Setting the scene: background and aim
Mastitis is an inflammation of the mammary gland, frequently caused by intramammary infections (IMI), and occasionally by mechanical or chemical trauma (through teat or udder trampling for instance), or when cleaning liquids are mistaken for teat disinfectants. Mastitis and IMI are different biological processes that require different diagnostic tools and treatments. Mastitis is characterised by physical and chemical changes in milk and, in moderate or severe cases, by pathological changes in the mammary gland or systemically. Clinical mastitis (CM) can be detected using human senses (vision, touch, and taste, although the latter is discouraged), whereas detection of subclinical mastitis (SCM) requires additional tools. With no one-to-one relationship between clinical severity and causative agent, the cause of mastitis can rarely be detected without further testing. Ideally, mastitis treatment is based on knowledge of severity, causative agent and prognosis so that anti-inflammatory, antimicrobial and supportive treatments can be selected for maximum efficacy and minimal side effects, which include potential selection for antimicrobial resistance (AMR). The contribution of antimicrobial use (AMU) to selection for AMR has become of concern due to actual or predicted adverse effects in human and veterinary health (O'Neill, Reference O'Neill2016). Both quantity and ‘quality’ (or type) of antimicrobials are important. The World Health Organization (WHO) has identified Highest Priority Critically Important Antimicrobials (HP-CIA) for human medicine (WHO, 2019), and suggested that HP-CIA ‘should not be used for treatment of food-producing animals with a clinically diagnosed infectious disease’ (WHO, 2017). Quinolones, macrolides and ketolides, polymixins and 3rd and 4th generation cephalosporins are all HP-CIA used for mastitis treatment and we can do better in this area (Turner et al., Reference Turner, Tisdall, Barrett, Wood, Dowsey and Reyher2018; Doehring and Sundrum, Reference Doehring and Sundrum2019).
Selective treatment is the practice of restricting AMU to cases most likely to benefit from treatment and may help to reduce AMU. The phrase ‘targeted treatment’ is also used and hints at a different underlying philosophy. ‘Selective treatment’ suggests blanket treatment as the default option, which is modified by selecting cows for treatment. Targeted treatment suggests no treatment to be the default option, with targeting of treatment to those cows that are most likely to benefit. Whilst selective dry cow treatment has been or become the norm in several countries (Vanhoudt et al., Reference Vanhoudt, van Hees-Huijps, van Knegsel, Sampimon, Vernooij, Nielen and van Werven2018), selective treatment of lactational mastitis is a more recent development (Lago and Godden, Reference Lago and Godden2018). Principles underpinning this approach include the notion that AMU must be reduced in livestock; the suggestion that HP-CIA should be avoided in veterinary medicine; the recognition that mild to moderate CM caused by gram-negative pathogens does not necessarily need antimicrobial treatment; evidence that narrow-spectrum antimicrobials are effective against most gram-positive mastitis; and the maxim that ‘lack of an indication is a contra-indication’, whereby absence of viable bacteria may be interpreted as lack of an indication. Each of these principles is subject to debate, to individual differences in weighing of available evidence, and to technical or societal opportunities and constraints, which explains the wide range of AMU practices on dairy farms. Central to targeted treatment of lactational CM is the ability to differentiate mild-to-moderate gram-positive mastitis from other forms of mastitis. Point-of-care (POC) testing can make this distinction. For the sake of this paper, we interpret ‘point-of-care’ as a tool to inform treatment decisions. Generally, this requires on-farm testing, although some commercial diagnostic laboratories or veterinary practices may offer a 24-h turn-around time (TAT) or service.
In this paper, we reflect on characteristics of POC tests with a focus on CM diagnostics that can inform treatment decisions. Specifically, we focus on how such tests succeed or fail in addressing professional and societal needs, and how future developments might change this.
What do we need?
