Predicting the Dispersal and Accumulation of Microplastic Pellets Within the Estuarine and Coastal Waters of South-Eastern Brazil Using Integrated Rainfall Data and Lagrangian Particle Tracking Models
- 1Commonwealth Scientific and Industrial Research Organisation, Oceans and Atmosphere, Canberra, NSW, Australia
- 2Oceanographic Institute, University of São Paulo, São Paulo, Brazil
- 3Oceanology Institute, Environment Ministry, La Habana, Cuba
- 4Institute of Health Sciences, Paulista University, São Paulo, Brazil
Understanding how microplastic particles move and accumulate within estuarine and coastal waters requires consideration of primary inputs (e.g., raw materials from industrial zones) as well as secondary inputs resulting from fluvial processes (i.e., materials carried into coastal waters by rivers and streams). This study presents a novel approach to achieve this aim, by comparing the individual and combined ability of Particle Tracking Models (PTMs) and seasonal rainfall data, to explain observed inputs of microplastic pellets to the ocean beaches of Santos City (south-eastern Brazil). A Lagrangian PTM based on high-resolution hydrodynamic models was used to simulate seasonal patterns of pellet dispersal from five release points within the Santos Estuarine System (SES) and nearshore waters which are known contributors to the regions microplastic debris problem. Model outputs suggested that the debris field is likely to be small within the estuary (ranging from 3.6 to 8.1 km2), intermediate at the river mouth (mean 34 km2) and greatest for near- and offshore sites (ranging from 34 to 40 km2). The spatial footprints were strongly modulated by season (and rainfall), with simulations alone unable to reconcile daily inputs of pellets observed on the beaches of Santos Bay (ranging from 2 to 51 particles m2 ⋅ d–1). Given this discrepancy, a Generalized Additive Modeling approach was employed to integrate the PTM outputs with rainfall data to improve predictions of beached particles. Results confirmed that considering fluvial processes, could significantly improve the ability to predict rates of pellet accumulation (raising the explained deviance in observed inputs from 41 to 93%). Thus, the study highlights the potential to couple widely used dispersion models with metrics that describe fluvial forcing (rainfall and estuarine flushing) in order to better understand the spatio-temporal dynamics of microplastic debris transport and accumulation within dynamic coastal environments.
Introduction
The accumulation of plastics in the biosphere is emerging as one of the most pervasive and intractable pollution problems facing the planet (Barnes et al., 2009). It has recently been estimated that 359 million tons of plastic are produced annually (PlasticsEurope, 2019) and a proportion of this waste ultimately finds its way into coastal and marine ecosystems (GESAMP, 2020). Microplastics, which are a subset of plastic particles that have an upper size limit of 5 mm (GESAMP, 2019) can include primary plastics (e.g., resin pellets for manufacturing, microbeads used in personal care products), as well as secondary materials that have broken down from larger items (Barnes et al., 2009). These particles, are now found in all of the world’s oceans, beaches and deep sea environments (Lebreton et al., 2012; Van Sebille et al., 2015; Zhang C. N. et al., 2020; Zhang D. D. et al., 2020) and can affect the health and survival of biota through accidental ingestion as well as by transferring hazardous chemicals (GESAMP, 2020). Indeed, there is increasing evidence that microplastics are entering marine foodwebs through zooplankton communities (Frias et al., 2014), mussels (Browne et al., 2008), corals (Hall et al., 2015), pelagic and demersal fish (Lusher et al., 2013), seabirds (Thiel et al., 2018), whales (Fossi et al., 2012) and even human beings (with a recent report suggesting that the average person ingests the equivalent of a credit card worth of plastic per year; De Wit and Bigaud, 2019).
A major impediment to managing and mitigating the problem of microplastic pollution in the marine environment is the incomplete understanding of processes that govern dispersal and accumulation (GESAMP, 2015). Although microplastics enter coastal ecosystems from both land and sea as a result of accidental spills (e.g., at stages of production, transportation and processing; Ogata et al., 2009) and the release of residential and industrial wastes (EPA, 1990; Cabral, 2014; Do Sul et al., 2014), they can also be swept into rivers, estuaries and the sea by fluvial (river born) processes and stormwater runoff (GESAMP, 2015) that are strongly influenced by seasonal rainfall. Indeed, a recent study suggested that up to 80% of the plastics entering the oceans do so through river networks (Lebreton et al., 2017). Not only this, but because a considerable proportion of microplastics are buoyant (i.e., often showing exponential decreases with depth; Kooi et al., 2016), they disperse widely in the open ocean as a result of phenomena such as surface currents, stokes drift and mesoscale eddies (Iwasaki et al., 2017; Onink et al., 2019). Closer to shore, patterns of accumulation on beaches and other habitats can be modulated by a range of factors including; terrestrial runoff, release point, hydrodynamics (i.e., tides and currents), weather, coastal geomorphology and a subsequent suite of physical, chemical and biological processes (Frere et al., 2017). Additionally, they can become trapped in sediments, riparian vegetation (e.g., mangroves and tidal marshes; Viehman et al., 2011; Debrot et al., 2013) and even human infrastructure (e.g., coastal dwellings that are common in many poorer countries), only to be remobilized as a result of fluvial processes driven by rainfall. This may be more pronounced in tropical and subtropical regions that experience strongly seasonal rainfall (Krelling et al., 2017) that can release microplastic particles that have been trapped in often dense estuarine vegetation and deep sediments (which characterize these regions) during more stable and dry periods (Ockelford et al., 2020). The failure of dispersal models to include such processes, may present an impediment to gauging risks to the environment, animal and human health, and may be exacerbated in the future given an increase in global flood risk and increased likelihood of extreme weather events.
Although understanding the spatial and temporal dynamics of microplastic accumulation has proven notoriously difficult (Fisner et al., 2017), the increasing resolution and performance of hydrological models is helping to address such challenges and inform policy (Braunschweig et al., 2003; Mateus et al., 2012; Krelling et al., 2017). While early studies focused on identifying point sources (Martinez et al., 2009; Lebreton et al., 2012; Maximenko et al., 2012; Reisser et al., 2013), there has been a shift toward understanding the effect of diffuse entry points and the consequences for debris accumulation within estuarine, coastal and marine habitats (Hardesty et al., 2017). Nevertheless, relying on simulation models alone can lead to a decoupling with reality if predictions are not validated against field observations and/or do not take into account stochastic processes like fluvial forcing (e.g., stormwater and surface runoff) which govern the input and/or reintroduction of particles from terrestrial sources that contribute to patterns of accumulation. Matching high resolution hydrodynamic models, with particle tracking approaches and local catchment characteristics such as rainfall may hold the potential to better reflect the spatio-temporal complexity of microplastic debris within coastal ecosystems to better inform management and intervention strategies (Ballent et al., 2013).
The goal of this study was to use hydrodynamic models and a simple Particle Tracking Model (PTM) to simulate the dispersal of microplastic pellets through the estuarine and coastal waters of the Santos region (south-eastern Brazil). The site was selected due to its complex geomorphological characteristics, strongly seasonal climatic and oceanographic conditions, and because it is home to the largest port in South America (Lamparelli, 1998) which contains several important manufacturing zones that involve the production and/or use of microplastic pellets that are often accidentally released into the environment. The aims of the study were threefold: (1) to simulate the effect of season and different release points on the dispersal footprint (km2) of pellets within the region, (2) to examine whether predicted dispersal area correlates with rainfall, and (3) to combine the outputs of the PTM with rainfall data and beach morphology (zonation) in order to improve predictions of daily rates of pellet accumulation (no. particles m2⋅d–1) on the beaches of Santos Bay.
Materials and Methods
Study Area
The study involved the estuarine channels and ocean beaches of the Santos region, Brazil (Figure 1) that included five simulated release points in the upper and middle estuary (sites A and B), river mouth (site C), inshore and offshore zones (sites D and E). The Santos Estuarine System (SES) is formed by the confluence of the Bertioga Channel Estuary, Port of Santos Channel Estuary and São Vicente Estuary and covers an area of approximately 44 km2 (Moser et al., 2005). The dominant vegetation type is mangrove forest (CETEC, 2000), of which there has been substantial loss since the 1980s as a result of urban and industrial development (Gorman, 2018). The SES is home to the largest port in Latin America and is heavily impacted by industrial activity from the Cubatão industrial complex (refineries and petrochemicals) located less than 20 km inland, in addition to three main industrial areas within the SES itself.
