An Approach for Assimilating FY4 Lightning and Cloud Top Height Data Using 3DVAR
- 1Research Center for the Development of the Earth System Model of Lanzhou University, College of Atmospheric Sciences, Lanzhou University, Lanzhou, China
- 2NOAA National Severe Storms Laboratory, Norman, OK, United States
- 3Cooperative Institute for Mesoscale Meteorological Studies, University of Oklahoma, Norman, OK, United States
The vertical distribution of water vapor affects the intensity of the updraft, downdraft and cold pool in convection, so how to adjust lightning proxy-humidity in the vertical direction is very important for convective scale numerical weather prediction (NWP). In this study, a data assimilation approach is presented that uses information from FengYun4 (FY4) lightning data with cloud top height (CTH) data. Specifically, the FY4 CTH is used to locate the upper boundary of the relative humidity adjustment. This method can effectively determine the vertical distribution of water vapor and obtain accurate pseudo-observations. Two severe convection events with different characteristics were studied to evaluate the data assimilation approach for short-term precipitation forecast. For comparison, two other relative humidity adjustment schemes with different vertical ranges were performed. One scheme adjusted the relative humidity between two isothermal layers and introduced the smallest water vapor increments compared with the other two data assimilation experiments and showed a slight improvement on precipitation forecast. The other scheme adjusted the relative humidity between the lifting condensation level (LCL) and a fixed height and introduced the maximum water vapor increments and exhibited better precipitation forecast based on Equitable Threat Scores (ETSs). The adjustment between LCL and CTH introduced appropriate amounts of water vapor and was adaptable for various convection developments and effectively avoided producing spurious convection in the particular case. Assimilation of FY4 lightning and CTH data improves the short-term precipitation forecast and provides the best forecast skill in a particular forecast period.
Key Points
• A FengYun4 (FY4) lightning data assimilation (LDA) approach was presented.
• The FY4 cloud top height was used to locate the upper boundary of the relative humidity adjustment in LDA.
• The LDA approach improves short-term convective precipitation forecasts.
Introduction
The hazardous weather induced by mesoscale convection systems (MCSs), including thunderstorms, gusts, hail, tornados and rainstorms, causes serious damage to lives and property. Timely and accurate forecasting of these hazardous weather events is key for saving lives and protecting property. However, accurate MCS forecasting is difficult because of imperfect initial numerical conditions that lack meso- and/or small-scale convective information and multiscale dynamic and thermodynamic consistency (Liu et al., 2010; Yi et al., 2011; Xiao et al., 2013). To improve the accuracy of MCS prediction, it is important to assimilate mesoscale and small-scale observations into numerical weather prediction (NWP) models (Sun, 2005; Pu et al., 2009; Sun and Wang, 2013; Wang H. et al., 2013). Lightning observations are good proxies for convective scale systems.
Lightning is an electrical manifestation of thermodynamic and mechanical activities associated with convective storms. Convective updrafts within storms determine the supply, growth and transport of water condensates to the upper regions, and these updrafts directly lead to the charge separation of lightning (Anagnostou and Chronis, 2007; Papadopoulos et al., 2009). There is a well-known relationship between the occurrence of total lightning activities and deep convective updrafts and precipitation (Goodman et al., 1988; MacGorman et al., 1989; Qie et al., 1993; Wiens et al., 2005; Fierro et al., 2006). Lightning is regarded as an indication of severe convection and is used to determine the possible location of deep convection. Many studies have shown the positive impact of assimilating lightning data into NWP models to improve MCS predictions (Alexander et al., 1999; Chang et al., 2001; Papadopoulos et al., 2005; Mansell et al., 2007; Fierro et al., 2012, 2014, 2016; Wang et al., 2014, 2018; Yang et al., 2015).
Lightning observation technology has developed rapidly in recent decades. The U.S. National Lightning Detection Network (NLDN) detects only cloud-to-ground (CG) flashes covering the western U.S. (Cummins et al., 1998) but not intracloud (IC) flashes. Most CG flashes occur during the maturation and extinction stages of a thunderstorm. IC flashes, however, accounting for most of the natural lightning occurrences, occur most often in the initial and development stages of a thunderstorm. Studies (MacGorman et al., 1989; Schultz et al., 2011; Fierro et al., 2012) have shown an excellent correlation between IC flashes and the updraft strength of deep continental storms. The Earth Networks Total Lightning Network (ENTLN) uses TOA detection methodology with Global Positioning System (GPS) technology and sophisticated algorithms to locate and classify lightning types. The ENTLN sites of electric field recorders are also limited by topographic conditions and cannot detect lightning flashes in areas with complex terrain. Furthermore, the ENTLN reports lightning as a point observation, which also limits its scope. The wide frequency range enables sensors to detect CG strokes and also weaker IC pulses. Consequently, there is low efficiency in IC lightning detection.
Compared with ground-based lightning detection, lightning instruments mounted on geostationary satellites have the advantages of wide coverage and few limitations related to topography. Lightning detection on geostationary satellites is continuous in the field of view from the platform of the geostationary orbit, which is the most effective measure of lightning flashes. The Lightning Mapping Imager (LMI) onboard FengYun 4 (FY4) is the first lightning detection sensor on a Chinese satellite. The LMI continuously measures the total lightning activity over China day and night (Yang et al., 2017). Assimilation of FY4 total lightning data to improve the prediction of severe convection in China is the subject of this research. Since lightning is not a model state variable, it is the key to establishing a reliable relationship between lightning observations and model state variables for assimilation.
In a particular climatic regime and a limited geographic region, there is a well-established relationship between lightning and convective precipitation (Zipser, 1994; Alexander et al., 1999). Alexander et al. (1999) inferred rain rates from lightning data combined with Special Sensor Microwave/Imager (SSM/I) rain-rate field and infrared (IR) brightness temperatures. Then, the lightning-converted rainfall rates were assimilated into the Mesoscale Model version 5 (MM5) by modifying the latent heat profiles. Chang et al. (2001) considered a more realistic relationship between the convective rainfall rate and lightning rate. Pessi and Businger (2009) implemented a similar scheme as those in Alexander et al. (1999) and Chang et al. (2001) that assimilated the rainfall rates in the Kain–Fritsch (KF) convective parameterization scheme (CPS) by modifying the model-generated vertical latent heating profiles. However, there is no universal relationship between lightning flash density and rainfall, and the relationship between lightning and rainfall is sensitive to storm location, especially whether they occur over land or water (Zipser, 1994; Price and Rind, 1992; Petersen and Rutledge, 1998; Alexander et al., 1999).
In general, the lack of sufficient fine-scale information and the use of coarse grid resolutions are the reasons for the failure to predict convection initiations at the model’s initial time (Kain and Fritsch, 1990). Therefore, improving the local environment (particularly moisture and temperature) initially for the mesoscale model by using lightning as a proxy variable has the potential to provide accurate predictions of convection initiations. Papadopoulos et al. (2005) developed an assimilation technique in the Betts-Miller-Janjic (BMJ) CPS, in which lightning data were used to determine the areas of convection, and thereafter, the model-generated humidity profiles were nudged to an empirical profile to activate deep convection. Mansell et al. (2007) used lightning data to control the KF CPS, which either activated the subgrid-scale deep moist convection by adding water vapor up to a fixed threshold or partially suppressing the KF by limiting the “boost” given to parcels by the trigger function [thereby making it harder to reach the level of free convection (LFC)] and reducing the KF updraft width. Subsequent studies by Lagouvardos et al. (2013) and Giannaros et al. (2016) implemented a similar approach with a different model.
Fierro and Reisner (2011) and Fierro et al. (2012, 2014) conducted assimilations of simulated Geostationary Lightning Mapper (GLM) total lightning data derived from ENTLN using convection-resolving (1 km) and convection-allowing (3 km) models. When a flash occurred in a given column, water vapor was added to the mixed-phase region within the column (defined as the layer between the 0 and −20°C isotherms) via a smooth continuous function of the gridded flash rate and the simulated graupel mixing ratio. Qie et al. (2014) established a relationship between flash rate and ice particle content based on the non-inductive electrification mechanism, which is a direct relationship between lightning and ice-phase particle content in thunderclouds. During the assimilation, the model ice-phase particle mixing ratio was adjusted between two temperature layers from 0 to −20°C isotherms, which represents the ice-particle-rich region within convection and hence is most likely associated with electrification, and thus the lightning activities occur according to the non-inductive charging mechanism. Using an assumed relationship between flash density and radar reflectivity, Wang et al. (2014) converted lightning data into three-dimension radar reflectivity as a lightning proxy, which was assimilated via the physical initialization method. In addition, a temperature adjustment scheme was also studied by Marchand and Fuelberg (2014), which warmed the most unstable low levels of the atmosphere at locations where lightning was observed but deep convection was not simulated.
The direct adjustment of the initial environment via the lightning proxy variable can activate convection well, but the adjustment is often arbitrary and can cause inconsistencies with the surrounding environment. To obtain initial fields that are close to the observations and consistent within the model, the variational method and ensemble techniques were used in the lightning data assimilation (LDA). Mansell (2014) performed an Observing System Simulation Experiment (OSSE) that applied a linear relation between flash extent density (FED) and graupel echo volume to assimilate the lightning data using the ensemble Kalman Filter (EnKF) method. Allen et al. (2016) studied two storm cases by assimilating real ground-based lightning mapping arrays simulated GLM FED observations using the EnKF method. However, the EnKF method cannot initiate convection where all the ensemble members have zero graupel volume despite the linear relationship between graupel and flash density (Marchand and Fuelberg, 2014). Fierro et al. (2016) utilized FED to create pseudo-observations of water vapor mass and then assimilated these observations into the model using a three-dimensional variational (3DVAR) method. Wang Y. et al. (2017); Wang et al. (2018) developed a retrieval procedure that uses total lightning rates to retrieve the graupel mixing ratio (qg), and then, the retrieved qg was assimilated as observations using a time-lagged ensemble method.
However, lightning data were used only to determine the horizontal location of convective activities in previous LDA studies, and these data were not used to determine the vertical height and extent of convection. In general, the vertical adjustment range of the lightning proxy variables is determined by the isothermal layer of the background or fixed height. The vertical range of water vapor adjustments in the isothermal layer (0 to −20°C) of the background environment is a convenient approach (Fierro et al., 2012; Qie et al., 2014). However, this approach depends heavily on the accuracy of the background environmental temperature, whereas large errors in the background temperature field make it possible that convection was not forecasted. Fierro et al. (2016) obtained water vapor pseudo-observations from the lifting condensation level (LCL) up to a fixed upper height of 15 km. This scheme is not suitable for strong local convection with large variations in the vertical direction.
The concept of a pseudo water vapor field derived from lightning data with empirical relationships that is assimilated into NWP models has been adopted by many researchers (Fierro et al., 2014, 2016; Wang Y. et al., 2017). The vertical distribution of water vapor affects the intensity of the updraft, downdraft and cold pool and thus affects the storm morphology, retention time and intensity of convection. When using water vapor as a lightning proxy variable for assimilation, if the adjustment range of water vapor in the vertical direction cannot be determined accurately, pseudo-observations with many pieces of inaccurate water vapor information will be assimilated into the NWP. Based on the above discussions, this work attempts to address two problems: (1) how to determine a more accurate range for the adjustment of water vapor in the vertical direction during the assimilation of lightning data and (2) what impact was produced using the different vertical adjustment ranges of water vapor on the analysis and forecast experiments.
According to the electrification mechanism inside thunderstorms, lightning occurs in clouds where water vapor is saturated or nearly saturated. Using the LCL as a surrogate for the cloud base, once the cloud top height (CTH) is determined, the three-dimensional distribution of saturated or near-saturated water vapor can be estimated along with the horizontal locations of lightning. In this study, FY4 CTH is used to locate the upper boundary of the relative humidity adjustment instead of the isothermal layer or the fixed upper height, which were used in previous studies. Two other LDA experiments are also implemented to determine the impacts produced using the different vertical adjustment ranges for relative humidity in the analysis and forecast. Two severe convective events that occurred over northern and southern China in June 2018 were examined to determine the impact of assimilating the lightning data using CTH, fixed height and isothermal layers. In section “Data and Method,” the setup of the model simulation and the severe convective events are described, and the data used for assimilation and assimilation procedures are introduced. The results, including the analysis and forecast field, are presented in section “Results.” The conclusions and discussion are provided in section “Conclusion and Discussion.”