We need to detect CM cases, which is usually done during milking, by people or by equipment (reviewed by Hogeveen et al., Reference Hogeveen, Kamphuis, Steeneveld and Mollenhorst2010). Where broad-spectrum antimicrobials are advocated for treatment of CM, one could argue that there is no need for further diagnostics. To reduce AMU, however, treatment would need to be targeted to a subset of CM cases based on severity and causative agent. In addition to reduction in AMU, refinement may be possible, e.g. by avoidance of HP-CIA antimicrobials, by use of narrow-spectrum antimicrobials, or possibly based on antimicrobial susceptibility of pathogens. Many professionals and scientists accept that pathogens such as Mycoplasma spp. and non-bacterial organisms such as algae, (Prototheca spp.) and yeast (e.g. Candida spp.) will not respond to antimicrobial treatment and that mild to moderate gram-negative mastitis does not need to be treated with antimicrobials (Lago and Godden, Reference Lago and Godden2018). Some will also argue that culture-negative mastitis cases are unlikely to respond to antimicrobial treatment because there are no viable bacteria to treat, whereas others emphasise that culture results may be false negative. Conversely, detection of gram-positive bacteria is not necessarily indicative of IMI as it may result from contamination of samples or tests, or from presence of clinically non-significant organisms (Nyman et al., Reference Nyman, Persson Waller, Emanuelson and Frössling2016). Here, we discuss ways to assess diagnostic test performance in relation to diagnostic needs before presenting detail of available tests in subsequent sections.
Convenience criteria
In terms of diagnostic capability, farms are resource-constrained settings. To evaluate POC test performance in resource-constrained human health care settings, The ASSURED criteria (Affordable, Sensitive, Specific, User-friendly, Rapid and robust, Equipment-free and Deliverable to end-users) were developed (Kosack et al., Reference Kosack, Page and Klatser2017). They capture scientific criteria (sensitivity, specificity) and convenience criteria (‘cheap’, ‘easy’, ‘quick’), and will be used here as framework for discussion of POC tests for CM.
Dairy production is a sector which often has low profit margins, which might seem to argue for tests with low costs, but price is not necessarily a priority (Griffioen et al., Reference Griffioen, Hop, Holstege, Velthuis and Lam2016). Cost may need to be balanced against ease of use, whereby some of the cheapest systems may require significant user training and hence staff retainment. More expensive systems may have higher ease of use due to automated sample processing, reading or interpretation and may be more robust to changes in environment or personnel. There may also be trade-offs between cost and time. For example, culture-based diagnostics inherently have a slow turn-around due to the time needed for bacterial growth, but they can be very affordable. Conversely, currently available DNA-based diagnostics are fast but require expensive instrumentation. The relative costs of reagents, equipment and labour may be quite different between countries, a phenomenon that is also reflected in the predominance of milking systems (hand milking, milking parlour, or automated milking system) in different parts of the world. Whether low-tech or high-tech, good diagnostic tests cannot compensate for poor quality samples. Any test applied to a contaminated sample is a waste of resources. Surprisingly, not every POC test has ‘contamination’ as a recognised outcome. This may mask the presence of false positives and give high test sensitivity at the expense of test specificity, potentially leading to ‘justification’ of AMU based on detection of contaminants rather than mastitis pathogens. Sample quality, although rarely discussed, may be more important than test characteristics.
Scientific criteria
Diagnostic tests are routinely judged based on the scientific criteria of sensitivity (‘ability to recognise positives’) and specificity (‘ability to recognise negatives’). Tests should be validated in the host species and under the conditions where they are intended to be applied but most published POC test evaluations are laboratory-based rather than farm-based (online Supplementary Table S1). Estimates of scientific criteria are often obtained by comparing the test results with a reference test or ‘gold standard’. Because no test is perfect, no-gold standard comparison or latent class analysis is also used (Nyman et al., Reference Nyman, Persson Waller, Emanuelson and Frössling2016). Outcomes of interest can be presence vs. absence or quantitative thresholds, such as counts of colony forming units in plate-based tests, Ct values in PCR-based analysis, or optical density in colorimetric analyses. When comparing test characteristics across studies, critical interpretation of the results is required as study designs (e.g. definitions, reference tests, thresholds used), study populations (animal and pathogen populations), and methods to calculate confidence intervals may vary and make estimates for scientific criteria study-specific. The summary of POC tests provided in the online Supplementary Table S1 should be read with those cautionary notes in mind. For on-farm application, predictive values are more important than sensitivity and specificity, but they are rarely reported because they are dependent on pathogen prevalence and hence population-specific. A high positive predictive value means that no unnecessary treatment is given (‘treat as little as possible’), which achieves the aim of reducing AMU, whereas a high negative predictive value means that treatment is only withheld when cows truly do not need it (‘treat as much as needed’), so that cow welfare is not compromised.