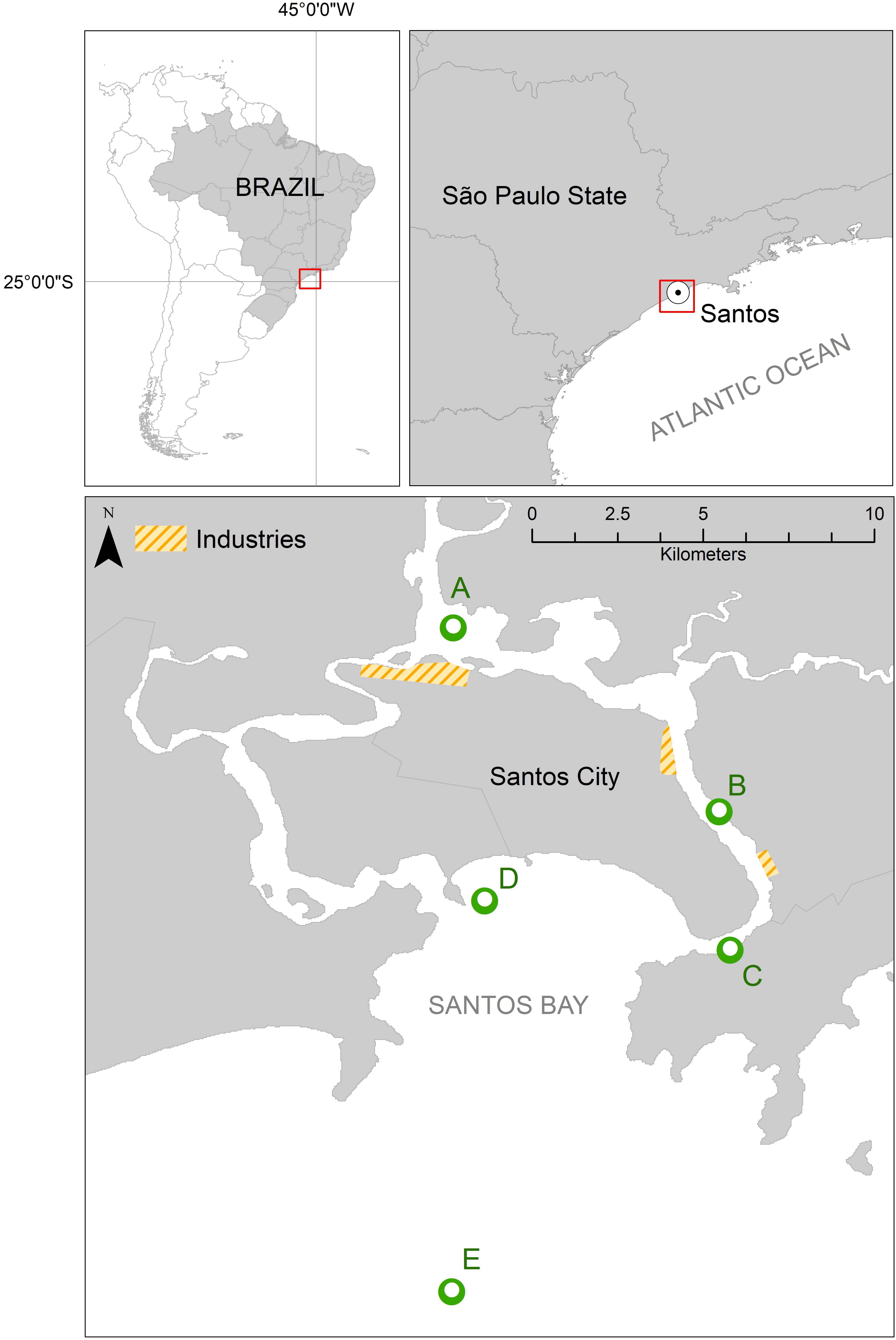
Figure 1. Details of the Santos Estuarine System (SES), within the Central Region of São Paulo State, in south eastern Brazil. Letters give modeled release points: A (upper estuary), B (mid estuary), C (estuary mouth), D (inshore beach) and E (offshore) for microplastic pellets. Hashing indicates areas with a high concentration of industries that produce and/or consume microplastic pellets.
Tide is the most important hydrological factor, with tidal ebb currents having a mean speed of ca. 1 m⋅s–1 (Moser et al., 2005), but may reach up to 1.5 m⋅s–1 as a result of strong winds from the NE and SW directions. Winds and resulting currents show marked seasonal difference with winds from the east, water moving to south during August to November, while winds predominate from the south, resulting in water moving to the west/coast during April to July. The ocean-shelf dynamics off the Santos region is influenced by coastal winds and tidal currents that are governed by coastline geomorphology and bathymetry (Harari et al., 2000, 2006; Harari and Gordon, 2001). The ocean beaches of Santos Bay have a mean tidal range of 1.5 m and a study by Harari et al. (2008) suggested that in absence of significant meteorological effects, tidal circulation is a good approximation of total circulation. The outflow of materials from the SES to Santos Bay is also influenced by the dynamics of the estuary mouth which is approximately 500 m wide and 12 m deep. The region experiences strong seasonal weather patterns, with a typically dry and stable period from August to November, a pronounced summer “wet season” from December to March (thunderstorms) and a winter period of strong frontal systems (sea storms) that peak in April to July. During the summer wet season, stormwater is discharged directly onto the beaches of Santos Bay through a series of six canals which has the effect of creating distinctly different beach morphological types (i.e., becoming wider with a more gradual slope toward the west) which has been shown to have a profound effect on the accumulation patterns along the beach (Balthazar-Silva, 2016).
Port and industrial activities are known sources of pellets to the SES and broader Santos region, with four of Brazil’s largest plastics producing factories being located within the study area. The greatest concentration of industries that produce and/or use polyethylene, PVC and polypropylene pellets (estimated production of 350,000 tons year–1) is centered in the industrial district within the inner SES called Alemoa (Manzano, 2009). In order to assess the influence of other potential release points, a preliminary survey was conducted [following the protocols of the U.S. Environmental Protection Agency (1992)] of the number of industries that produce or use pellets within the industrial districts of Alemoa and the city of Cubatão; the middle reaches of Santos Harbor and Guarujá; those that enter via terrestrial runoff and stormwater outflow through beach channels and potential offshore inputs resulting from shipping activities (Manzano, 2009). Overall, 5 potential release points were chosen (Table 1) that coincide with the “upper estuary” (i.e., adjacent site A), “middle estuary (site B), “river mouth” (site C), “inshore beach” (site D) and “offshore” (site E) and attribute the main sources of inputs to each. While in reality, the contribution of primary inputs from these points is likely to differ (due to the spatial concentration of industries), no weighting was applied in order to provide an unbiased assessment of the cumulative input throughout the SES and adjacent coastal waters of Santos Bay.

Table 1. Summary of simulated release points within the SES and nearshore zones, potential sources, and modeled dispersal area.
Modeling Particle Dispersal
The Lagrangian particle tracking model developed by Harari and Gordon (2001) was used to simulate the dispersal of plastic particles from the five release points. This model incorporated the hydrological features of the SES and neighboring coastline (i.e., tides, currents, particle density and wind) to simulate seasonal patterns of dispersal over a single year (see: Harari et al., 2000). The adaptations of the current model to Harari et al. (2000), involved the use of flexible code to combine hydrological models of different spatial resolutions (i.e., a coastal shelf model with a resolution of 1 km, and a nested estuarine model with a resolution of 100 m) into a single model sufficient to resolve the particle tracking within the predicted debris field (described below). The typical formulation of a Lagrangian model treats any pollutant as a set of particles moving through a bi-dimensional space (here representing the water surface), with zonal and meridional directions (x, y). Particles are randomly released from a sub-region within the domain (here, potential release points within the SES) at the initial time of the simulation. Subsequently, for each time-step Δt, each individual particle P has its original position x(P) and y(P) updated to a new position x(Pnew) and y(Pnew), depending on the interpolated current vectors (u, v), at that time and at that point and including a random variation of the particles position x(Prandom) and y(Prandom) which represent the diffusion effect. The model can be summarized using the equations:
where u and v are computed by the underlying hydrodynamic model and x(Prandom) and y(Prandom) are derived from a random number generator with an interval [−1,+1], multiplied by the maximum displacement allowed for any particle in one time step Δt. In this way, the model employed polynomial interpolations, both horizontally and vertically, to estimate the value of currents between grid meshes and store the origin and trajectory information (u and v). While the effect of waves and Stokes drift can be important when modeling microplastics for open ocean areas and at higher latitudes such as the Arctic and Sea of Japan (Iwasaki et al., 2017), their effect in subtropical regions is thought to be less (Onink et al., 2019) and was therefore not considered in the present study.