Data and Method
Model Setup
The numerical model used for this study is the three-dimensional compressible non-hydrostatic Weather Research and Forecasting model version 3.7.1 (WRF3.7.1; Skamarock et al., 2008). All of the simulations are configured with a model domain (Figure 1) that has a horizontal resolution of 9 km and 50 terrain-following ETA levels from the surface up to 50 hPa in the vertical direction. Initial and lateral boundary conditions are provided with the 6-hourly 0.25° Global Forecast System (GFS) datasets. The main physical schemes used include the WRF single-moment 6-class (WSM6) microphysical parameterization (Hong and Lim, 2006), the Rapid Radiative Transfer Model for General Circulation Models (RRTMG) scheme for longwave and shortwave radiation (Iacono et al., 2008), the Yonsei University planetary boundary layer scheme (Hong et al., 2006), the thermal diffusion scheme for the land surface model (Dudhia, 1996) and the KF cumulus parameterization scheme (Kain, 2004).
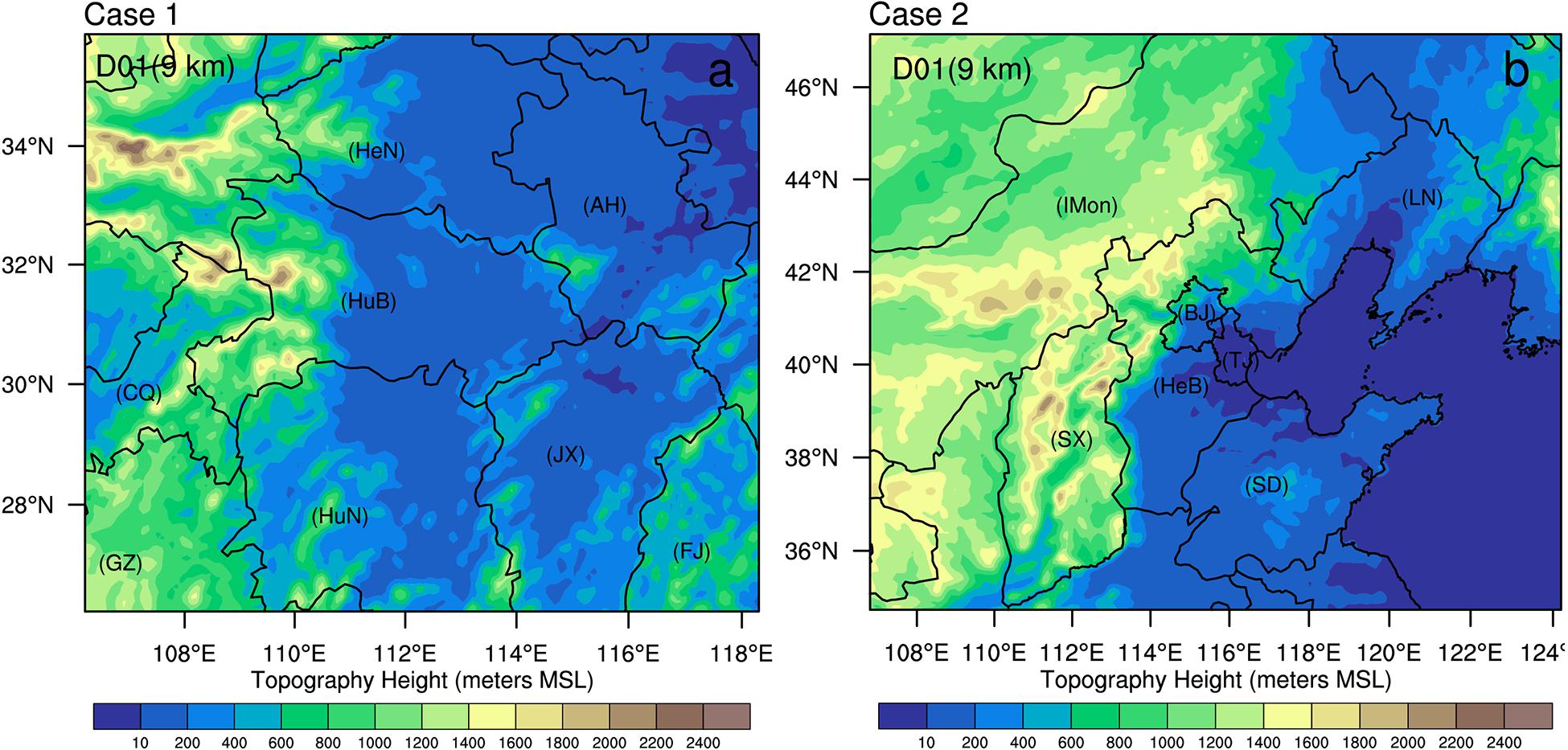
Figure 1. Configuration of the WRF model domain for two cases. (a) Case 1 for 30 June 2018. The abbreviations for Henan, Anhui, Hubei, Chongqing, Guizhou, Hunan, Jiangxi, and Fujian Provinces are HeN, AH, HuB, CQ, GZ, HuN, JX, and FJ, respectively. (b) Case 2 for 12 June 2018. The abbreviations for Inner Mongolia, Liaoning, Beijing, Tianjin, Hebei, Shanxi, and Shandong Provinces are IMon, LN, BJ, TJ, HeB, SX, and SD, respectively. The colors represent model terrain heights.
Description of the Severe Convective Events
Two severe convection events with different characteristics were studied to evaluate the data assimilation approach for short-term precipitation.
Case 1
A severe convection event occurred in the middle and lower reaches of the Yangtze River under the influence of the Meiyu front around 30 June 2018. Discrete storms first developed in western Anhui Province and northern Jiangxi Province at approximately 2000 UTC on 29 June 2018. After a few hours, these discrete storms gradually developed and merged into MCSs (Figure 2a). At 0300 UTC on 30 June, the maximum convective available potential energy (CAPE) exceeded 1700 J/kg (Figure 2b), and there was a low-pressure system in the southern region of Hubei Province. The lowest pressure reached 1001 hPa (Figure 2b). The southwest wind near the surface provided favorable water vapor conditions for the convection system. The convective system eventually caused heavy precipitation at a rate of more than 20 mm per hour in Hubei Province.
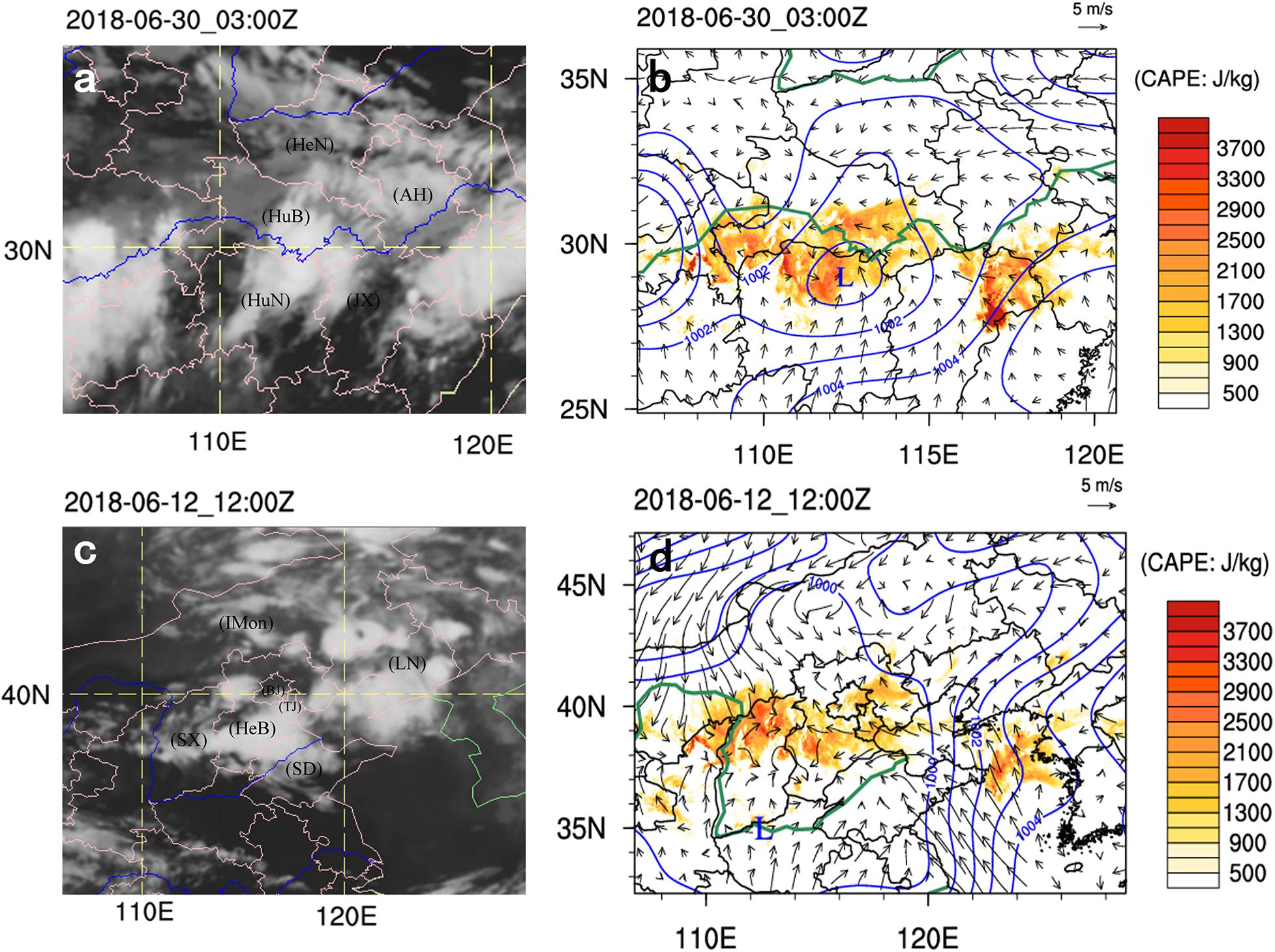
Figure 2. Infrared image from the FY2 geostationary satellite (a,c) and the synoptic analysis derived from the 0.25° Global Forecast System (GFS) reanalysis (b,d). The shaded colors in (b,d) represent the maximum convective available potential energy, the solid blue lines represent the contours for sea level pressure, and wind arrows are for the 10 m wind vectors. (a,b) Case 1 at 0300 UTC on 30 June 2018. (c,d) Case 2 at 1200 UTC on 12 June 2018. The abbreviations are the same as in Figure 1.
Case 2
A severe convection event occurred on 12 June 2018 in North China under the influence of the Northeast Cold Vortex (NCV), initially developing over northwestern Beijing and Hebei, moving southeastward through central Hebei, Beijing and southern Liaoning (Figure 2c). There was a strong north wind near the surface in central Inner Mongolia and a strong south wind near the surface in southern Shandong (Figure 2d). The severe convection events brought heavy precipitation and accompanied lightning and hail.
Data
The lightning data used in this study were retrieved from the FY4 LMI, which continuously measures the total lightning (IC and CG) activities over China 24 h a day. The spatial resolution of the data is approximately 7.8 km at nadir. The LMI 400 × 600 pixel charge-coupled device (CCD) camera operates at 777.4 nm to count flashes and measure their intensity (Yang et al., 2017), and the detection efficiency exceeds 90%. Since March 2018, the Chinese National Satellite Meteorology Center (NSMC) has provided publicly downloadable LMI products with 1 min intervals. The horizontal positions of lightning events were interpolated to the nearest model grid points from the lightning event points. To accommodate more lightning data in the NWP model, the lightning event time window was traded off at 30 min before and after the assimilation time.