Level of differentiation
A crucial question is how POC test results inform decision making. Several plate-based assays have been developed to identify mastitis pathogens to species or genus level (see section What do we have?), but the rationale for use of selective treatment for mild to moderate CM is largely based on differentiation of gram-positive vs. other forms of mastitis. Hence, one could argue that a POC test only needs to give information about presence of gram-positive organism. In addition, it needs to distinguish between contamination and IMI. If genus or species level information influences treatment decisions, however, for example by differentiating Streptococcus spp. from Staphylococcus aureus or S. aureus from non-aureus Staphylococci, this is relevant at POC level. In some countries, narrow spectrum penicillins are the 1st line of treatment for CM, in which case differentiation of penicillin-susceptible and penicillin-resistant isolates may be useful, particularly for S. aureus (Barkema et al., Reference Barkema, Schukken and Zadoks2006). Some POC tests now include antimicrobial susceptibility testing (Jones et al., Reference Jones, Bork, Ferguson and Bates2019). When susceptibility testing is conducted at sample level rather than isolate level, without the ability to differentiate between pure cultures, mixed cultures and contamination, the value of such testing to inform treatment decisions is unknown. In addition, its value may differ between farming systems and regions. For example, predominance of Strep. uberis without presence of Escherichia coli as a major cause of CM is uniquely associated with the pasture-based system in New Zealand and predictive values from that system may not translate to other dairy-production systems or regions (Bates et al., Reference Bates, Laven, Bork, Hay, McDowell and Saldias2020). A recent study suggested a 24% reduction of AMU could be achieved when using a POC test with susceptibility testing, yet no change in milk withhold was observed, which is surprising as milk of treated cows should be withheld (Bates et al., Reference Bates, Laven, Bork, Hay, McDowell and Saldias2020).
Societal criteria
Like convenience criteria (cost vs. ease of use) or scientific criteria (sensitivity vs. specificity), societal drivers for POC test use may be at odds with each other. For animal welfare reasons, a ‘security blanket’ of broad-spectrum antimicrobials for all CM cases may be preferred, but this does not sit well with calls to reduce and refine AMU. From an economic point of view, the value of diagnostic tests is situation-specific. Like predictive values, the value of information depends on pathogen prevalence. If most CM is caused by a single species, the cost of testing may not be outweighed by its financial benefits (Cha et al., Reference Cha, Smith, Kristensen, Hertl, Schukken, Tauer, Welcome and Gröhn2016). This line of reasoning does not account for the potential introduction of AMU quota, which, like milk quota, extend the impact of decisions at individual level to other cows in the herd.
Societal concerns may also exist around work health and safety. On-farm bacteriology by non-experienced people is forbidden is some countries because of concerns about propagating pathogens, including hazard group 2 pathogens which can infect and kill people. Several major mastitis pathogens belong to this category, including E. coli, Klebsiella spp., S. aureus, and Streptococcus agalactiae (Zadoks et al., Reference Zadoks, Middleton, McDougall, Katholm and Schukken2011). In addition to concerns about hazardous waste, environmental implications of POC testing may need to be considered. Ideally, POC tests would use materials that are bio-degradable or come from renewable sources.
What do we have?
We currently have POC tests that detect mastitis (inflammation) or pathogen presence (Adkins and Middleton, Reference Adkins and Middleton2018). Inflammation is an indirect measure of infection whereas pathogen detection is a direct measure of infection, with the caveat that pathogen detection without evidence of inflammation may not be sufficient evidence for IMI nor adequate justification for AMU (Nyman et al., Reference Nyman, Persson Waller, Emanuelson and Frössling2016). All currently available on-farm diagnostics for pathogen identification are culture based (online Supplementary Table S1), whilst culture-independent methods are available off-farm or underdevelopment for on-farm use.