The particle tracking model employed a different formulation for the horizontal eddy diffusivity than the underlying hydrodynamic model. The approach considered the relationship between maximum displacement in a time step and the diffusivity coefficient, while the hydrodynamic model considered the Smagorinsky formulation for horizontal diffusion. Particle diffusion was simulated using the well-known random walk method (Rubinstein and Kroese, 2016), considering a function of uniform probability density, with zero mean and unitary standard deviation which meant that the maximum displacement of each particle, for each time interval, is about 50 m, corresponding to a typical diffusion coefficient in this area between 10 and 100 m2 s–1, as computed by Ghisolfi and Garcia (1996). This computation is equivalent to a coefficient of the diffusion equation corresponding to (N L2/2) where N is the number of displacements per time unit and L is the maximum displacement allowed at every walk (Bowden, 1983). The model included “beaching” whenever an individual particle reached dry cells (i.e., crossing a land boundary and leaving the fluid field), whereby it was fixed in space and not subject to any further advection, diffusion or decay (i.e., and thus the model did not include wetting and drying). The model did not incorporate sinking of particles, as most types of polyethylene resin pellets encountered in the study region float on or just below the seawater surface. Overall, the simulations were designed to evaluate the intra-annual (seasonal) variability in pellet dispersal and forecast the progress of the debris field across a typical year.
The current field was provided by the three-dimensional hydrodynamic numerical model adapted by Harari et al. (2000). This numerical model is based on the Princeton Ocean Model (POM) developed by Blumberg and Mellor (1987) and presented in detail by Mellor (1998). POM is a three-dimensional model of primitive equations, with time-varying free surface and sigma vertical coordinates. The prognostic variables are the three components of velocity, in addition to temperature and salinity. The model grid for the São Paulo State shelf contains 150 × 450 points and 11 sigma levels, with a uniform resolution of 1 km in the horizontal plane, and time steps of 180 and 6 s, for the internal and external modes. The main advantage of the implemented model configuration is the possibility of using nested grids in estuarine regions, such as the Santos study region.
The model was run for the entire year of 2008 considering mean monthly conditions of temperature and salinity, extracted from Levitus and Boyer (1994); typical river discharges (DAEE, 1999); the tidal elevations at the boundaries based on results of the global tidal model (Leprovost et al., 1994), offshore tidal measurements (De Mesquita and Harari, 2003); mean sea level oscillations from the Oceanographic Institute of São Paulo University and surface wind conditions from the global atmospheric model of NCEP / NCAR, available at http://www.cdc.noaa.gov/cdc/data.ncep.reanalysis.html. The bathymetry of the grid layer was obtained from the global database GEBCO bathymetry (General bathymetric chart of the Oceans)1, which was merged with local bathymetric charts obtained from the Brazilian Geography and Statistics Institute database2. The total prediction area encompassed 232 km2 of coastal and estuarine waters within the SES. Model outputs were integrated into ArcGIS version 10.1 (Environmental Systems Research Institute, Inc., Redlands, CA, United States) and used to calculate the total dispersal area (km2) for each month of the year. Because the model represented a simulation of particle transport (i.e., no actual particles were released) outputs were calibrated and validated only qualitatively, based on the assessments of pellets inputs along the beaches of Santos Bay (described in the next section). The discrete particle field was mapped into a continuous field using the “Point Density” function in the ArcGIS Spatial Analyst toolbox, which calculates a magnitude-per-unit area (m2) from points using a kernel function to fit a smoothly tapered surface to each point.
Predicting Rates of Pellet Accumulation on the Beaches of Santos Bay
To test if the dispersal models could predict rates of pellet accumulation on the beaches of Santos Bay, model outputs were compared with daily inputs of pellets (no. m2 ⋅ d–1) sampled at six points along the beaches of Santos Bay during “stable weather” (November), at the “end of the wet season” (March), and during the “storm season” (July). Pellet accumulation was quantified according to the standard protocols for micro-litter (GESAMP, 2019) which involved the marking out and preparation (24 h prior to sampling), of 2-m wide fixed transects (n = 5 replicates) established at 6 sites along the beaches of Santos Bay (separated by ∼1 km). Preparation involved pre-cleaning all surface debris (plastics, wood, vegetation) from the water’s edge to the high strandline using a 50 cm wide squeegee to establish a virgin surface from which to evaluate the daily accumulation rate. Sampling was done using the same squeegee, to concentrate and collect all beached debris for separation and identification. This approach has been used previously to measure pellet inputs to the beaches of Santos Bay (Balthazar-Silva et al., 2020) and conforms to the requirements of “rapid repeated surveys of accumulation” outlined by GESAMP (2019). Separation involved washing the mix of sediment and plastic particles through a 0.1 mm sieve, packaging all retained material on-site and transportation to the laboratory for classification and counting. Sampling was repeated over three consecutive days following the high tide of spring (full moon) for each month to provide a seasonal comparison of daily pellet inputs (no. m2 ⋅ d–1). The relationship between observed inputs of pellets, PTM outputs, rainfall and beach position were investigated using Generalized Additive Models (GAMs), fit with the “mgcv” package (Wood, 2006) in the R statistical software environment (R Development Core Team, 2011). Models employed a Poisson distribution with significance assessed using the test criterion (α = 0.05) and significant combinations of predictor variables reported in terms of their level of explained deviation.
Results
Pellet Dispersal Footprint
The dispersal footprint of microplastic pellets was strongly contingent on release point and month (Table 1). The smallest dispersal area coincided with the middle estuarine release point (site B), which showed a mean footprint of only 3.6 km2 and exhibited little change across the year. The upper estuary release point (site A) showed a larger mean dispersal of 8.1 km2 and comparatively greater seasonal variation. Nevertheless, the majority of microplastic particles released from these two estuarine points concentrated close to their point of origin, rapidly becoming static (dry cell). In contrast, river mouth, inshore and offshore release points showed significantly greater dispersal (Table 1). Pellets released from the estuary mouth (point C) exhibited broad dispersal across the year (mean 34 km2) but showed marked seasonal variation (ranging from 24 km2 in December to 41 km2 in July). The inshore and offshore release points produced the greatest and most consistent dispersal areas (39 and 33 km2, respectively), with inshore releases from the beach exhibiting comparatively greater seasonal range (ranging from 39 km2 in November to 61 km2 in June) when compared to the offshore release point (ranging from 33 km2 in November to 41 km2 in October).
The effect of season on pellet dispersal is clearly illustrated by comparing the footprints of points A, C and D, for the three most characteristic periods of the year (i.e., November, March and July; Figure 2). Again, releases from the upper estuary (site A) during November did not appear to lead to major downstream transport when compared to March, or the more dynamic period of winter storms. Releases from the river mouth (site C) showed large seasonal variation and a pattern likely to lead to significant inputs of stranded pellets to the ocean beaches of Santos Bay. Likewise, simulated releases from inside the bay itself (site D), show that this could also be a major contributor of accumulated debris, especially at the western end, where beach morphology (typically wider and more gently sloping) and inputs from the stormwater canals can lead to more pronounced accumulation (see also Turra et al., 2014).