The CTH product is derived from the Advanced Geosynchronous Radiation Imager (AGRI) mounted onboard FY4. The NSMC provides CTH data channels for the full disk and China region with a 4 km spatial resolution, and they are updated approximately every 15 and 4 min in real time, respectively. CTH provided useful information about the height of convective clouds in this study. The nearest neighbor method was used to interpolate the original CTH product to the model grid points at the assimilation time.
Hourly site accumulation precipitation observations and China Meteorological Administration (CMA) Land Data Assimilation System version 2.0 (CLDAS v2.0) precipitation product provided by the Chinese National Meteorological Information Center (NMIC) were used to assess the model’s performance for precipitation. The CLDAS v2.0 dataset contains an hourly 0.0625° precipitation field that merges in situ surface precipitation observations, 0.0625° hourly East Asian Multi-Satellite Integrated Precipitation products, a fusion precipitation product from the Fengyun-2 satellite, and numerical analysis/predicted precipitation products from the GFS with a temporal resolution of 3 h and a spatial resolution of 0.25° (Shi et al., 2014; Liu et al., 2019). Hourly site accumulation precipitation was used for case 1, and the CLDAS-V2.0 precipitation product was used for case 2. To reach the precipitation score, the nearest neighbor method was used to interpolate the observations of accumulated precipitation at each site to the model grid points.
Assimilation Method
The assimilation procedure in this study consisted of two parts. The first part obtained the pseudo-observations of relative humidity based on the lightning data and CTH observations. The second part assimilated the pseudo-observations of relative humidity into the NWP model using a 3DVAR method. Figure 3 shows the flow chart of this LDA procedure. In the first part, the relative humidity adjustment was performed within the cloud columns determined using lightning data, i.e., the relative humidity was set to 90%. The threshold was based on Wang et al. (2014). The lightning data within the time window between 30 min before and 30 min after the assimilation time were used for the humidity adjustment. When the lightning frequency was greater than or equal to 1 times/hour in a column, that is, at the location where the lightning occurred, the relative humidity from the background at that column was adjusted to 90% if it was less than 90%. In particular, when the relative humidity was greater than or equal to 90% at that location, there was no adjustment. The CTH was determined using the CTH observations from FY4. The calculated LCL from the background fields was approximated as the cloud base height. The LCL was calculated using equation (1) (Sheng, 2003). Where T and TD are the temperature and dew point at a height of 2 m, respectively.
This approximation refers to Wang et al. (2014) and describes the characteristics of moisture in a convection environment (Fierro et al., 2016). The adjusted relative humidity at each grid point was saved as a pseudo-observation of the relative humidity at that location.
In the second component, the pseudo-observations of relative humidity obtained above were assimilated into the WRF model through the WRF data assimilation (WRFDA) 3DVAR system (Barker et al., 2004). The 3DVAR method has achieved numerous beneficial results in many early studies (Gao et al., 2004, 2013; Gao and Stensrud, 2012; Wang Y. et al., 2013, 2017; Lai et al., 2019) and has been proven to be useful for assimilating conventional observations, radar data and satellite data. The purpose of 3DVAR is to obtain an optimal estimate of the true atmospheric state at analysis time by minimizing a prescribed cost-function (Ide et al., 1997):
The minimization of equation (2) can be solved through iterations, and the analysis state x is the state vector that minimizes J(x). The xb and y0 are the first guess (or background) and observations, respectively. B and O are the background and observation (instrumental), respectively. The background error covariance B is estimated in grid space using the National Meteorological Center (NMC) method (Parrish and Derber, 1992).
Results
Four experiments (Table 1) for each convection case are designed in this section. Case 1 is initialized at 1800 UTC on 29 June 2018, case 2 is initialized at 0300 UTC on 12 June 2018. There are 6 h for spin-up period. The control experiment (CTL) assimilates no observations. The three LDA experiments for each case are designed to evaluate the impact of pseudo-observations of relative humidity and FY4 CTH on the short-term precipitation forecasts. The first LDA experiment (LDA_CTH) adjusts the relative humidity between the LCL and FY4 CTH based on lightning observations. The second LDA experiment (LDA_15km), following Fierro et al. (2016), adjusts the relative humidity between the LCL and a fixed upper height (15 km). The third LDA experiment (LDA_T020) is based on the experiments in Fierro et al. (2012) and Qie et al. (2014), which adjust the relative humidity between the 0°C and −20°C isotherm layers.
There are similar changes to the analysis field and forecast in case 1 and case 2. To avoid too much repeated content being displayed, a detailed analysis is conducted for case 1. The innovations of relative humidity in the analysis field and the difference in various forecast variables between the LDA experiments and CTL are analyzed in sections “The analysis fields” and “The forecast fields” for case 1. The simulated precipitation performances of case 1 and case 2 are evaluated against precipitation observations in section “Evaluation of precipitation forecasts.”
The Analysis Fields
According to Bielli and Roux (1999) and Ge et al. (2013), in addition to the horizontal and vertical winds, the most important field for convective scale NWP is humidity available for convection initiation. When the atmosphere is dry, there is less water vapor condensation to form cloud water or cloud ice, i.e., less release of latent heat to support upward motion. Thus, convection does not develop. Therefore, an accurate estimate of the low-level water vapor field is essential for convective storms. After assimilating the pseudo-observations of relative humidity, a positive increase in relative humidity in the analysis field is obvious at the locations of lightning flashes, which provides the necessary condition for saturated water vapor in the environment. Figure 4 shows the increments of relative humidity at 700 hPa. In LDA_T020 (Figure 4c1), the positive increments of relative humidity are small. This result is due to the lack of moisture and thermodynamic conditions that support the development of convection in the background. With no strong updraft in the environment, the 0°C isothermal layer is relatively close to the −20°C isothermal layer, and the adjustment of relative humidity in the environment with lightning data is insufficient. The assimilation of lightning data at the initial time aids in convection initiation but does not help much for simulating existing convection or suppressing spurious convection. However, the effective use of lightning data is also related to the model background information. Therefore, if no convection exists in the background, LDA_T020 cannot trigger convection initiation.
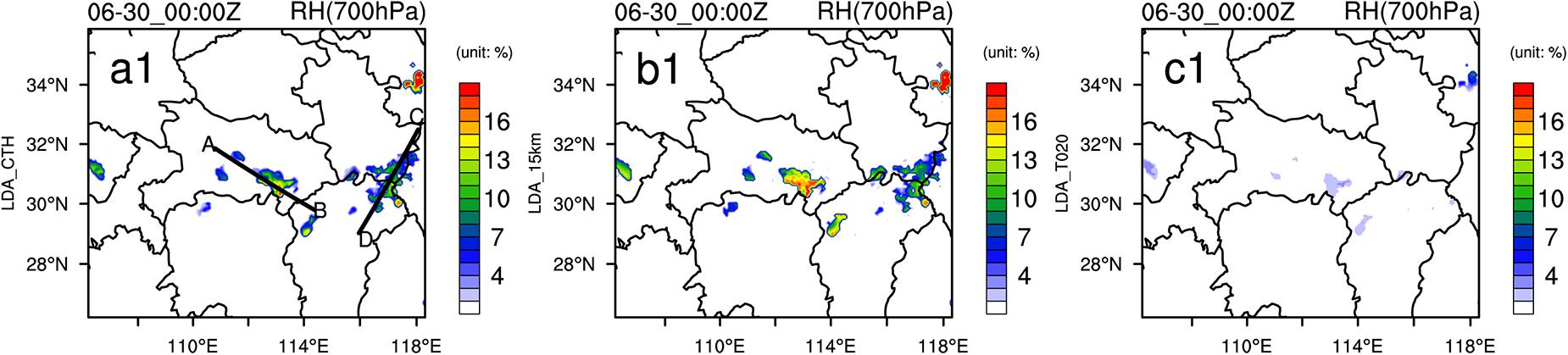
Figure 4. Analysis increments of relative humidity (RH) for the LDA_CTH (a1), LDA_15km (b1) and LDA_T020 (c1) experiments at 700 hPa at 0000 UTC on 30 June. The black lines AB and CD in (a1) denote the locations of the vertical cross sections for subsequent figures.
Figure 5 shows vertical cross sections of the relative humidity for the pseudo-observation minus the background and the analysis increments. LDA_T020 shows the smallest amount of relative humidity in all three assimilation experiments. LDA_15km exhibits more positive relative humidity increments between 200 and 700 hPa than LDA_CTH. For the cross section along line AB, approximately 10% relative humidity increments from LDA_15km could reach a height of 150 hPa near 112°E. The same increments also hold in the cross section along line CD and near 118.8°E. This result occurs because the relative humidity is adjusted between the LCL and a fixed 15 km level with LDA_15km at horizontal lightning locations, even if the convection cannot reach that high or above that height. The increments of humidity are flat along longitude at high levels in the cross section in LDA_15km. However, the CTH should be different at each horizontal position. The increments of humidity for LDA_CTH change along the longitude in the cross section. At 150 hPa (less than 15 km), the pseudo-observation of LDA_15km minus background shows a large positive difference between 112.0°E to 112.5°E. Because the FY4 CTH does not reach a height of 15 km (Figure 6d), there is almost no positive difference at this location in LDA_CTH (Figures 5a1,a2). In the 3DVAR method, to satisfy the physical consistency and dynamic coordination, this higher “observed” relative humidity in the upper layers of LDA_15km affects the relative humidity in the lower layers, which greatly increases the relative humidity in the lower layers of the analysis field. If the relative humidity at a fixed height is adjusted without considering the actual convection top height, an excessive amount of water vapor may also be created in the lower layer, which introduces spurious convections.
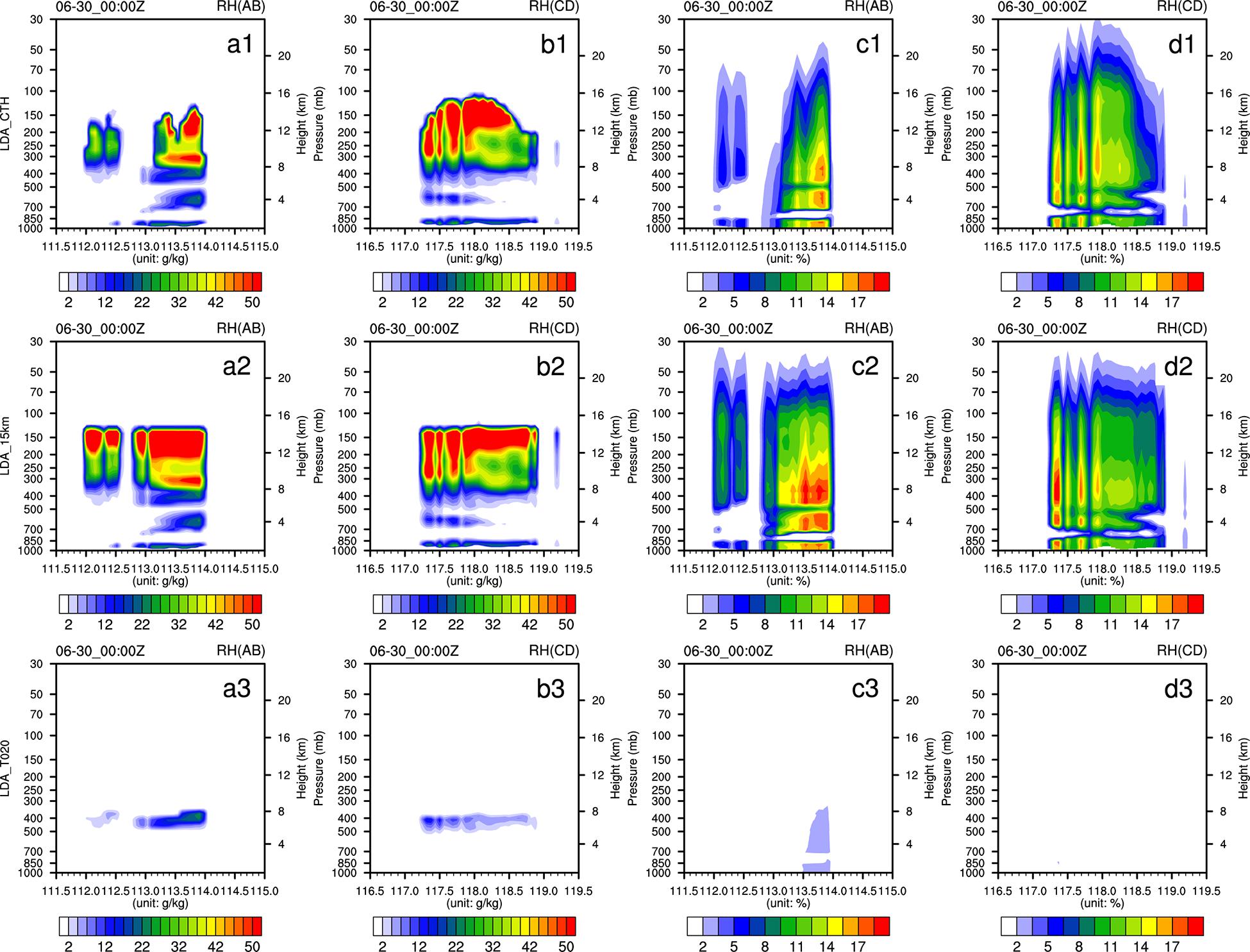
Figure 5. Vertical cross sections of the relative humidity (RH) for the pseudo-observation minus the background (a1–a3,b1–b3) and the analysis increments (c1–c3,d1–d3) along lines AB and CD in Figure 4 a1 for 0000 UTC on 30 June 2018. The first row shows LDA_CTH, the second row shows LDA_15km, and the third row shows LDA_T020. The first and third columns show cross section AB, and the second and fourth columns show cross section CD.