Culture-dependent pathogen information
Several formats are available for culture-based POC tests, including petrifilm, agar plates, and tube-based systems. Some identify bacteria as gram-positive, gram-negative, or absent, e.g. MastDecide, Petrifilm, Point-of-Cow, and VétoSlide. Others identify bacteria to genus and species-level, e.g. VétoRapid, Minnesota Easy Culture Tri-plate, Accumast, SSGN plate, Hardy Diagnosis Triplate, Micromast, Dip-Slide Mastitis, Selma and Selma Plus. Some assays include antibiotic susceptibility testing, e.g. Mastatest, Point-of-Cow and Speed Mam colour. All tests are more reliable when used for diagnosis of broad categories (growth, gram-positive, gram-negative) than at genus or species level (Lago and Godden, Reference Lago and Godden2018). Broad categories may suffice to inform treatment decisions but for culling decisions, more detail or accuracy can be desirable. For such decisions, however, TAT is often less critical, and laboratory-based testing may be more appropriate than on-farm testing.
Reported sensitivities for gram-positive bacteria range from 58.6% (MastDecide; Leimbach and Krömker, Reference Leimbach and Krömker2018) to 98% (Minnesota easy culture system Bi-plate; McCarron et al., Reference McCarron, Keefe, McKenna, Dohoo and Poole2009a), and specificities from 48% (Petrifilm; MacDonald, Reference MacDonald2011) to 97% (MastDecide). The inherent trade-off between sensitivity and specificity tends to limit accuracy, although exceptions exist, e.g. for E. coli (online Supplementary Table S1). Low pathogen prevalence in POC test evaluation studies will result in wide confidence intervals around point estimates for test characteristics. Interestingly, Strep. agalactiae is a major pathogen in many emerging dairy industries but almost no information is available on accuracy of POC tests for this organism (online Supplementary Table S1). The market that is currently targeted for POC testing presumes a certain standard of farm management, which would generally be associated with successful control of Strep. agalactiae. Accuracy also depends on observer skills. For example, the ability to detect S. aureus based on haemolysis depends on the population under investigation and the experience of the reader (McCarron et al., Reference McCarron, Keefe, McKenna, Dohoo and Poole2009b). Petrifilm or agar-based tests tend to involve relatively cheap equipment and reagents. However, they require considerable user training, as well as sufficiently high throughput to maintain user skills. Agar-based tests often allow for easy visual identification of sample contamination, which is important to monitor sample quality. Unfortunately, not all user manuals provide guidance on the distinction between positive and contaminated samples, or its importance. In recent market introductions, such as Point-of-Cow and Mastatest (Jones et al., Reference Jones, Bork, Ferguson and Bates2019), consumables and equipment are more sophisticated and more expensive to increase ease of use. Although the test is run on-farm, data interpretation may happen off-farm, using cloud-based computing systems. Issues around data confidentiality and data ownership in such systems may need further consideration, especially if test data is combined with data at cow or farm level. Culture-based tests all have similar TAT of up to 24 h. Although this is fast enough to inform decision making, farmers would prefer less delay with turn-around from one milking to the next (Griffioen et al., Reference Griffioen, Hop, Holstege, Velthuis and Lam2016).
Culture independent pathogen information
Commercially available culture-independent pathogen detection systems are currently based on polymerase chain reaction (PCR), a method to selective amplify target DNA of interest. Targets may be genus specific, species specific, or indicative of AMR, as in the case of the blaZ gene encoding penicillin resistance in Staph. aureus. DNA amplification is possible even when bacteria are non-viable or in a viable but non-culturable state, which enables pathogen detection in culture-negative samples. This is probably more relevant for samples that are shipped to a laboratory than for POC testing and use of PCR is largely limited to professional laboratories. Whether detection of bacterial DNA in culture negative samples provides justification for AMU is an open question (Nyman et al., Reference Nyman, Persson Waller, Emanuelson and Frössling2016). Growing recognition of the existence of a mammary microbiota, and descriptions of mastitis as a ‘dysbiosis’, suggest that presence of DNA in low quantities may be normal. Studies on associations between treatment and cure have historically been culture based. If or how treatment impacts on the outcome of CM cases with PCR-positive, culture-negative results is untested.