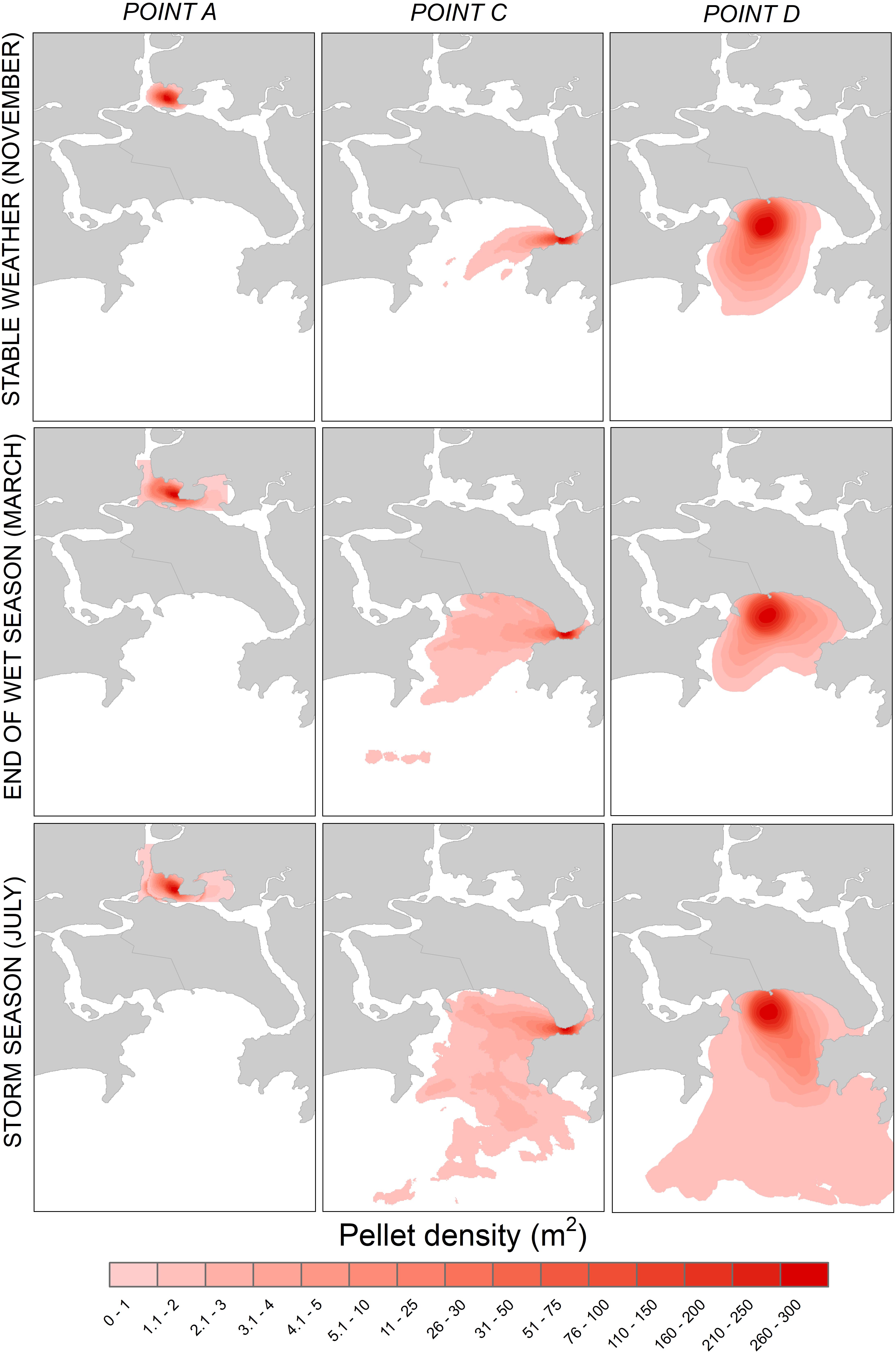
Figure 2. Dispersal simulations for plastic pellets during the stable weather conditions of November (top), the end of the rainfall period (March, middle) and the period of peak sea storm activity during June (bottom) considering 5 different release points within the SES and coastal waters of Santos Bay. Polygons show the area of dispersal, with the color ramp indicating the number of accumulated particles predicted using a “density plot” function that calculates a magnitude-per-unit area (m2) from points using a kernel function to fit a smoothly tapered surface to each point.
The dispersal footprint of pellets also highlighted interesting correlations with seasonal rainfall across a typical year (Supplementary Figure 1). Overall, the greatest period of rain occurred between December and March, the period May through to July was comparatively dry, and the period from August through to November was intermediate. The simulated dispersal of pellets from each release point differed considerably with monthly average rainfall. Site A showed a strong positive relationship with rainfall (with the greatest dispersal coinciding with the highest rainfall records over the first 4 months of the year); while inshore site D showed a negative relationship (high dispersal during lower rainfall during the winter months due to frontal systems from the south). Interestingly, the scale of dispersal from the river mouth (site C) did not present either a significant positive or negative relationship with rainfall.
Microplastic Litter Accumulation on the Beaches of Santos Bay
Pellet inputs rates to Santos beach varied with season and distance along the beach (zonation). Inputs during November (mean: 2 pellets m2) were substantially lower than July (14 m2), which was lower than March (51 m2); and for all periods there was a pronounced decrease with increasing distance westwards from the Santos river mouth (showing an average decline of 13 pellets m2 for every kilometer). The output of the Generalized Additive Model highlights the value of Lagrangian predictions, but also the effect of rainfall and beach zone (Table 2). Overall, the hydrodynamic dispersal model performed well in predicting the observed pellet inputs to Santos Beach (explaining 46% of the deviation), however, the inclusion of total rainfall and beach zonation (i.e., distance from river mouth) improved the predictive power substantially (93%) leading to a relationship between observed and predicted inputs that did not differ greatly from a slope of 1 (Figure 3).
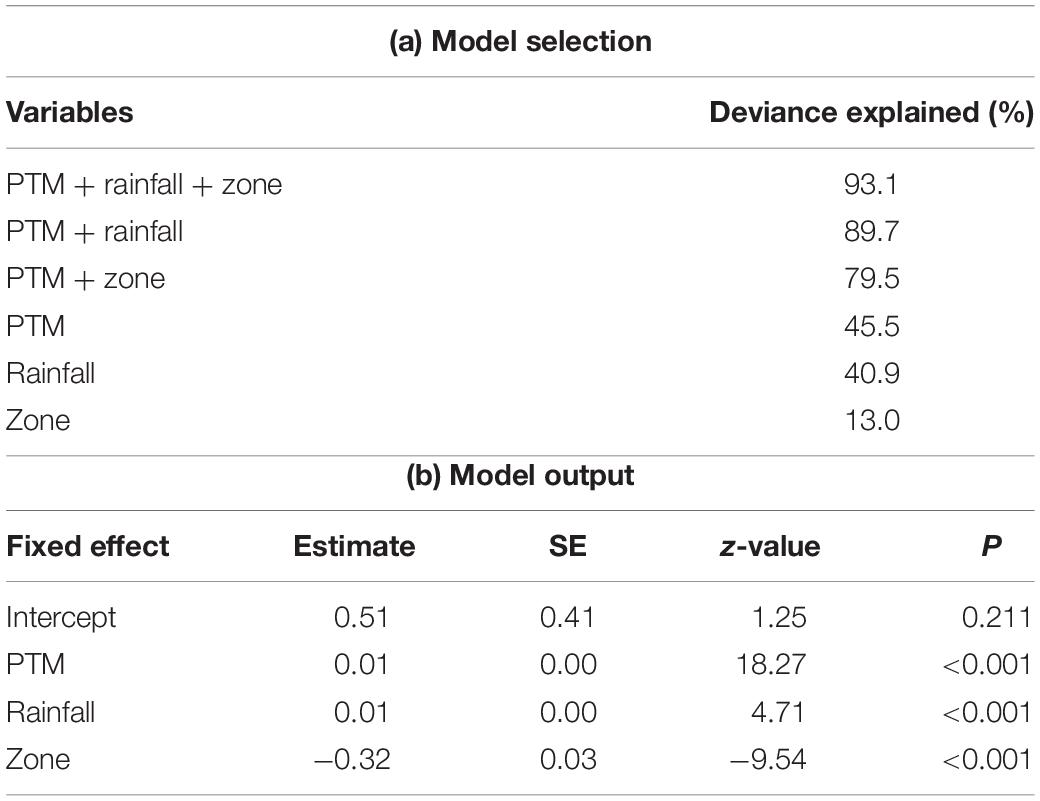
Table 2. Model selection based on explained deviance related to the inclusion of different predictors: Particle Tracking Model (PTM) outputs, rainfall and beach longshore zonation to explain rates of observed input of microplastic pellets to the open coastal beaches of Santos Bay.
