. IEEE (2019)
Yoo, G.-S., Kim, D.-K., Chon, K.S.: Frequency design in urban transit networks with variable demand: model and algorithm. KSCE J. Civ. Eng. 14(3), 403–411 (2010)
Yu, B., Yang, Z.-Z., Jin, P.-H., Wu, S.-H., Yao, B.-Z.: Transit route network design-maximizing direct and transfer demand density. Transp. Res. Part C Emerg. Technol. 22, 58–75 (2012)
Zak, J., Jaszkiewicz, A., Redmer, A.: Multiple criteria optimization method for the vehicle assignment problem in a bus transportation company. J. Adv. Transp. 43(2), 203–243 (2009)
Zhang, W., Jenelius, E., Badia, H.: Efficiency of semi-autonomous and fully autonomous bus services in trunk-and-branches networks. J. Adv. Transp. (2019). https://doi.org/10.1155/2019/7648735
Acknowledgements
This work is supported by Singapore Ministry of Education Academic Research Fund MOE2017-T2-2-093.
Author information
Authors and Affiliations
Contributions
QT Drafting of manuscript, model formulation and solution method design. YHL Computational experiments and solution analysis. DZWW Study conception and critical revision.
Corresponding author
Ethics declarations
Conflict of interest
On behalf of all authors, the corresponding author states that there is no conflict of interest.
Additional information
Publisher's Note
Springer Nature remains neutral with regard to jurisdictional claims in published maps and institutional affiliations.
Appendix 1
Appendix 1
Minimizing the objective function is equivalent to maximizing the following function:
where Z(P2) is the objective function of P2. Define a variable y such that \(y_{l,r} = \frac{\sqrt{\delta (f_l^c + f_{l,r}^a) - k s_{l,r}}}{f_l^c + f_{l,r}^a}\). (40) is equivalent to
since plugging \(y_{l,r}\) into (41) will lead to (40). Finally, define function \(h(f_l^c, f_{l,r}^a,s_{l,r}) = \sqrt{\delta (f_l^c + f_{l,r}^a) - k s_{l,r}}\), we can rewrite the formulation in the hypograph form of h, i.e., \(\beta \le h\), leading to the formulation in P4. Note that, to ensure the square root function is well-defined, \(\delta \) should be chosen such that \(\delta (f_l^c + f_{l,r}^a) - k s_{l,r} > 0, \forall l \in I, r \in R\).
Rights and permissions
About this article
Cite this article
Tian, Q., Lin, Y.H. & Wang, D.Z.W. Autonomous and conventional bus fleet optimization for fixed-route operations considering demand uncertainty. Transportation 48, 2735–2763 (2021). https://doi.org/10.1007/s11116-020-10146-4
Published:
Issue Date:
DOI: https://doi.org/10.1007/s11116-020-10146-4