To combine the scientific characteristics of DNA-based testing with the convenience characteristics of on-farm testing, loop-mediated isothermal amplification (LAMP) of DNA is explored. This test uses different chemistry than PCR, requires less sample preparation, and is less sensitive to inhibitory substances present in biological samples (Bosward et al., Reference Bosward, House, Deveridge, Mathews and Sheehy2016). It can be configured as a pregnancy test-like lateral flow device, which could be a rapid and cost-effective on-farm diagnostic (Cornelissen et al., Reference Cornelissen, De Greeff, Heuvelink, Swarts, Smith and Van der Wal2016). LAMP has received attention in the scientific literature as a means to make molecular diagnostics feasible and affordable in low resource settings. Its implementation as on-farm POC test for CM is hampered by heterogeneity of mastitis pathogens and resistance determinants and the limited ability for multiplex testing. The latter may be addressed through developments in microfluids (see Future developments).
Future developments
Technological development progresses extremely quickly, demonstrated by revolutionary changes in computing, mobile phone technology, and DNA-sequencing over recent decades. The biology of CM in dairy cattle has not changed as much over the same period, although developments in genetics and husbandry have led to significant shifts in milk production and predominant pathogen populations in developed countries. Societal attitudes towards AMU have changed more recently and influence availability and use of antimicrobials for CM treatment. Possibly most resistant to change is human behaviour, which drives the need for as well as the development and uptake of diagnostics. In this section, we discuss opportunities to harness the power of the technological revolution, the data revolution, and the people that milk or manage cows (Fig. 1).
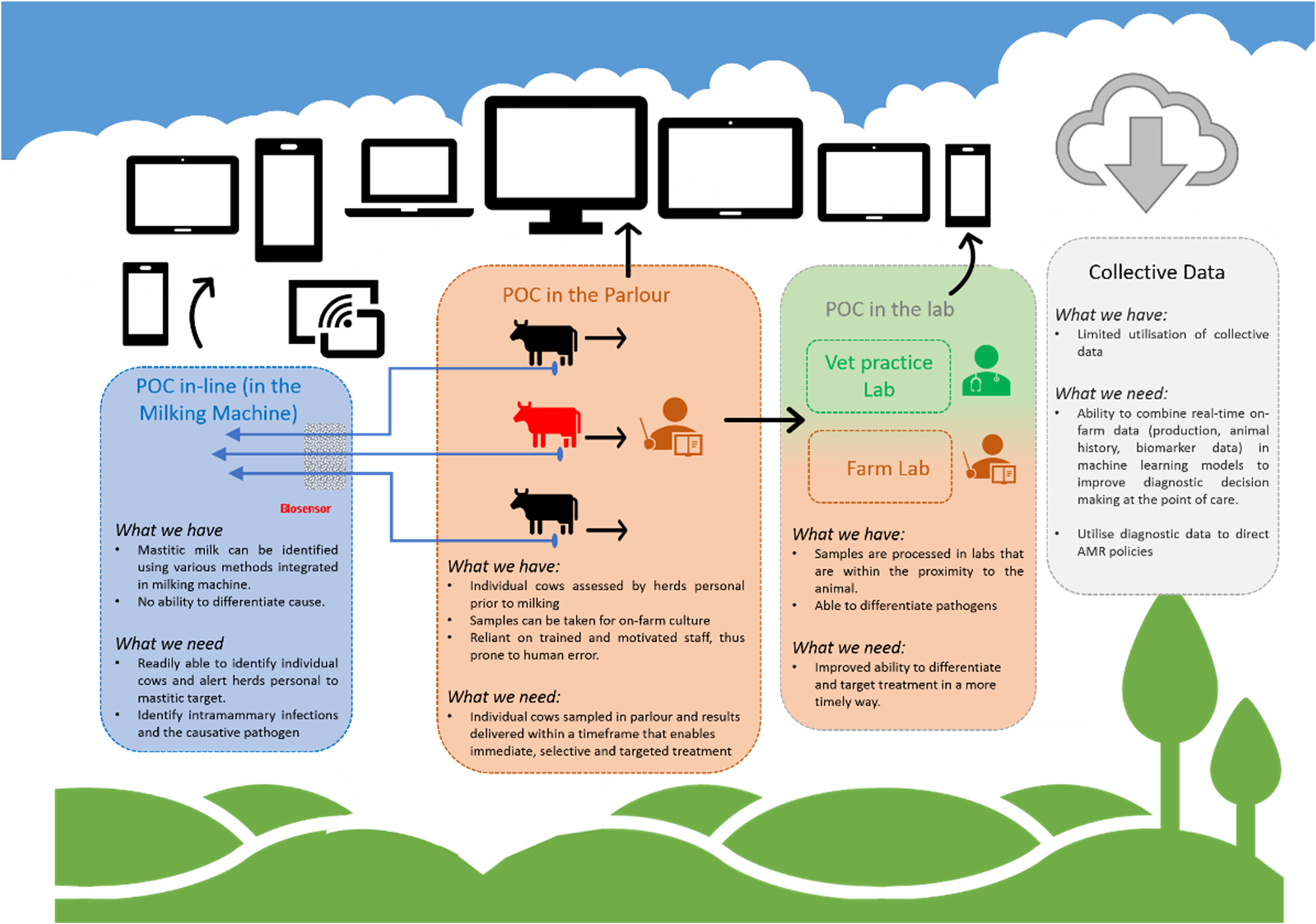
Fig. 1. Point-of-care tests for bovine clinical mastitis. What do we have and what do we need.
Harnessing the power of technology