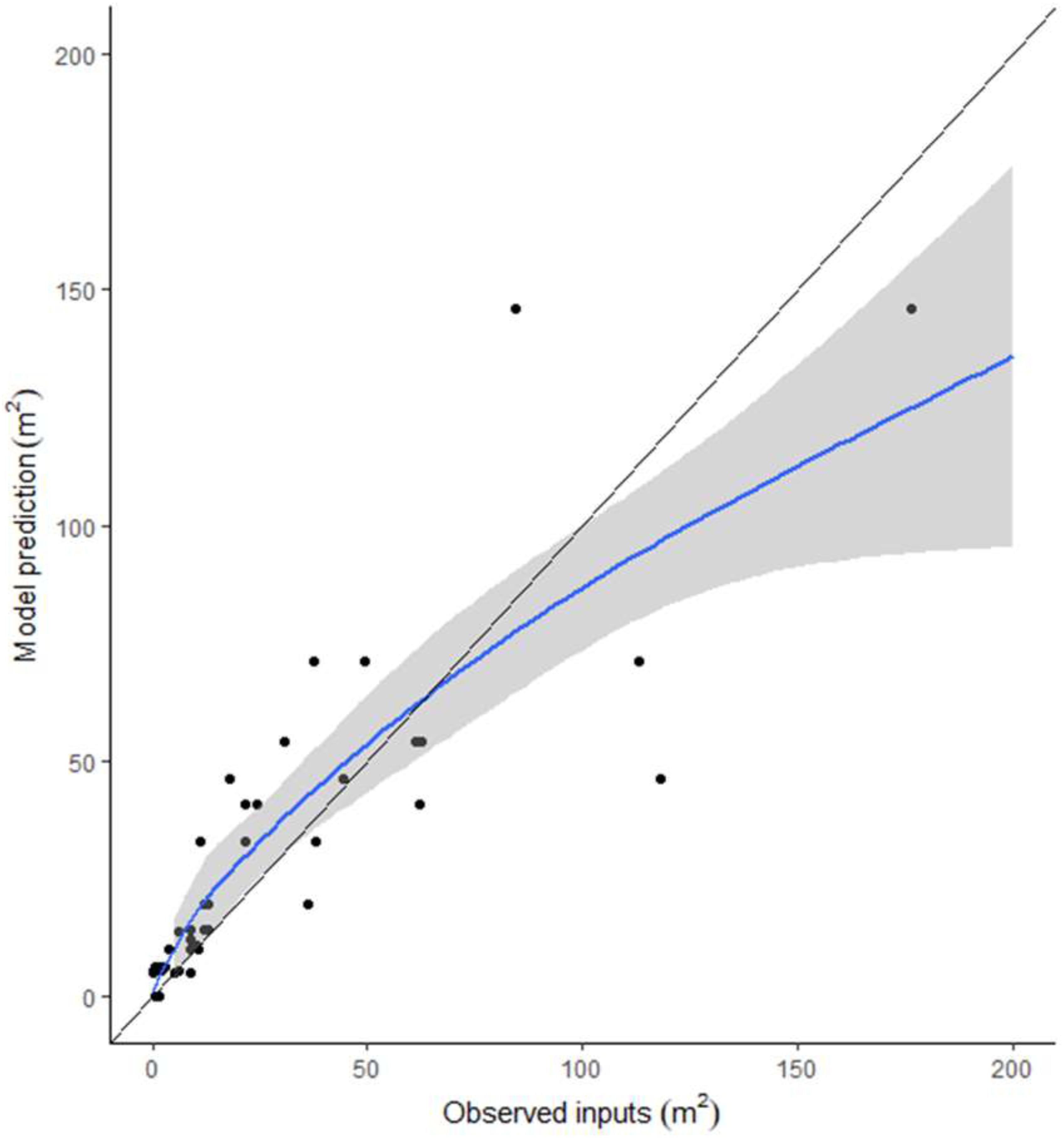
Figure 3. Relationship between observed pellet inputs to the beaches of Santos Bay (particles m2⋅d– 1) and predicted pellet inputs from the best performing model that incorporated the factors; PTM, rainfall and beach zone. Shading is the smooth term of the fitted model and the dashed line represents a 1:1 ratio between observed and predicted values.
Discussion
The goal of this study was to improve predictions of the dispersal and accumulation of microplastic particles within the estuarine and coastal waters of the Santos region (south-east Brazil). This information is crucial for regional policy development, given that this stretch of the Brazilian coast continues to be profoundly impacted by microplastic pollution (Turra et al., 2014) and there is a broad desire to identify and control further inputs (Izar et al., 2019). Simulations of the dispersal footprint (in km2) from different release points within the SES, suggest that microplastics disperse more broadly from river mouth and nearshore points than they do within the estuary. By combining PTM predictions with data that describe fluvial processes (governed by rainfall) and beach zonation (reflecting morphology and the influence of stormwater canals), it was possible to achieve a more accurate account of observed daily rates of pellet accumulation. This improvement in predictive power, suggests that integrated models might help to reconcile the interplay between raw material inputs from known point sources and the reintroduction of particles from diffuse sources through fluvial forcing and thereby guide management and mitigation policy relating to microplastic debris within linked estuarine and coastal systems (Ballent et al., 2013; Critchell et al., 2015).
Pellet Dispersal Footprint
The dispersal footprints generated for each release point (upper and middle estuary, mouth, inshore and offshore) reflect the likelihood that pellets released from these zones will be deposited along the beaches and coastal environments of Santos Bay. Pellets released from the upper and middle reaches of the SES (points A and B) appear more likely to interact with the edge of the predicted debris field (i.e., the river bank or shoreline) and are therefore likely to become beached or retained by vegetation, sediments or coastal infrastructure. From a modeling perspective, this means that they are subsequently excluded from further consideration, even though they might be re-introduced into the aquatic environment through rainfall events, flooding or extreme events that release them from the terrestrial environment, back into the debris field. Indeed, the retention and exportation of plastic debris from mangroves has been observed throughout the world, including the northeast and southern coasts of Brazil (Do Sul et al., 2014; Gorman and Turra, 2016).
Release points located at the river mouth, inshore (beach) and offshore areas of Santos Bay, showed significantly greater dispersal footprints across an entire year (ranging from 34 to 41 km2). Pellets released near the river mouth (point C) flowed out into the bay during all seasons, but there was a noticeable peak in March (coinciding with highest seasonal rainfall) that led to greater diffusion into coastal waters when compared to November and July. Although arguably less important than terrestrial inputs of microplastics, the release of particles from ships has been recognized as a pervasive source of marine litter in some regions (Derraik, 2002). This is true of Santos Bay, where continued illegal dumping and ship maintenance (e.g., blasting of tanks; Manzano, 2009) of anchored vessels is believed to contribute pellets to the regions beaches, especially during winter, when these buoyant particles are washed up in high concentrations (Magini et al., 2007).
Our models suggest that pellets released from inshore (beach) and offshore zones (points D and E) disperse broadly during winter months and make a significant contribution to the microplastic debris accumulating on the beaches of Santos Bay (see; Turra et al., 2014; Balthazar-Silva et al., 2020). These patterns of accumulation however, are unlikely to be uniform and will be governed by sometimes strong tidal currents (up to 100 cm s–1) that include the westward drift vortex and the Itararé vortex (Maggi et al., 2012) which coincide with the passage of winter storm fronts. This type of alongshore variability in plastic debris accumulation in other parts of the world has been attributed to a combination of complex coastline geomorphology, oceanic forcing and resuspension of floating debris by waves (Critchell et al., 2015). It is equally likely that a significant proportion of the pellets released from offshore sources are exported to neighboring beaches (Turra et al., 2014; Moreira et al., 2016) and even as far afield as other states hundreds of kilometers away (e.g., Paraná; Gorman et al., 2019).
Overall, the data presented suggests that the factors governing dispersal and accumulation of microplastics in the Santos region may differ spatio-temporally between estuarine and nearshore zones. While the origin of plastic debris in estuaries may be easier to delineate spatially (i.e., they tend to “beach” close to their origin), the temporal contribution of these materials may be harder to resolve because they may become alternatively “beached” and then “reintroduced” as a result of fluvial processes. In contrast, nearshore and offshore sources may be easier to delineate if there is detailed knowledge of the timing and intensity of oceanographic circulation and winter storms but may show weaker links to rainfall and fluvial possesses. Contrasting the relative importance of these processes in estuaries vs. open coasts is an intriguing topic and one that may help reconcile the role of local rivers vs. ocean processes as transport pathways for plastic debris in coastal and marine environments (Zhao et al., 2019).
While dispersal models accurately predict the behavior of a particle in the water column under a given set of internal conditions (i.e., wind, currents, density, etc.), they may be improved substantially by including external drivers such as rainfall and beach characteristics (morphology and terrestrial inputs). The fact that a high proportion of simulated particles released from the upper and middle zones of the estuary become rapidly beached and were considered no longer active, highlights a potential limitation to the modeled behavior (similar to model limitations at the scale of oceans, see; Iwasaki et al., 2017). Accounting for the re-introduction of these materials during rainfall events using additive modeling approaches (e.g., GAMs), might help to reconcile and improve our predictive capacity. The GAM outputs highlight marked but contrasting relationships between simulated dispersal and total monthly rainfall for different release points. This is likely to reflect flood events that reintroduce trapped particles by dislodging riparian vegetation and eroding sediments. In this way, by including rainfall data into models, it was possible to increase current understanding of the temporal cycles that capture pulse-driven inputs of debris to coastal and adjacent offshore areas. While, there have been attempts to quantify the magnitude of plastic items exported from coastal wetlands during increased periods of runoff (Ryan et al., 1990; Coe and Rogers, 1997; Do Sul et al., 2014) this field of research remains a challenge because the response is likely to be decoupled in space and time and moreover confounded by shoreline complexity (i.e., the density of shoreline vegetation and shoreline topography).