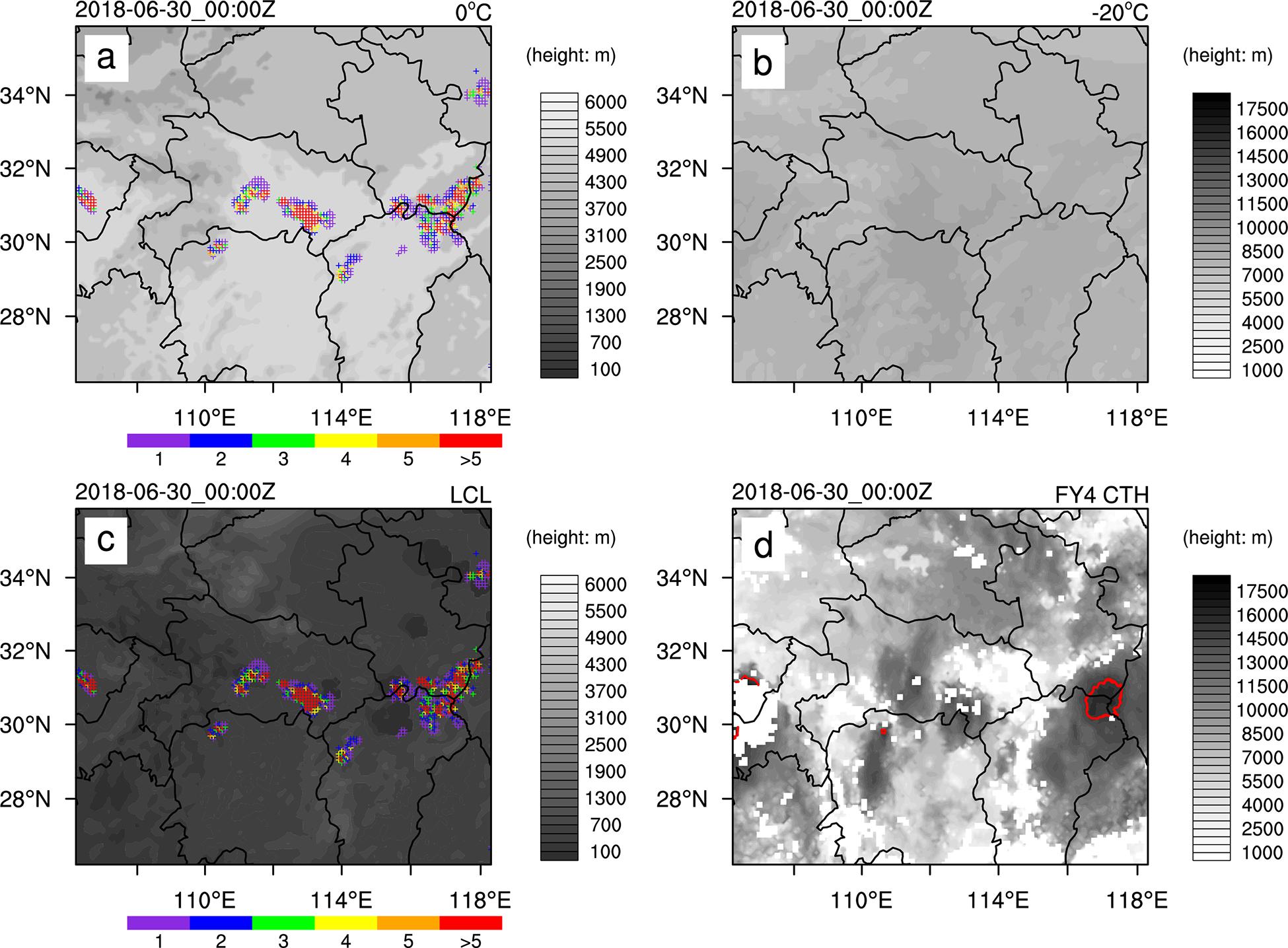
Figure 6. The heights of 0°C (a) and −20°C (b) in the background field, LCL (c) and FY4 CTH (d) (gray-shaded) at 0000 UTC on 30 June 2018. The color marker (in a,c) indicate the frequency of lightning occurrence within 1 h of FY4. The red contours (in d) are for the FY4 CTH 15 km heights.
To illustrate the importance of different vertical adjustment ranges, Figure 6 shows plots of the height of the 0°C layer (Figure 6a), the height of the −20°C layer (Figure 6b) in the background field, LCL (Figure 6c) and FY4 CTH (Figure 6d) at the analysis time. The height of the 0°C layer in the background is much higher than that of the LCL, and the height of the −20°C layer in the background is also lower than that of the FY4 CTH. Therefore, the adjustment of relative humidity in LDA_T020 is very small, which explains why LDA_T020 has the smallest water vapor increments. At the time of assimilation, FY4 CTH can reach 15 km in only limited regions, for example, near the border between Anhui and Jiangxi provinces. Lightning is indeed observed over northwest Jiangxi Province, but the CTH observation is far less than 15 km. LDA_15km adds an excessive amount of humidity in northwest Jiangxi Province, causing spurious convection to develop at this position (shown in a later section). The use of the FY4 CTH observations as the upper boundary of relative humidity adjustment is adaptable for various convection developments and effectively avoids the problem associated with fixed height adjustment as in LDA_15km because not all convections develop up to a fixed height. In case 2, the same information can be find in Figures 7a–c.
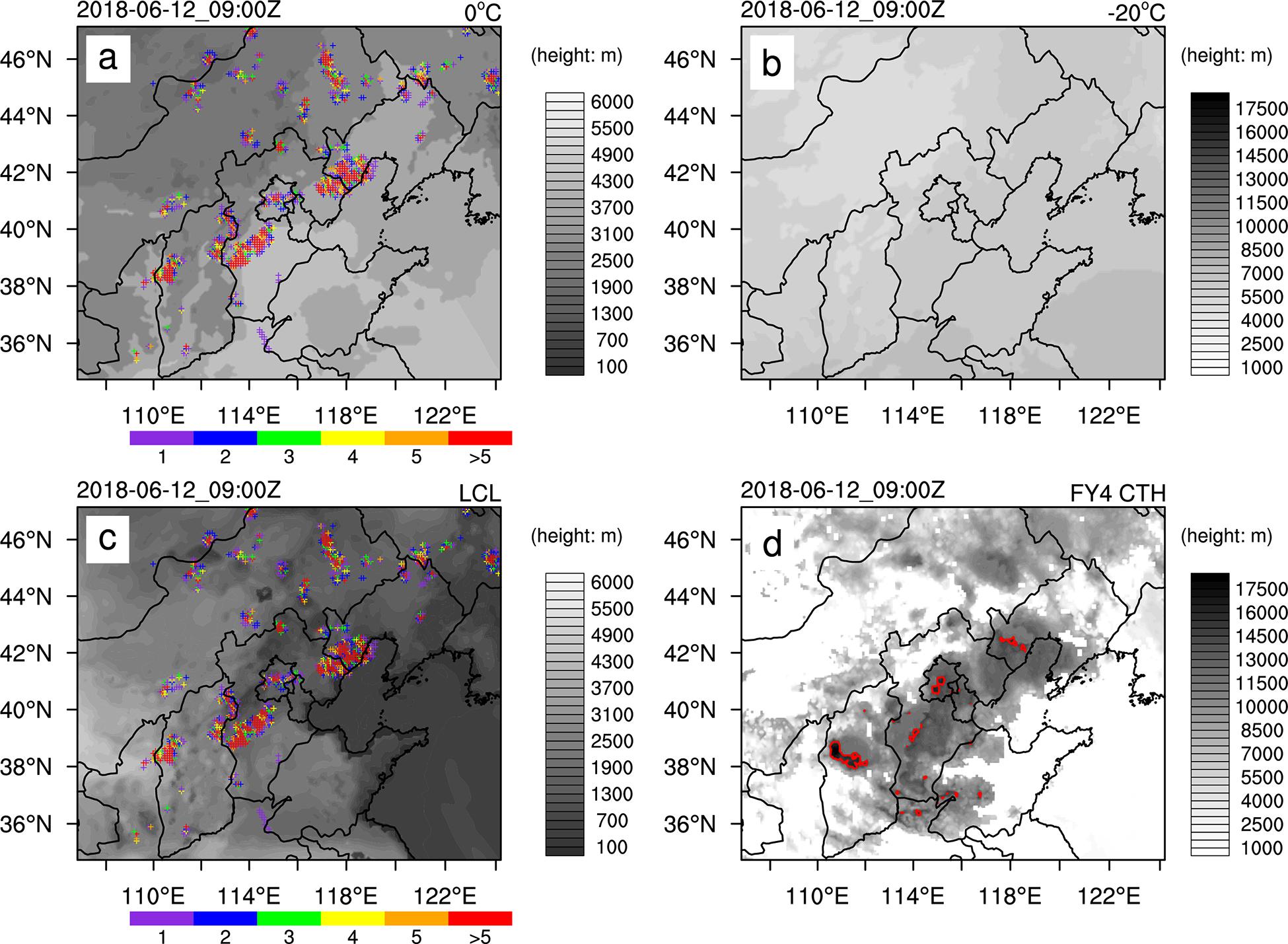
Figure 7. The heights of 0°C (a) and −20°C (b) in the background field, LCL (c) and FY4 CTH (d) (gray-shaded) for case 2 at 0900 UTC on 12 June 2018.
The Forecast Fields
The effects on the 1-h forecast with LDA are analyzed for each experiment of case 1 in this section. Because the forecast from LDA_T020 is very close to that of the CTL, the result is not shown in this section.
The Wind Field
Figure 8 shows the difference in horizontal wind vectors at 700 hPa and the vertical cross sections of the u-w vectors, v-w vectors, and vertical velocity after a 1-h forecast at 0100 UTC on 30 June for LDA_CTH minus CTL and LDA_15km minus CTL. There were weak low-level inflows and updrafts in southeastern Hubei Province near the border between Anhui Province and Jiangxi Province in the CTL. Both LDA_CTH and LDA_15km generate strong wind field convergence and positive vertical velocity at this position, and there is a negative vertical velocity around the positive vertical velocity in the area southeast of Hubei Province (Figures 8a1,a2). It is obvious that the low-level updraft inflows are enhanced at the locations with large water vapor increments in both LDA_CTH and LDA_15km.