Advances in microfluidics allow development of technologies that can be incorporated into automatic monitoring systems and portable devices for sensitive and rapid mastitis diagnostics (Viguier et al., Reference Viguier, Arora, Gilmartin, Welbeck and O'Kennedy2009). After somatic cell count, perhaps biomarkers such as acute phase proteins (APPs) are the most widely studied inflammatory biomarkers in milk. Haptoglobin (Hp) and milk-amyloid A (MAA) are especially prominent members of this group in dairy cattle and both are synthesised locally in mammary tissue. To inform treatment decisions, biomarker patterns would need to be pathogen-specific. Whilst there is some evidence to support this approach, which could be enhanced by multiplex assays linked to machine learning technology (see next section), it is not always clear whether APP profiles reflect severity of inflammation or causative agents and concerns exist about sensitivity and specificity (Pyörälä et al., Reference Pyörälä, Hovinen, Simojoki, Fitzpatrick, Eckersall and Orro2011). Small inflammatory mediators such as cytokines and chemokines have shown significant promise with proteomic platforms differentiating the host response to different pathogens (Kusebauch et al., Reference Kusebauch, Hernández-Castellano, Bislev, Moritz, Røntved and Bendixen2018), and progress is being made in the development of biosensors for detecting such biomarkers (reviewed by Martins et al., Reference Martins, Martins, Cardoso, Germano, Rodrigues, Duarte, Bexiga, Cardoso and Freitas2019). Increasingly, the line between biomarker detection and pathogen detection is blurred, as culture-free pathogen detection becomes feasible on microfluidic devices popularly known as ‘lab on a chip’. One of the key challenges in the development of such devices is the need to detect multiple targets. We are not aware of developments aimed at differentiating gram-positive and other causes of mastitis, although identification of a few major gram-positive mastitis pathogens might suffice to support on-farm treatment decisions. If so, paper-based multiplex LAMP assays may provide a low-cost option that is fast, microbiologically safe (no pathogen amplification, paper can be burned after use) and environmentally sustainable (Reboud et al., Reference Reboud, Xu, Garrett, Adriko, Yang, Tukahebwa, Rowell and Cooper2019).
Harnessing the power of data
Technological developments should be accompanied by developments in data analysis to maximise the benefit from increasingly sophisticated biomarker or pathogen detection systems.