Coupling PTM’s With Fluvial Processes
Although several studies have validated the output of particle tracking models using survey data over large spatial scales (e.g., the entire Sea of Japan; Iwasaki et al., 2017), the present study is one of few to compare model predictions with observed particle inputs at the scale of an individual beach. Results show that pellet inputs vary both seasonally (March having particle counts 19 × greater than November) and that there is consistent variation with distance from the river mouth (density declining by 7% per km) that reflects beach morphology and the level of terrestrial inputs. While this finding is not new for the region (see previous studies by‘: Turra et al., 2014; Fisner et al., 2017) the present data points to the value of combining PTM outputs with fluvial processes using a stepwise correlative approach. Indeed, the Generalized Additive Model emphasizes the need to consider such factors to accurately and consistently predict microplastic accumulation on ocean beaches. The marked effect of rainfall for the study region is to be expected, as heavy falls can occur over relatively short periods of time (Gorman et al., 2017) washing material from roads, parks and drainage networks (canals) into the marine environment. Indeed, the effect of fluvial forcing on the dispersal of microplastics from rivers is highly dependent on season, proximity to sources and sedimentary dynamics (e.g., China; Wan et al., 2018; Italy; Guerranti et al., 2017; Korea; Seo and Park, 2020 and the Atlantic coast of France; Frere et al., 2017). A previous study by Manzano (2009), suggested greater inputs of plastic pellets to Santos beaches during winter (average of 102 pellets/m2 and maximum of 377 pellets/m2). This matches similar reports across the globe, whereby the highest accumulation of plastic waste on beaches typically occurs during winter months and after storm events (Shiber, 1987; Moore et al., 2002). Indeed, recent data from Brazil, demonstrates that short-term episodic events can be just as important as seasonal variation in terrestrial runoff as a driver of debris accumulation in subtropical systems (i.e., a 20-fold increase in pellet inputs per m2, before and after rainfall events; Balthazar-Silva et al., 2020).
In conclusion, this study highlights the value of hydrodynamic models for mapping the spatial footprint of microplastic debris in complex coastal systems with strong seasonal climate. Further, by coupling the predictions from dispersal models with seasonal trends in rainfall and beach zonation, it is possible to explain variability in the input of pellets to open coastal beaches at an even finer spatial resolution. This will not only help to delineate the sources of microplastic pollution in complex marine and coastal systems (permitting effective local intervention and mitigation; Vegter et al., 2014), but can improve the ability of models to explain and replicate real-time spatial patterns. This information will not only join with efforts around the world to better understand the growing problem of plastic pollution across a range of spatial scales (Maximenko et al., 2019) but will help to reconcile processes occurring within dynamic estuarine systems where the interaction of processes occurring on land and water make predicting the dispersal and accumulation of microplastics a challenge.
Data Availability Statement
The raw data supporting the conclusions of this article will be made available by the authors, without undue reservation.
Author Contributions
DG: conceptualization, methodology, formal analysis, writing – original draft, project administration, and funding acquisition. AG: methodology, software, formal analysis, and writing – original draft. AT: conceptualization, writing – review and editing, project administration, and funding acquisition. AM: conceptualization and investigation. DB-S: investigation, validation, and writing – review and editing. NO: investigation and writing – review and editing. JH: conceptualization, resources, methodology, software, formal analysis, and supervision. All authors contributed to the article and approved the submitted version.
Conflict of Interest
The authors declare that the research was conducted in the absence of any commercial or financial relationships that could be construed as a potential conflict of interest.
Funding
This work was funded by the São Paulo Research Foundation (FAPESP) through Young Researcher scholarships to DG (2016/26064-3 and 2018/06162-6), a research grant to AT (2007/51924-7), and by the National Council for Scientific and Technological Research (CNPq) to AT (301240/2006-0; 484475/2011-8; 309697/2015-8; 310553/2019-9). Support for the development of the article in the special issue Microplastics in the Marine Environment: Sources, Distribution, Biological Effects and Socio-Economic Impacts was provided by the CSIRO Oceans and Atmosphere – Coasts Program.
Supplementary Material
The Supplementary Material for this article can be found online at: https://www.frontiersin.org/articles/10.3389/fenvs.2020.559405/full#supplementary-material
Supplementary Figure 1 | Relationship between monthly rainfall and modeled dispersal area (km2) of microplastic pellets from three different release points within the estuary and open coastal waters of the Santos region. Correlations are Pearson’s correlation (r) and two-tailed probability (P).
Footnotes
- ^ http://www.ngdc.noaaGov/mgg/GEBCO/
- ^ http://mapas.ibge.gov.br/bases-e-referenciais/basescartograficas/
References
Ballent, A., Pando, S., Purser, A., Juliano, M. F., and Thomsen, L. (2013). Modelled transport of benthic marine microplastic pollution in the Nazare Canyon. Biogeosciences 10, 7957–7970. doi: 10.5194/bg-10-7957-2013
Balthazar-Silva, D. (2016). Spatial Distribution, Input and Dispersion of Plastic Pellets in Coastal Zones. Ph. D. thesis, University of São Paulo, São Paulo.
Balthazar-Silva, D., Turra, A., Moreira, F. T., Camargo, R. M., Oliveira, A. L., Barbosa, L., et al. (2020). Rainfall and tidal cycle regulate seasonal inputs of microplastic pellets to sandy beaches. Front. Environ. Sci. 8:123. doi: 10.3389/fmars.2019.00123
Barnes, D. K. A., Galgani, F., Thompson, R. C., and Barlaz, M. (2009). Accumulation and fragmentation of plastic debris in global environments. Philos. Trans. R. Soc. B Biol. Sci. 364, 1985–1998. doi: 10.1098/rstb.2008.0205
Blumberg, A., and Mellor, G. (1987). “A description of a three-dimensional coastal ocean circulation model,” in Three-Dimensional Coastal Ocean Models, Vol. 4, ed. N. Heaps (Washington, DC: American Geophysical Union), 1–16. doi: 10.1029/co004p0001
Braunschweig, F., Martins, F., Chambel, P., and Neves, R. (2003). A methodology to estimate renewal time scales in estuaries: the Tagus Estuary case. Ocean Dyn. 53, 137–145. doi: 10.1007/s10236-003-0040-0
Browne, M. A., Dissanayake, A., Galloway, T. S., Lowe, D. M., and Thompson, R. C. (2008). Ingested microscopic plastic translocates to the circulatory system of the mussel, Mytilus edulis (L.). Environ. Sci. Technol. 42, 5026–5031. doi: 10.1021/es800249a
Cabral, F. (2014). Microplásticos no Ambiente Marinho: Mapeamento de Fontes e Identificação de Mecanismos de Gestão Para Minimização da peRda de Pellets Plásticos SP, Brasil. M.Sc. thesis, University of São Paulo, São Paulo.
CETEC (2000). Centro Tecnológico da Fundação Paulista de Tecnologia e Educação. Minuta preliminar do relatório de Situação dos Recursos Hídricos da UGRHI7”, in: Comitê da Bacia Hidrográfica da Baixada Santista. Relatório Zero. Available online at: http://www.sigrh.sp.gov.br/cgibin/sigrh_home_colegiado.exe?TEMA=RELATOR IO&COLEGIADO=CRH/CBH-BS&lwgactw=267731 (accessed February 4, 2020).
Coe, J., and Rogers, D. (1997). Marine Debris: Sources, Impacts, and Solutions. New York, NY: Springer-Verlag. doi: 10.1007/978-1-4613-8486-1
Critchell, K., Grech, A., Schlaefer, J., Andutta, F. P., Lambrechts, J., Wolanski, E., et al. (2015). Modelling the fate of marine debris along a complex shoreline: lessons from the great barrier reef. Estuar. Coast. Shelf Sci. 167, 414–426. doi: 10.1016/j.ecss.2015.10.018
DAEE (1999). Banco de dados Pluviográficos do Estado de São Paulo Fundação do Centro Tecnológico de Hidráulica, USP - CD-ROOM. São Paulo: Departamento de Águas e Energia Elétrica.
De Mesquita, A. R., and Harari, J. (2003). On the harmonic constants of tides and tidal currents of the South-eastern brazilian shelf. Continent. Shelf Res. 23, 1227–1237. doi: 10.1016/s0278-4343(03)00107-9
De Wit, W., and Bigaud, N. (2019). No Plastic in Nature: Assessing Plastic Ingestion From Nature To People. Gland: WWF.
Debrot, A. O., Meesters, H. W. G., Bron, P. S., and De Leon, R. (2013). Marine debris in mangroves and on the seabed: largely-neglected litter problems. Mar. Pollut. Bull. 72:1. doi: 10.1016/j.marpolbul.2013.03.023
Derraik, J. G. B. (2002). The pollution of the marine environment by plastic debris: a review. Mar. Pollut. Bull. 44, 842–852. doi: 10.1016/s0025-326x(02)00220-5
Do Sul, J. A. I., Costa, M. F., Silva-Cavalcanti, J. S., and Araujo, M. C. B. (2014). Plastic debris retention and exportation by a mangrove forest patch. Mar. Pollut. Bull. 78, 252–257. doi: 10.1016/j.marpolbul.2013.11.011
EPA (1990). Methods to Manage and Control Plastic Wastes. Report to the Congress/530-SW-89-051. Washington, DC: Environmental Protection Agency.