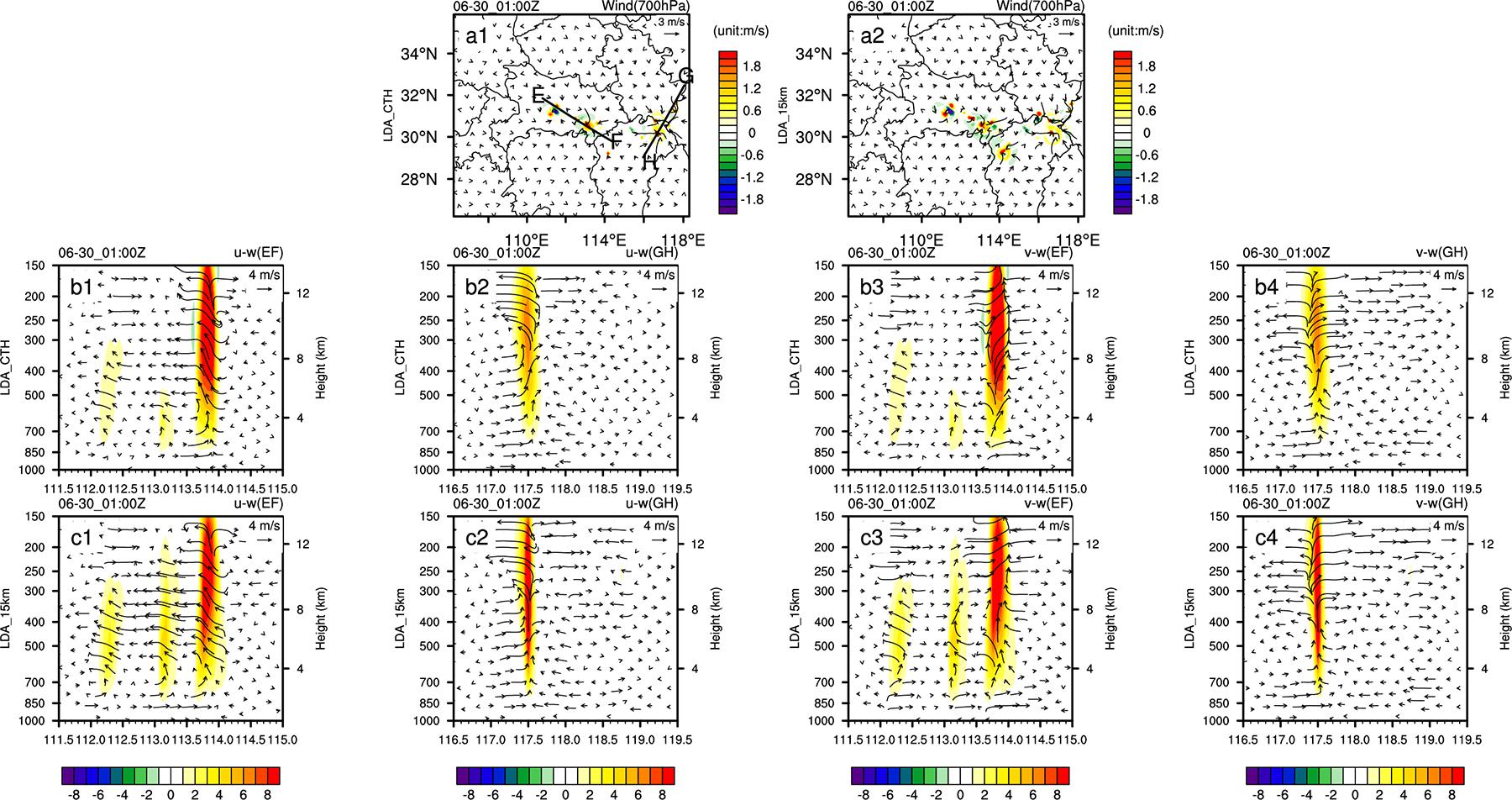
Figure 8. The difference in horizontal wind vectors (a1,a2) at 700 hPa and vertical cross sections of the u-w vectors (b1,b2,c1,c2), v-w vectors (b3,b4,c3,c4), and vertical velocity between LDA_CTH (a1 and the second row)and LDA_15km (a2 and the third row) with CTL for 0100 UTC on 30 June 2018. The legend for the colors for the vertical velocity is shown to each panel. The black lines EF and GH in (a1) denote the locations of the vertical cross sections for subsequent figures. For vertical cross sections, the first and third columns show the cross section along line EF, and the second and fourth columns show the cross section along line GH.
The difference in the u-w vectors, and v-w vectors in the vertical cross sections between LDA_CTH and CTL, as well as those between LDA_15km and CTL is shown in Figure 8. There is a positive vertical velocity difference near 113.8°E in Figures 8b1,b3,c1,c3) and near 117.5°E in Figures 8b2,b4,c2,c4). The strong updraft aids the movement of the moist air in the lower layer to the upper layer. Below 700 hPa, the stronger convergent flow replenishes the moist air entrained by the updraft (Figures 8b2,c2). The assimilation experiments strengthen the convergent flow at the low level, supplement the surrounding water vapor in the center of convection, and cause condensation of the rising water vapor. The increased divergent flow at the upper level sustains the divergence of the top mass and heat of the convective cloud, which aids convection development and maintenance.
Comparing LDA_CTH and LDA_15km, the wind convergence in LDA_15km is stronger than that of LDA_CTH in southeast Hubei. LDA_15km generates a strong convergence center in northwest Jiangxi Province with a positive vertical velocity difference (Figure 8a2), which is not present in LDA_CTH (Figure 8a1). This result indicates that assimilating pseudo-observations of relative humidity obtained at a fixed height (15 km) is associated with a large increase in water vapor, which produces additional convective cells in the forecast. The vertical cross section of the wind fields shows that LDA_15km generates stronger vertical velocities than LDA_CTH (Figures 8b1,b3,c1,c3). LDA_15km produces more intense divergent flow near 113°E with stronger divergent winds than those produced by LDA_CTH. These results show that for LDA, the choice of adjustment range for water vapor in the vertical direction affects the variation in water vapor increments in the analysis field and then affects the local horizontal circulation of convection and thus the updraft in the forecast. When the adjustment range of the water vapor is so high that it exceeds the development height of the actual convection, the convection is unrealistically strengthened, and spurious convection cells can be activated.
The Temperature Field
Accurate thermodynamic conditions are necessary for convection initiation in the model. Figure 9 shows the difference in the 1-h temperature forecast between the assimilation experiments and the control run. In the lower layers, there is a negative temperature difference at the location of water vapor increments introduced by the lightning data, possibly accredited to the cooling of precipitation or inflow of cold moisture near the ground (Figure 8). With respect to the horizontal distribution of the 700 hPa temperature difference (Figure 9a1,a2), there is a positive temperature difference southeast of Hubei Province near the border between Anhui Province and Jiangxi Province. This temperature difference reduces convective inhibition and increases CAPE, which provides the necessary thermodynamic condition for convection development. There is a positive temperature difference above 700 hPa, similar to the “warm tongue” at the location of the relative humidity increments in vertical cross sections, which coincides with the location of the strong updraft. It is shown that the updraft moves the moisture airflow from the low and middle levels to the upper layer, where the water vapor is saturated and the condensation releases latent heat to warm the surrounding atmosphere. In the LDA experiments, the −20°C isothermal layer is higher than that in the control experiment. When the isothermal layers of the background are used to determine the adjustment range of water vapor in the vertical direction, there will be a significant difference in thermodynamic conditions between the background and actual convection environment. Therefore, the water vapor adjustment range is inaccurate, and convection initiation cannot be properly simulated in LDA_T020. This phenomenon explains why the increments of relative humidity in LDA_T020 are significantly smaller than those in the other LDA experiments. The large relative humidity increments in LDA_15km, on the other hand, introduce more water vapor condensation at approximately 500 hPa and result in the largest positive temperature difference.
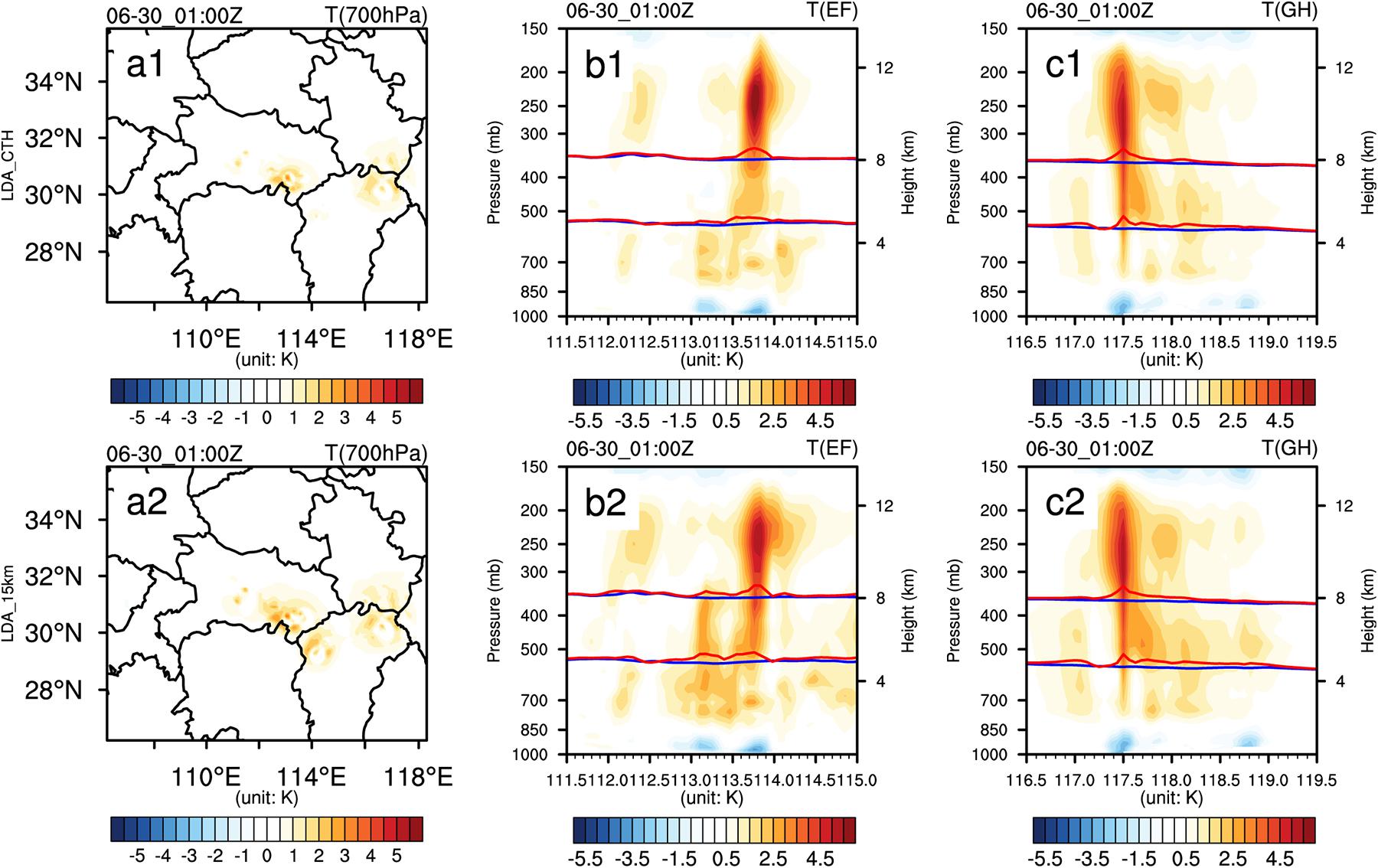
Figure 9. The temperature difference between LDA_CTH and CTL (a1,b1,c1) and between LDA_15km and CTL (a1,b2,c2) at 700 hPa for 0100 UTC on 30 June 2018. The locations of the vertical cross sections are denoted with black lines EF and GH in Figure 8 (a1). The second column shows the cross sections along line EF, and the third column shows the cross section along line GH. The red lines (b1,c1 for LDA_CTH, b2,c2 for LDA_15km) are the 0 and −20°C isothermal levels, and the blue lines are those for CTL.