Machine learning methods became popular for many applications in which predictive performance is the main aim. This type of narrow artificial intelligence has been applied to mastitis diagnosis, focusing on real-time detection from milking data. Artificial neural networks (ANN) and tree based models perform best in this context (Ebrahimie et al., Reference Ebrahimie, Ebrahimi, Ebrahimi, Tomlinson and Petrovski2018; Ebrahimi et al., Reference Ebrahimi, Mohammadi-Dehcheshmeh, Ebrahimie and Petrovski2019), linking parameters such as milk yield, electrical conductivity, and lactose level to SCM. This black-box type of model is optimised for prediction at the cost of interpretability, which is a good trade-off for real-time mastitis diagnosis. Adding more layers of weights to ANNs gives rise to the ‘deep learning’ models that are successful in many fields. Dhoble et al. (Reference Dhoble, Ryan, Lahiri, Chen, Pang, Cardoso and Bhalerao2019) recommends neural networks also for label-free flow cytometry data to routinely screen for mastitis.
By contrast, simple classification tree models are easy to interpret, and cut-off values calculated from them are easily applicable in POC tests such as lateral flow immunoassays. More complex models (random forests, gradient boosted trees) gain predictive accuracy at the cost of interpretability (Ebrahimi et al., Reference Ebrahimi, Mohammadi-Dehcheshmeh, Ebrahimie and Petrovski2019). Like neural networks, complex tree models are mostly black boxes to the user. Machine learning methods are more flexible than classic multivariate statistical methods, requiring fewer assumptions about the data generating mechanisms and independence of various measurements. They can combine multiple data types into a single prediction model, making them useful for mastitis diagnosis based on a combination of demographic, epidemiological, milking, flow cytometry, and biomarker data. However, the lack of human oversight in the model specification process is also the drawback of many machine learning models. Biases in the data on which these models are trained tend to get reinforced in the model fitting process, reducing the applicability of the model to the wider animal/farm population. Training data sets should therefore be representative of the target population, and also relatively large to avoid overfitting.
In the era of cheap sensors, the goal will be to develop rapid (semi-)automated diagnostic systems that apply artificial intelligence to real-time data streams (e.g. from automated milking) and biomarker information to distinguish between gram-positive and gram-negative pathogens. Differences in baseline parameters between farms will pose a challenge, which can be overcome by either standardising the machine learning models, or retraining them for use on new farms (Ebrahimi et al., Reference Ebrahimi, Mohammadi-Dehcheshmeh, Ebrahimie and Petrovski2019). Ideally, data from diagnostic systems would be combined with prognostic factors, such as duration of infection (SCC and CM history), parity, as well as measures of cow value (genetic merit and lactational performance) to weigh the probability of treatment success against the value of the individual animal. Studies on prognostic indicators are limited but suggest the importance of similar factors across multiple gram-positive organisms (Barkema et al., Reference Barkema, Schukken and Zadoks2006, Samson et al., Reference Samson, Gaudout, Schmitt, Schukken and Zadoks2016).
Harnessing the power of people
Scientific research has generated sufficient knowledge to support targeted treatment of non-severe CM based on pathogen identification and cow factors. However, this knowledge is hardly implemented and farmers frequently experience ‘insecurity’ and ‘uncertainty’ about mastitis treatment (Swinkels et al., Reference Swinkels, Hilkens, Zoche-Golob, Krömker, Buddiger, Jansen and Lam2015). Such feelings may motivate farmers to seek social approval and emulate peer behaviour, for example by extending treatment where increased AMU is perceived to be ‘better’ (Swinkels et al., Reference Swinkels, Hilkens, Zoche-Golob, Krömker, Buddiger, Jansen and Lam2015). Ideally, the same social phenomenon would be harnessed to reduce AMU, e.g. by training ‘champions’ of antimicrobial stewardship and using peer networks to spread prudent AMU. Farmer-led approaches rather than traditional passive knowledge transfer methods may be the best way to motivate change in AMU or POC test use (Bard et al., Reference Bard, Main, Haase, Whay, Roe and Reyher2017). In addition, communication approaches like motivational interviewing, which are designed to facilitate clients' internal motivation to change may improve uptake of veterinarian advice (Bard et al., Reference Bard, Main, Roe, Haase, Whay and Reyher2019; Svensson et al., Reference Svensson, Lind, Reyher, Bard and Emanuelson2019). Demographic factors and affective attributes, such as a veterinarian's age, respectfulness and dominance, or a farmer's education level also influence farmers' satisfaction and willingness to adopt veterinary advice (Ritter et al., Reference Ritter, Adams, Kelton and Barkema2019). Such insight could be implemented to promote POC tests uptake and targeted treatment approaches.