Fisner, M., Majer, A. P., Balthazar-Silva, D., Gorman, D., and Turra, A. (2017). Quantifying plastic pellets on sandy beaches: the conundrum of large sample variation and spatial heterogeneity. Environ. Sci. Pollut. Res. 24, 13732–13740. doi: 10.1007/s11356-017-8883-y
Fossi, M. C., Panti, C., Guerranti, C., Coppola, D., Giannetti, M., Marsili, L., et al. (2012). Are baleen whales exposed to the threat of microplastics? A case study of the Mediterranean fin whale (Balaenoptera physalus). Mar. Pollut. Bull. 64, 2374–2379. doi: 10.1016/j.marpolbul.2012.08.013
Frere, L., Paul-Pont, I., Rinnert, E., Petton, S., Jaffre, J., Bihannic, I., et al. (2017). Influence of environmental and anthropogenic factors on the composition, concentration and spatial distribution of microplastics: a case study of the Bay of Brest (Brittany, France). Environ. Pollut. 225, 211–222. doi: 10.1016/j.envpol.2017.03.023
Frias, J., Otero, V., and Sobral, P. (2014). Evidence of microplastics in samples of zooplankton from Portuguese coastal waters. Mar. Environ. Res. 95, 89–95. doi: 10.1016/j.marenvres.2014.01.001
GESAMP (2015). Sources, Fate and Effects of Microplastics in the Marine Environment: a Global Assessment 90. Report Stud. GESAMP No. 90, 96. London: GESAMP.
GESAMP (2019). Guidelines or the Monitoring and Assessment of Plastic Litter and Microplastics in the Ocean. Report Stud. GESAMP No. 99, 130. London: GESAMP.
GESAMP (2020). Proceedings of the GESAMP International Workshop on Assessing the risks Associated with Plastics and Microplastics in the Marine Environment (Kershaw, P.J., Carney Almroth, B., Villarrubia-Gómez, P., Koelmans, A.A., and Gouin, T., eds) (IMO/FAO/UNESCO-IOC/UNIDO/WMO/IAEA/UN/UNEP/UNDP/ISA Joint Group of Experts on the Scientific Aspects of Marine Environmental Protection). Reports to GESAMP No. 103, 68. Available online at: http://www.gesamp.org/site/assets/files/2136/rs103e-1.pdf (accessed March 4, 2020).
Ghisolfi, R. D., and Garcia, C. A. E. (1996). “Efeitos do processo difusivo na estimativa de correntes superficiais pelo método MCC,” in Proceedings of the Anais VIII Simpósio Brasileiro de Sensoriamento Remoto, Salvador.
Gorman, D. (2018). “Historical losses of mangrove systems in south America from both human-induced and natural impacts,” in Threats to Mangrove Forests: Hazards, Vulnerability, and Management, ed. C. Makowski (Cham: Springer). doi: 10.1007/978-3-319-73016-5_8
Gorman, D., Moreira, F. T., Turra, A., Fontenelle, F. R., Combi, T., Bicego, M. C., et al. (2019). Organic contamination of beached plastic pellets in the South Atlantic: risk assessments can benefit by considering spatial gradients. Chemosphere 223, 608–615. doi: 10.1016/j.chemosphere.2019.02.094
Gorman, D., and Turra, A. (2016). The role of mangrove revegetation as a means of restoring macrofaunal community structure along subtropical coasts. Sci. Total Environ. 566–567, 223–229. doi: 10.1016/j.scitotenv.2016.05.089
Gorman, D., Turra, A., Connolly, R. M., Olds, A. D., and Schlacher, T. A. (2017). Monitoring nitrogen pollution in seasonally-pulsed coastal waters requires judicious choice of indicator species. Mar. Pollut. Bull. 122, 149–155. doi: 10.1016/j.marpolbul.2017.06.042
Guerranti, C., Cannas, S., Scopetani, C., Fastelli, P., Cincinelli, A., and Renzi, M. (2017). Plastic litter in aquatic environments of Maremma Regional Park (Tyrrhenian Sea, Italy): contribution by the Ombrone river and levels in marine sediments. Mar. Pollut. Bull. 117, 366–370. doi: 10.1016/j.marpolbul.2017.02.021
Hall, N. M., Berry, K. L. E., Rintoul, L., and Hoogenboom, M. O. (2015). Microplastic ingestion by scleractinian corals. Mar. Biol. 162, 725–732. doi: 10.1007/s00227-015-2619-7
Harari, J., Camargo, R., and Cacciari, P. (2000). Resultados da modelagem numérica hidrodinâmica em simulações tridimensionais das correntes de maré na Baixada Santista. Rev. Bras. Rec. Hidric. 5, 71–87. doi: 10.21168/rbrh.v5n2.p71-87
Harari, J., Camargo, R., Franca, C. A. S., Mesquita, A. R., and Picarelli, S. S. (2006). Numerical Modeling of the hydrodynamics in the coastal area of São Paulo State Brazil. J. Coast. Res. 39, 1560–1563.
Harari, J., França, C., and Camargo, R. (2008). “Climatology and hydrography of the Santos Estuary,” in Perspectives on Integrated Coastal Zone Management in South America, eds R. Neves, J. Baretta, and M. Mateus (Lisboa: IST Press), 147–160.
Harari, J., and Gordon, M. (2001). Simulações numéricas da dispersão de substâncias no porto e baía de santos, sob a Ação de Marés. Rev. Bras. Rec. Hídric. 6, 115–131. doi: 10.21168/rbrh.v6n4.p115-131
Hardesty, B. D., Harari, J., Isobe, A., Lebreton, L., Maximenko, N., Potemra, J., et al. (2017). Using numerical model simulations to improve the understanding of micro-plastic distribution and pathways in the marine environment. Front. Mar. Sci. 4:30. doi: 10.3389/fmars.2019.00030
Iwasaki, S., Isobe, A., Kako, S., Uchida, K., and Tokai, T. (2017). Fate of microplastics and mesoplastics carried by surface currents and wind waves: a numerical model approach in the Sea of Japan. Mar. Pollut. Bull. 121, 85–96. doi: 10.1016/j.marpolbul.2017.05.057
Izar, G. M., Morais, L. G., Pereira, C. D. S., Cesar, A., Abessa, D. M. S., and Christofoletti, R. A. (2019). Quantitative analysis of pellets on beaches of the São Paulo coast and associated non-ingested ecotoxicological effects on marine organisms. Reg. Stud. Mar. Sci. 29:100705. doi: 10.1016/j.rsma.2019.100705
Kooi, M., Reisser, J., Slat, B., Ferrari, F. F., Schmid, M. S., Cunsolo, S., et al. (2016). The effect of particle properties on the depth profile of buoyant plastics in the ocean. Sci. Rep. 6:33882. doi: 10.1038/srep33882
Krelling, A. P., Souza, M. M., Williams, A. T., and Turra, A. (2017). Transboundary movement of marine litter in an estuarine gradient: evaluating sources and sinks using hydrodynamic modelling, and ground truthing estimates. Mar. Pollut. Bull. 119, 48–63. doi: 10.1016/j.marpolbul.2017.03.034
Lamparelli, C. C. (1998). Mapeamento dos Ecossistemas Costeiros do Estado de São Paulo. São Paulo: Companhia de Tecnologia de Saneamento Ambiental.
Lebreton, L. C. M., Greer, S. D., and Borrero, J. C. (2012). Numerical modelling of floating debris in the world’s oceans. Mar. Pollut. Bull. 64, 653–661. doi: 10.1016/j.marpolbul.2011.10.027
Lebreton, L. C. M., Van Der Zwet, J., Damsteeg, J. W., Slat, B., Andrady, A., and Reisser, J. (2017). River plastic emissions to the world’s oceans. Nat. Commun. 8:15611. doi: 10.1038/ncomms15611
Leprovost, C., Genco, M. L., Lyard, F., Vincent, P., and Canceil, P. (1994). Spectroscopy of the world ocean tides from a finite-element hydrodynamic model. J. Geophys. Res. Oceans 99, 24777–24797. doi: 10.1029/94JC01381
Levitus, S., and Boyer, T. (1994). World Ocean Atlas 1994 Volume 4: Temperature. Washington, DC: U.S. Department of Commerce.
Lusher, A. L., Mchugh, M., and Thompson, R. C. (2013). Occurrence of microplastics in the gastrointestinal tract of pelagic and demersal fish from the English Channel. Mar. Pollut. Bull. 67, 94–99. doi: 10.1016/j.marpolbul.2012.11.028
Maggi, E., Bulleri, F., Bertocci, I., and Benedetti-Cecchi, L. (2012). Competitive ability of macroalgal canopies overwhelms the effects of variable regimes of disturbance. Mar. Ecol. Prog. Ser. 465, 99–109. doi: 10.3354/meps09903
Magini, C., Harari, J., and Abessa, D. (2007). Circulação recente de sedimentos costeiros nas praias de Santos durante eventos de tempestades: dados para a gestão de impactos físicos costeiros. Geociências 26, 349–355.
Manzano, A. B. (2009). Distribuição, Taxa de Entrada, Composição Química e Identificação de Fontes de Grânulos Plásticos na Enseada de Santos, SP, Brasil. M.Sc. thesis, São Paulo University, São Paulo.