The Hydrometeor Fields
With constant aerosol and temperature environments, more water vapor will cause more condensation to form droplets and/or ice crystals. After the pseudo-observations of relative humidity are assimilated, the formation process of cloud droplets and ice crystals are also similarly affected by the change in water vapor and wind vectors in the environment. Thus, the hydrometeor mass is also changed. Figure 10 shows the difference in hydrometeor fields between the two LDA experiments (LDA_CTH and LDA_15km) and the control experiment at 0100 UTC on 30 June. Between 113.5°E and 114°E along line EF (see Figure 8a1), the cross section shows a large positive difference in the graupel mixing ratio above 500 hPa. The large positive difference in the snow mixing ratio appears above 300 hPa (i.e., −20°C isothermal level). The positive difference in the rain mixing ratio appears below 500 hPa and coincides with the positive difference in vertical velocity in Figure 8. The difference along line GH is similar to that along line EF, i.e., the vertical positions of the large change in hydrometeor coincide with the positions of the positive change in the updraft. This finding indicates that positive increments of water vapor at those locations where lightning occurs at the analysis time cause the change in wind fields at subsequent forecasting times, and the updraft is enhanced at those locations. Lightning occurs where ice crystals and graupel collide with each other during a thunderstorm. These differences also reflect the dynamic conditions and microphysical processes in the thunderstorm that produce the observed lightning. The graupel mixing ratio, the snow mixing ratio and the rain mixing ratio in the cross section along line EF for LDA_CTH and LDA_15km are different, and the positive change in the hydrometeors for LDA_15km is greater than that for LDA_CTH. The difference in the hydrometeor fields between LDA_CTH and LDA_15km is credited to the difference in vertical extensions when performing a relative humidity adjustment, which introduces different dynamics and microphysical processes within the cloud. Therefore, it is important to determine an appropriate vertical adjustment range for the model proxy variable when performing LDA.
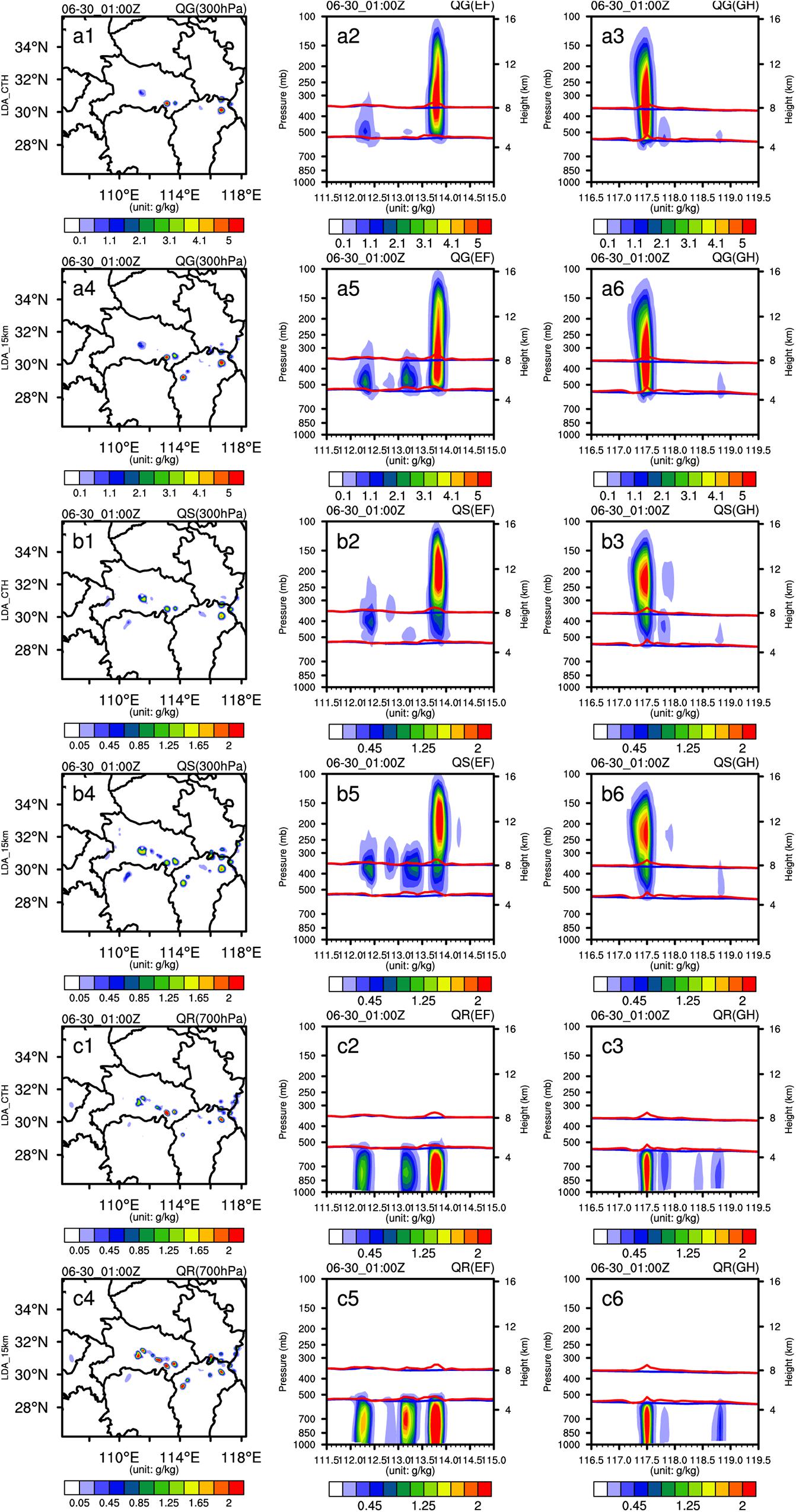
Figure 10. The hydrometeor field differences at 0100 UTC on 30 June 2018 for the graupel mixing ratio (QG) (a1–a6), snow mixing ratio (QS) (b1–b6) and rain water mixing ratio (QR) (c1–c6) between LDA_CTH and CTL (a1–a3,b1–b3, c1–c3) and between LDA_15km and CTL (a4–a6,b4–b6,c4–c6). The first column shows the difference for QG at 300 hPa, QS at 300 hPa and QR at 700 hPa. The second column shows the cross sections along line EF, and the third column shows the cross sections along line GH.
Evaluation of Precipitation Forecasts
The precipitation forecast performance for case 1 and case 2 is evaluated according to the spatial distribution pattern of 6-h accumulated precipitation, and the Equitable Threat Score (ETS) and missing rate (MR) for 1-h precipitation forecasts against observations are also calculated. ETS = 1 defines a perfect forecast, and ETS = 0 defines a forecast without any skill. MR = 1 indicates that all observation stations are missed, and MR = 0 indicates that all observation stations are accurately forecasted (Brill and Mesinger, 2009).
Case 1 of 30 June 2018
Figure 11 shows the spatial distribution of the observed and simulated 6-h accumulated precipitation from 0000 UTC to 0600 UTC on 30 June. Figure 12 shows ETS and MR against observed data for 1-h accumulated precipitation in the simulated region with thresholds of 1 and 5 mm, respectively. The 1 mm and 5 mm thresholds correspond to precipitation occurrence and strong precipitation, respectively. The spatial distribution (Figure 11a) of the observed 6-h accumulated precipitation shows three strong precipitation (over 30 mm) bands in the model domain. The three strong precipitation bands include one in central Hubei Province, one over southeast Hubei Province and the other near the border between Jiangxi Province and Anhui Province. All experiments simulate the strong precipitation center in central Hubei, but the placement is slightly west of its actual location. LDA_CTH (Figure 11d) and LDA_15km (Figure 11e) simulate the strong precipitation observed southeast of Hubei and near the border between Jiangxi Province and Anhui Province. CTL (Figure 11b) and LDA_T020 (Figure 11c) forecast strong precipitation in southeast Hubei Province with a small magnitude and fail to produce precipitation areas near the border between Jiangxi Province and Anhui Province. LDA_15km produces a strong spurious precipitation center northwest of Jiangxi Province.
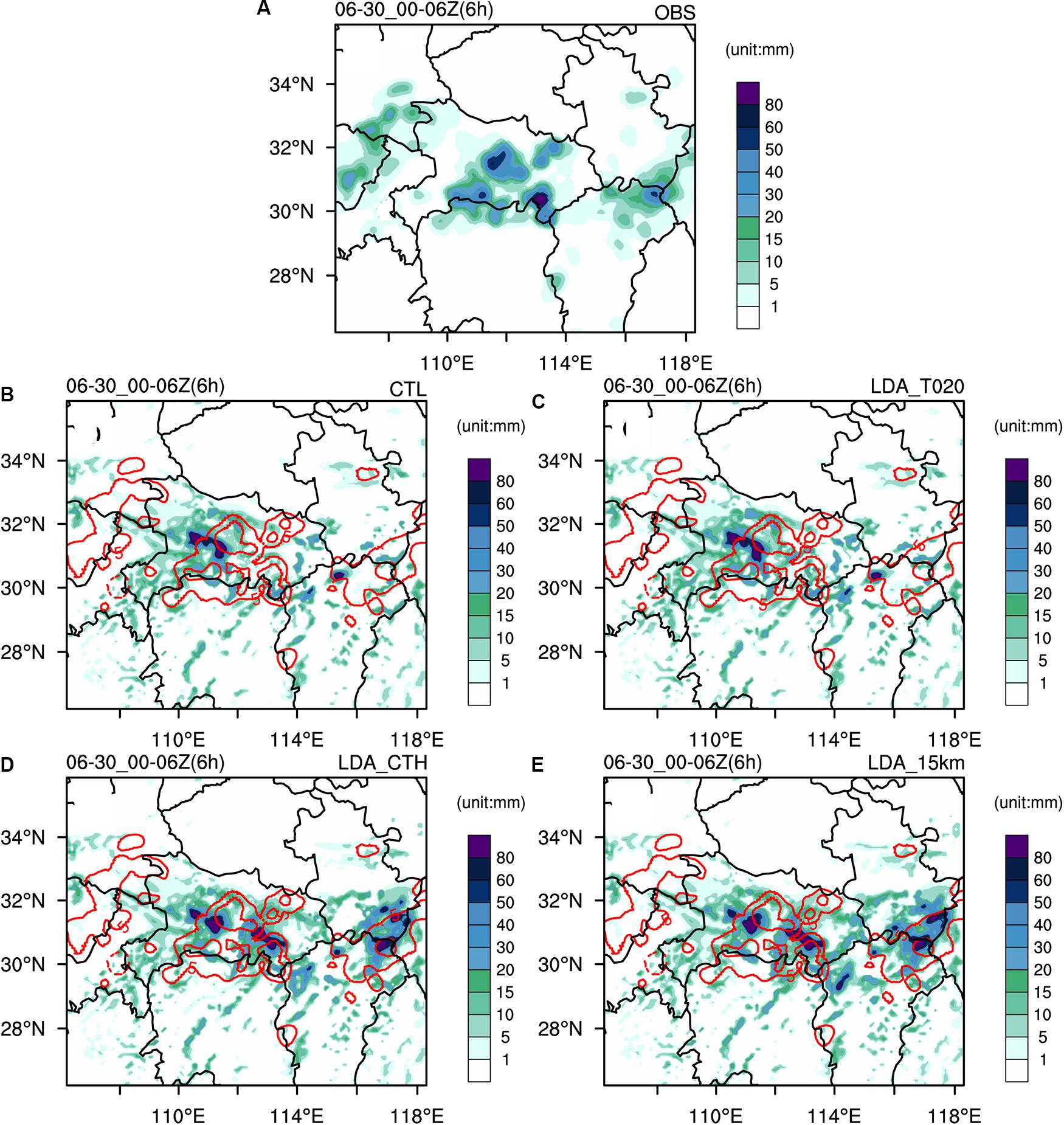
Figure 11. Observations and forecasted 6-h accumulated precipitation from 0000 UTC to 0600 UTC on 30 June for each experiment. (A) Observations (OBS), (B) CTL, (C) LDA_T020, (D) LDA_CTH, and (E) LDA_15km. The contours for observations (5 mm, 30 mm) are highlighted with red lines in the panels for LDA experiments (B–E).