Farmers and veterinarians are part of food production and health care systems that may provide barriers or incentives for behaviour change. Perceived barriers at farm level include lack of time, economic investment with no financial short-term benefits and labour shortages (Ritter et al., Reference Ritter, Jansen, Roche, Kelton, Adams, Orsel, Erskine, Benedictus, Lam and Barkema2017). Drivers for uptake may include improvement of cows' welfare and farm profitability, long-term job satisfaction, reputational benefits in relation to consumer demands, social recognition and pride, and the desire to conform to perceived standards of ‘being a good farmer’ (Swinkels et al., Reference Swinkels, Hilkens, Zoche-Golob, Krömker, Buddiger, Jansen and Lam2015). In some countries AMU quota have been implemented successfully or recommended to reduce AMU (Bos et al., Reference Bos, Mevius, Wagenaar, van Geijlswijk, Mouton and Heederik2015; O'Neill, Reference O'Neill2016). Benchmarking of individual AMU against average AMU of peers or at national level can provide a sense of being part of a nationwide reduction campaign and may encourage POC test uptake, as farmers are more inclined to assume their responsibility as a part of joint effort (Ritter et al., Reference Ritter, Jansen, Roche, Kelton, Adams, Orsel, Erskine, Benedictus, Lam and Barkema2017). It would be naïve, however, to ignore the role of commercial drivers in uptake of selective treatment to reduce AMU. Many veterinarians generate income from sales of antimicrobials, and this may serve as a disincentive for promotion of targeted treatment. In many countries, antimicrobial use and sales are not under veterinary control, which makes the issue even more complicated. Contracts and demands from milk processors and retailers may override preferences or recommendations from farmers and veterinarians. The balance between ‘stick’ (forced reduction in AMU) and ‘carrot’ (financial and reputational benefits from reduction in AMU) will differ between countries and production systems and change over time.
Final thoughts
The 3Rs of Reduction, Refinement and Replacement are well known in the context of experimental animal research. The same concepts could be applied to antimicrobial treatment of mastitis. Reduction can be achieved if AMU for non-severe CM is targeted to gram-positive IMI only. As reviewed here, this may require further improvements in convenience characteristics and scientific criteria of POC tests and changes in knowledge, attitudes and behaviours among veterinarians and dairy farmers. Behaviour change should also include refinement, whereby we should abstain from the use of HP-CIA in dairy cattle (Turner et al., Reference Turner, Tisdall, Barrett, Wood, Dowsey and Reyher2018). Professionals in the dairy industry, processors, retailers, pharmaceutical companies and regulators all have a role to play in reduction and refinement as they buy milk, or produce, approve and market antimicrobial products. Ideally, refinement of herd management practices would reduce the incidence of mastitis and the need for treatment. The concept of replacement is used in dry cow treatment, where internal teat sealants increasingly replace antimicrobials as tools for infection prevention. To some extent, anti-inflammatory and supportive therapy can act as replacements in treatment of lactational CM. They are not causative in removing the infectious agent but contribute to a successful host response. Alternatives that target mastitis pathogens, such as biocins and phage therapy, require further development (reviewed by Angelopoulou et al., Reference Angelopoulou, Warda, Hill and Ross2019).
Regardless of the need for further research on replacement, we already have the tools and the knowledge to reduce and refine the use of antimicrobials in treatment of lactational mastitis. The onus is on the veterinary profession, the farming industry, and associated industries and regulators to decide how best to incentivise and implement them.
Supplementary material
The supplementary material for this article can be found at https://doi.org/10.1017/S002202992000062X
Acknowledgements
This article is based upon work from COST Action FA1308 DairyCare, supported by COST (European Cooperation in Science and Technology, www.cost.eu). COST is a funding agency for research and innovation networks. COST Actions help connect research initiatives across Europe and enable scientists to grow their ideas by sharing them with their peers. This boosts their research, career and innovation. Additional funding was received from Innovate UK (grant reference TS/R020515/ Rapid, accurate, on-farm diagnosis and monitoring of mastitis in dairy cows).