Martinez, E., Maamaatuaiahutapu, K., and Taillandier, V. (2009). Floating marine debris surface drift: convergence and accumulation toward the South Pacific subtropical gyre. Mar. Pollut. Bull. 58, 1347–1355. doi: 10.1016/j.marpolbul.2009.04.022
Mateus, M., Riflet, G., Chambel, P., Fernandes, L., Fernandes, R., Juliano, M., et al. (2012). An operational model for the West Iberian coast: products and services. Ocean Sci. 8, 713–732. doi: 10.5194/os-8-713-2012
Maximenko, N., Corradi, P., Law, K. L., Van Sebille, E., Garaba, S. P., Lampitt, R. S., et al. (2019). Toward the integrated marine debris observing system. Front. Mar. Sci. 6:447. doi: 10.3389/fmars.2019.00447
Maximenko, N., Hafner, J., and Niiler, P. (2012). Pathways of marine debris derived from trajectories of Lagrangian drifters. Mar. Pollut. Bull. 65, 51–62. doi: 10.1016/j.marpolbul.2011.04.016
Mellor, G. (1998). A Three-Dimensional, Primitive Equation, Numerical Ocean Model User’ s Guide. London: Princeton University.
Moore, C. J., Moore, S. L., Weisberg, S. B., Lattin, G. L., and Zellers, A. F. (2002). A comparison of neustonic plastic and zooplankton abundance in southern California’s coastal waters. Mar. Pollut. Bull. 44, 1035–1038. doi: 10.1016/s0025-326x(02)00150-9
Moreira, F. T., Balthazar-Silva, D., Barbosa, L., and Turra, A. (2016). Revealing accumulation zones of plastic pellets in sandy beaches. Environ. Pollut. 218, 313–321. doi: 10.1016/j.envpol.2016.07.006
Moser, G., Gianesella, S., Alba, J., Bérgamo, A., Corrêa, F., Miranda, L., et al. (2005). Instantaneous transport of salt, nutrients, suspended matter and clorophyll a in the tropical estuarine system of Santos. Braz. J. Oceanogr. 53, 115–127. doi: 10.1590/s1679-87592005000200003
Ockelford, A., Cundy, A., and Ebdon, J. E. (2020). Storm response of fluvial sedimentary microplastics. Sci. Rep. 10:1865. doi: 10.1038/s41598-020-58765-2
Ogata, Y., Takada, H., Mizukawa, K., Hirai, H., Iwasa, S., Endo, S., et al. (2009). International Pellet Watch: global monitoring of persistent organic pollutants (POPs) in coastal Waters. 1. Initial phase data on PCBs, DDTs, and HCHs. Mar. Pollut. Bull. 58, 1437–1446. doi: 10.1016/j.marpolbul.2009.06.014
Onink, V., Wichmann, D., Delandmeter, P., and Van Sebille, E. (2019). The role of ekman currents, geostrophy, and stokes drift in the accumulation of floating microplastic. J. Geophys. Res. Oceans 124, 1474–1490. doi: 10.1029/2018jc014547
PlasticsEurope (2019). Plastics - the Facts 2019An Analysis of European Plasticsproduction, Demand and Waste Data. Düsseldorf: PlasticsEurope.
R Development Core Team (2011). R: A Language and Environment for Statistical Computing, 2.11.1 ed. (Vienna, Austria: R Foundation for Statistical Computing).
Reisser, J., Shaw, J., Wilcox, C., Hardesty, B. D., Proietti, M., Thums, M., et al. (2013). Marine plastic pollution in waters around australia: characteristics, concentrations, and pathways. PLoS One 8:e80466. doi: 10.1371/journal.pone.0080466
Rubinstein, R., and Kroese, D. (2016). Simulation and the Monte Carlo Method. New York: John Wiley & Sons. doi: 10.1002/9781118631980
Ryan, C. G., Cousens, D. R., Sie, S. H., Griffin, W. L., and Suter, G. F. (1990). Quantitativepixe microanalysis of geological material using the CSIRO proton microprobe. Nuclear Instrum. Methods Phys. Res. B 47, 55–71. doi: 10.1016/0168-583x(90)90047-x
Seo, S., and Park, Y. G. (2020). Destination of floating plastic debris released from ten major rivers around the Korean Peninsula. Environ. Intern. 138:105655. doi: 10.1016/j.envint.2020.105655
Shiber, J. G. (1987). Plastic pellets and tar on Spain Mediterranean beaches. Mar. Pollut. Bull. 18, 84–86. doi: 10.1016/0025-326x(87)90573-x
Thiel, M., Luna-Jorquera, G., Alvarez-Varas, R., Gallardo, C., Hinojosa, I. A., Luna, N., et al. (2018). Impacts of marine plastic pollution from continental coasts to subtropical gyres-fish, seabirds, and other vertebrates in the SE pacific. Front. Mar. Sci. 5:238. doi: 10.3389/fmars.2019.00238
Turra, A., Manzano, A. B., Dias, R. J. S., Mahiques, M. M., Barbosa, L., Balthazar-Silva, D., et al. (2014). Three-dimensional distribution of plastic pellets in sandy beaches: shifting paradigms. Sci. Rep. 4:4435. doi: 10.1038/srep04435
U.S. Environmental Protection Agency (1992). Statistical Methods for Evaluating the Attainment of Cleanup Standards, Volume 3: Reference-Based Standards for Soils and Solid Media. Washington, DC: Office of Policy Planning and Evaluation.
Van Sebille, E., Wilcox, C., Lebreton, L., Maximenko, N., Hardesty, B. D., Van Franeker, J. A., et al. (2015). A global inventory of small floating plastic debris. Environ. Res. Lett. 10:11. doi: 10.1088/1748-9326/10/12/124006
Vegter, A. C., Barletta, M., Beck, C., Borrero, J., Burton, H., Campbell, M. L., et al. (2014). Global research priorities to mitigate plastic pollution impacts on marine wildlife. Endang. Spec. Res. 25, 225–247. doi: 10.3354/esr00623
Viehman, S., Pluym, J. L. V., and Schellinger, J. (2011). Characterization of marine debris in North Carolina salt marshes. Mar. Pollut. Bull. 62, 2771–2779. doi: 10.1016/j.marpolbul.2011.09.010
Wan, J., Wang, Y. G., Cheng, M. L., Engel, B. A., Zhang, W. S., and Peng, H. (2018). Assessment of debris inputs from land into the river in the Three Gorges Reservoir Area, China. Environ. Sci. Pollut. Res. 25, 5539–5549. doi: 10.1007/s11356-017-0881-6
Wood, S. N. (2006). Generalized Additive Models : An Introduction with R. Boca Raton, FL: Chapman & Hall. doi: 10.1201/9781420010404
Zhang, C. N., Wang, S. D., Sun, D., Pan, Z. K., Zhou, A. G., Xie, S. L., et al. (2020). Microplastic pollution in surface water from east coastal areas of Guangdong, South China and preliminary study on microplastics biomonitoring using two marine fish. Chemosphere 256:127202. doi: 10.1016/j.chemosphere.2020.127202
Zhang, D. D., Liu, X. D., Huang, W., Li, J. J., Wang, C. S., Zhang, D. S., et al. (2020). Microplastic pollution in deep-sea sediments and organisms of the Western Pacific Ocean. Environ. Pollut. 259:113948. doi: 10.1016/j.envpol.2020.113948
Keywords: microplastic, pellet, hydrodynamic dispersion model, fluvial processes, marine debris
Citation: Gorman D, Gutiérrez AR, Turra A, Manzano AB, Balthazar-Silva D, Oliveira NR and Harari J (2020) Predicting the Dispersal and Accumulation of Microplastic Pellets Within the Estuarine and Coastal Waters of South-Eastern Brazil Using Integrated Rainfall Data and Lagrangian Particle Tracking Models. Front. Environ. Sci. 8:559405. doi: 10.3389/fenvs.2020.559405
Received: 06 May 2020; Accepted: 07 September 2020;
Published: 15 October 2020.
Edited by:
João P. G. L. Frias, Galway-Mayo Institute of Technology, IrelandReviewed by:
Erik Van Sebille, Utrecht University, NetherlandsMei Xuefei, East China Normal University, China
Copyright © 2020 Gorman, Gutiérrez, Turra, Manzano, Balthazar-Silva, Oliveira and Harari. This is an open-access article distributed under the terms of the Creative Commons Attribution License (CC BY). The use, distribution or reproduction in other forums is permitted, provided the original author(s) and the copyright owner(s) are credited and that the original publication in this journal is cited, in accordance with accepted academic practice. No use, distribution or reproduction is permitted which does not comply with these terms.
*Correspondence: Daniel Gorman, d.gorman@csiro.au