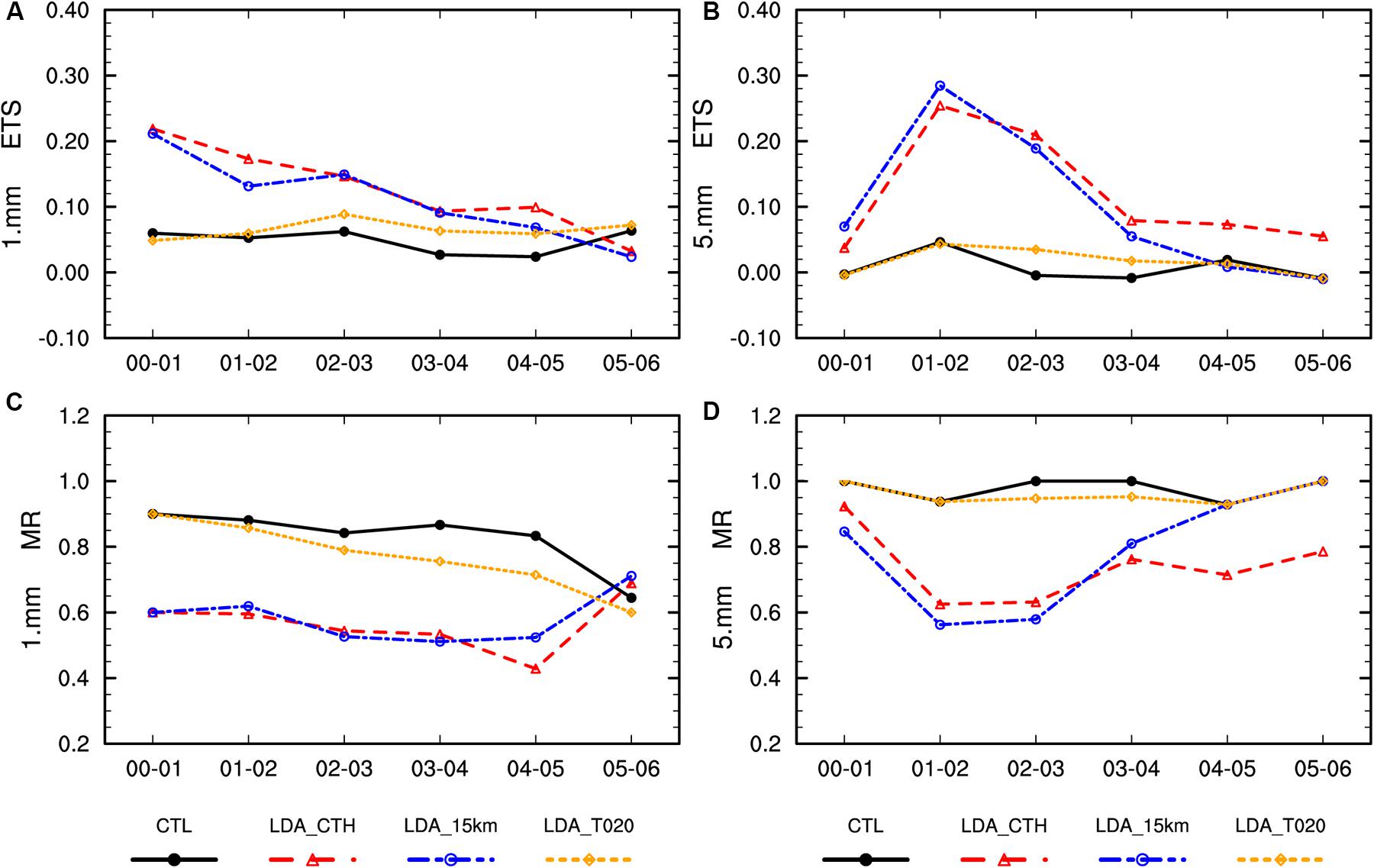
Figure 12. The equitable threat score (ETS) (A,B) and missing rate (MR) (C,D) of the simulated hourly accumulated precipitation against the observations from 0000 UTC to 0600 UTC on 30 June. The thresholds for precipitation are 1 mm (A,C) and 5 mm (B,D).
For the results of ETS and MR in Figure 12, LDA_CTH and LDA_15km are much better than LDA_T020 and CTL for both the 1 mm and 5 mm thresholds. For the 1 mm threshold, LDA_T020 is better than CTL. For the last 5 h with the 5 mm threshold, LDA_T020 scores a higher ETS than CTL most of the time. Thanks to the assimilation of the relative humidity pseudo-observations with LDA_T020, the increments of relative humidity, though small (Figure 5), still have a positive impact on the subsequent precipitation forecast. For the 1 mm threshold, the ETSs of LDA_CTH and LDA_15km are similar, and the MR of LDA_CTH is less than that for LDA_15km, especially after 4-h forecasts. For the 5 mm threshold, the ETSs of LDA_CTH are close to those of LDA_15km most of the time, while LDA_15km has a slightly higher ETS in the first 2 h. After 2 h in the forecast, LDA_CTH has a higher ETS than LDA_15km. This difference occurs because LDA_15km produces more relative humidity increments at the analysis time than LDA_CTH. Therefore, the model activates convection more quickly and results in a higher ETS during the early forecast hours. However, the relative humidity increments are excessive, and more spurious convections are triggered as the forecast moves forward, which results in a decline in forecast skill with precipitation.
Case 2 of 12 June 2018
From 0900 UTC to 1500 UTC, the accumulated precipitation was more than 50 mm in central Hebei and was more than 20 mm in southern Liaoning at 6 h (Figure 13a). CTL cannot forecast these two observed heavy precipitation centers (Figure 13b). For LDA_T020, the precipitation forecast is less than the observed precipitation in central Hebei, and the precipitation forecast is relatively strong in southern Liaoning (Figure 13c). Both LDA_CTH (Figure 13d) and LDA_15km (Figure 13e) can forecast the heavy precipitation in central Hebei, and the forecasted precipitation in the area is slightly weaker than the observed precipitation. In Figure 7d, the CTH is more than 15 km in only central Shanxi, central Hebei, Beijing and southern Liaoning and less than 15 km in northeastern Inner Mongolia and northern Liaoning. The adjustment of the relative humidity may be excessive in LDA_15km. This result may be the reason why there is a spurious precipitation forecast in northern Liaoning and northeastern Inner Mongolia in LDA_15km.
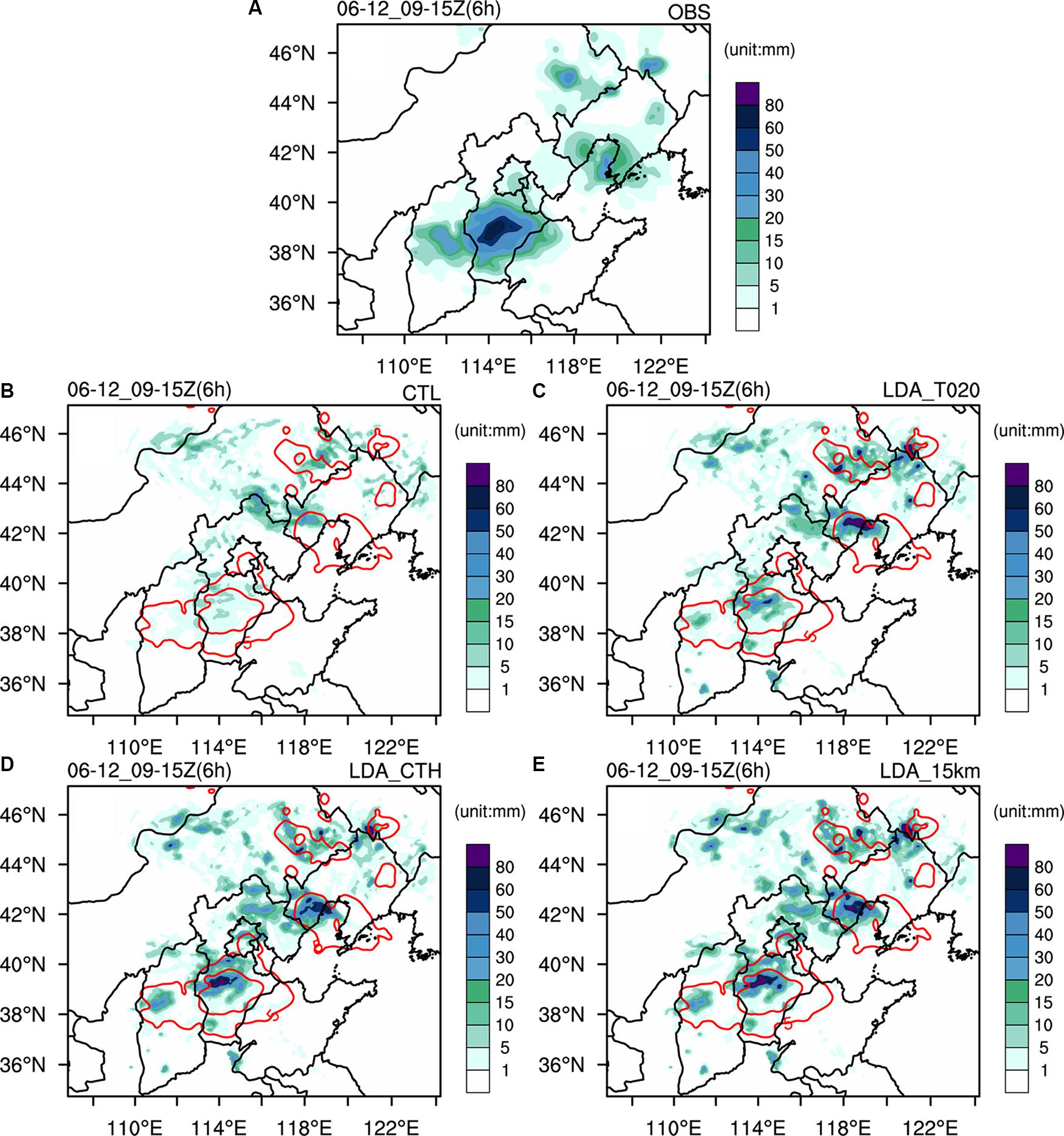
Figure 13. As in Figure 11, but for case 2 from 0900 UTC to 1500 UTC on 12 June 2018 for each experiment. (A) Observations (OBS), (B) CTL, (C) LDA_T020, (D) LDA_CTH, and (E) LDA_15km.
The spatial distribution of the observed and simulated 6-h accumulated precipitation indicates that LDA_T020 is slightly improved compared with CTL, and LDA_CTH and LDA_15km can forecast the central heavy precipitation that fails to be forecasted by CTL. Based on CTH as the upper limit of water vapor adjustment, LDA_CTH can reduce the phenomenon of spurious strong precipitation forecasts in northeastern Inner Mongolia and northern Liaoning. The results of the skill score for 1 h accumulated precipitation show that LDA_CTH and LDA_15km are close to each other at the 1 mm threshold, which improves the precipitation forecast in the first 5 h (Figure 14a,c). The improvement is not obvious in LDA_T020 because it fails to predict strong precipitation in central Hebei. For the 5 mm threshold, the ETS of LDA_CTH is better than that of LDA_15km in the first 2 h (Figures 14b,d). Although the improvement is not strong, this improvement provides a new idea for further improving the prediction of convective heavy precipitation.
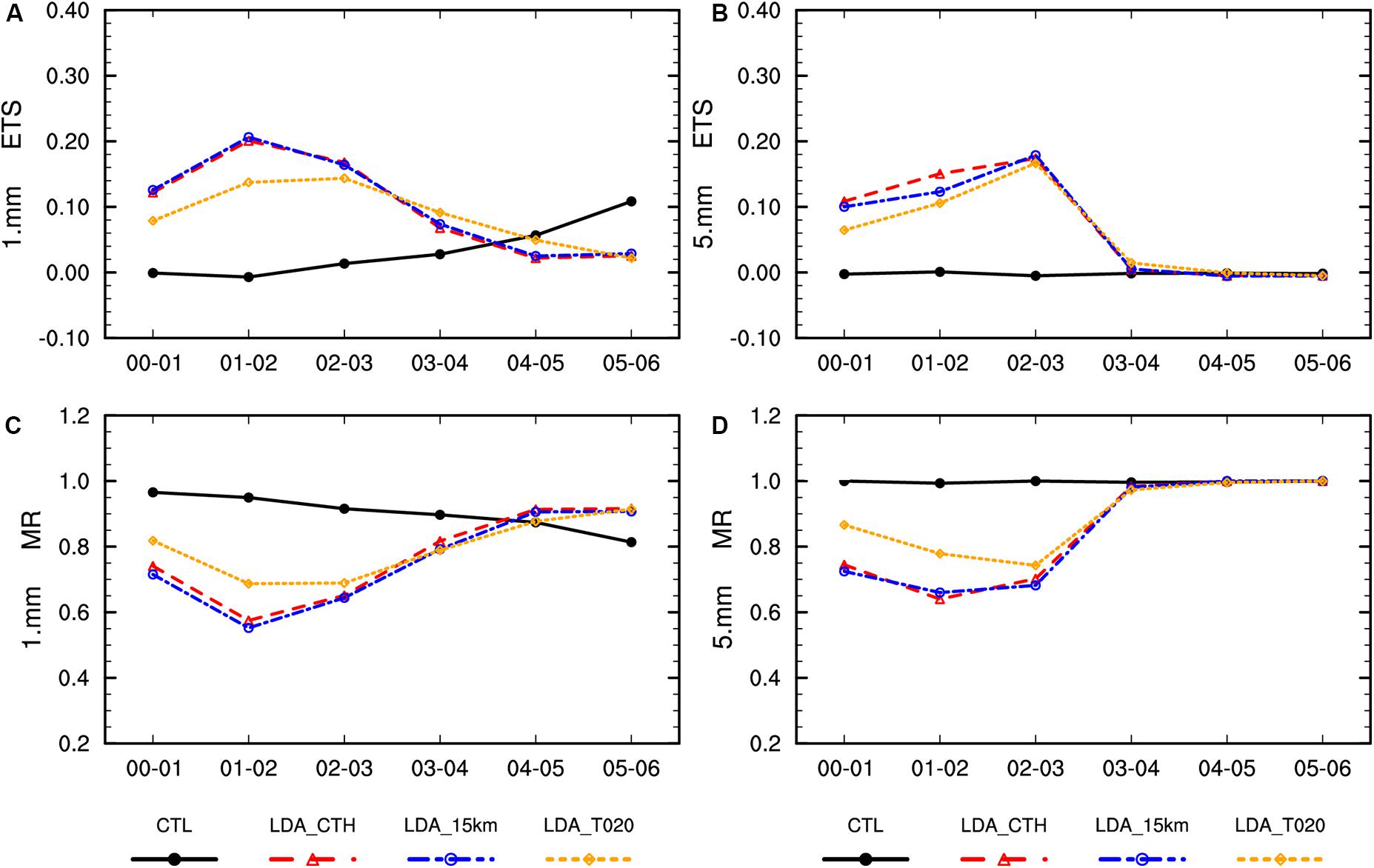
Figure 14. As in Figure 12, but for case 2 from 0900 UTC to 1500 UTC on 12 June 2018 for each experiment. (A) ETS for 1 mm, (B) ETS for 5 mm, (C) MR for 1 mm, (D) MR for 5 mm.
Conclusion and Discussion
An efficient LDA method was proposed that combines the application of FY4 LMI data with FY4 CTH observations. In this LDA method, we attempt to properly determine the adjustment range of water vapor in the vertical direction and obtain pseudo-observations with improve accuracy. According to the water vapor conditions in the cloud at the time of lightning occurrence, CTH and LCL are used as the lower and upper boundaries of the water vapor adjustment. The relative humidity pseudo-observations are obtained based on FY4 LMI and are then assimilated in the model through the WRF 3DVAR method. Two severe convection events with different characteristics were studied to evaluate the data assimilation approach for short-term precipitation forecast. Two other vertical adjustment schemes were performed to find the impact of different adjustment schemes in the vertical direction on the convective environment. The water vapor increments in the analysis field and the wind field, temperature field and hydrometer field in the forecast are analyzed in detail for case 1, and the forecasted hourly precipitation for case 1 and case 2 are quantitatively verified using observed precipitation data.
The results show that the assimilations of relative humidity pseudo-observations created with different adjustment schemes produce different water vapor increments at the analysis time, which can directly change wind, temperature and hydrometer distributions in the subsequent forecasts. Unsurprisingly, the assimilations also produce different precipitation forecasts. (1) The analyzed relative humidity increments in LDA_T020 are much smaller than those for the other two LDA experiments. Therefore, the forecast result for the two cases is close to the control run, although the precipitation forecast is improved slightly over the CTL. (2) The assimilation experiment in LDA_15km introduces the maximum relative humidity increments at the analysis time, and the updraft in the forecast field is also stronger than that with LDA_T020 and LDA_CTH. Therefore, the forecasted precipitation is relatively strong. However, LDA_15km also produces a strong spurious precipitation area in northwest Jiangxi Province for case 1 and northeast Inner Mongolia and northern Liaoning for case 2. The skill scores of the 1-h precipitation forecast of LDA_15km are close to those of LDA_CTH. The ETS of the 5 mm threshold is lower for the 3-h forecast in case 1 and is lower for the first 2-h forecast in case 2. (3) The relative humidity increments in LDA_CTH change with longitude over the analysis time, which is an expected feature. The wind field converges at a low level and diverges at a high level. The updraft is enhanced, and moist air is lifted. The condensation of water vapor releases latent heat as expected, and the hydrometer masses increase at the vertical position where lightning occurs. From the spatial distribution of precipitation in case 1 and case 2, the pattern of 6-h accumulated precipitation is most similar to the observations. There is an improvement of ETS for the 5 mm threshold for the forecast in the later 3 h in case 1 and in the forecast for the first 2 h in case 2. In this study, only two severe convection events were evaluated the data assimilation approach for short-term precipitation forecast, which may have a limitation for the conclusions. In order to obtain more accurate and reliable conclusion, we will conduct LDA studies for different convective events (such as squall lines, tropical cyclones, etc.) in the subsequent work.
This study adjusts the relative humidity using simple adjustment schemes in which the relative humidity of all adjustment layers is adjusted to a fixed value (90%) to analyze the influence of different adjustment ranges along the vertical direction on the assimilation results and to illustrate the importance of the vertical distribution of the relative humidity in deep moist convections. In the LDA, the other proxy variables (temperature profile, radar reflectivity, graupel mixing ratio, and so on) of lightning can be assimilated into the model through different assimilation methods (nudging, 3DVAR, and EnKF). For example, Marchand and Fuelberg (2014) used temperature as a proxy variable, Wang et al. (2014) used radar reflectivity as a proxy variable, and Wang H. et al. (2017) used graupel mixing as a proxy variable. However, most LDA experiments assimilate only one proxy variable when assimilating water vapor without temperature assimilation, which may introduce only minor changes in the overall convective environment. For example, although the content of the ice-phase particles is directly adjusted at the analysis time with an environment that is not conducive to the formation of ice-phase particles, the ice-phase particles will gradually dissipate as the model integration moves forward. By assimilating several of the proxy variables for lightning, a dynamic and thermodynamic environment conducive to the formation of ice-phase particles can be obtained, and the ice-phase particle content in the environment is adjusted through the development of the microphysical process in the model. Thus, an ice-phase particle content consistent with the occurrence of lightning is obtained. Of course, adjustments to multiple variables come with the risk of producing more spurious convection, but this is difficult to predict without thorough testing. Simultaneously, lightning data may help reduce placement errors for convective storms, but more studies are needed to confirm these results.
Data Availability Statement
The raw data supporting the conclusions of this article will be made available by the authors, without undue reservation. The NCEP GFS analysis data are available from the NCAR Research Data Archive (RDA, https://rda.ucar.edu/datasets/ds084.1/index.html#Wdescription).
Author Contributions
PL and YY: conceptualization, data curation, and methodology. PL: investigation and writing original draft. YY: project administration. PL, YY, JG, YW, and CW: writing – review and editing.
Funding
This work was supported by the National Key Research and Development Program of China (No. 2017YFC1502102) and the National Natural Science Foundation of China (No. 41175092).
Conflict of Interest
The authors declare that the research was conducted in the absence of any commercial or financial relationships that could be construed as a potential conflict of interest.
Acknowledgments
We thank the Chinese National Satellite Meteorological Center (NSMC) for providing the FY4 lightning data and FY4 cloud top height data (http://satellite.nsmc.org.cn/PortalSite/Data/Satellite.aspx) and the Chinese National Meteorological Information Center (NMIC) for providing site precipitation observations (http://data.cma.cn/data/detail/dataCode/A.0012.0001.html) and Land Data Assimilation System version 2.0 (CLDAS v2.0) precipitation product (http://data.cma.cn/data/cdcdetail/dataCode/NAFP_CLDAS2.0_RT.html). The NCEP GFS analysis data are available from the NCAR Research Data Archive (RDA, https://rda.ucar.edu/datasets/ds084.1/index.html#!description). We also wish to thank Shuang Xi and He Huang for beneficial guidance on FY4 cloud top height and site observation process, respectively.
References
Alexander, G. D., Weinman, J. A., Karyampudi, V., Olson, W. S., and Lee, A. C. L. (1999). The effect of assimilating rain rates derived from satellites and lightning on forecasts of the 1993 superstorm. Mon. Weather Rev. 127, 1433–1457. doi: 10.1175/1520-04931999127<1433:teoarr<2.0.co;2
Allen, B. J., Mansell, E. R., Dowell, D. C., and Deierling, W. (2016). Assimilation of pseudo-GLM data using the ensemble Kalman filter. Mon. Weather Rev. 144, 3465–3486. doi: 10.1175/mwr-d-16-0117.1
Anagnostou, E. N., and Chronis, T. G. (2007). “The worth of long-range lightning observations on overland satellite rainfall estimation,” in Measuring Precipitation From Space. Advances In Global Change Research, Vol. 28, eds V. Levizzani, P. Bauer, and F. J. Turk (Dordrecht: Springer).
Barker, D. M., Huang, W., Guo, Y. R., and Xiao, Q. N. (2004). A three-dimensional (3DVAR) data assimilation system for use with MM5: implementation and initial results. Mon. Weather Rev. 132, 897–914. doi: 10.1175/1520-0493(2004)132<0897:atvdas>2.0.co;2
Bielli, S., and Roux, F. (1999). Initialization of a cloud-resolving model with airborne doppler radar observations of an oceanic tropical convective system. Mon. Weather Rev. 127, 1038–1055. doi: 10.1175/1520-04931999127<1038:ioacrm<2.0.co;2
Brill, K. F., and Mesinger, F. (2009). Applying a general analytic method for assessing bias sensitivity to bias-adjusted threat and equitable threat scores. Weather Forecast. 24, 1748–1754. doi: 10.1175/2009WAF2222272.1
Chang, D. E., Weinman, J. A., Morales, C. A., and Olson, W. S. (2001). The effect of spaceborne microwave and ground-based continuous lightning measurements on forecasts of the 1998 Groundhog Day storm. Mon. Weather Rev. 129, 1809–1833. doi: 10.1175/1520-04932001129<1809:teosma<2.0.co;2
Cummins, K. L., Murphy, M. J., Bardo, E. A., Hiscox, W. L., Pyle, R. B., and Pifer, A. E. (1998). A Combined TOA/MDF technology upgrade of the U.S. National lightning detection network. J. Geophys. Res. Atmos. 103, 9035–9044. doi: 10.1029/98jd00153
Dudhia, J. (1996). “A multi-layer soil temperature model for MM5,” in The 6th PSU/NCAR Mesoscale Model Users Workshop, At Bouilder, CO, 49–50.
Fierro, A. O., Gao, J., Ziegler, C. L., Calhoun, K. M., Mansell, E. R., and MacGorman, D. R. (2016). Assimilation of flash extent data in the variational framework at convection-allowing scales: proof-of-concept and evaluation for the short-term forecast of the 24 may 2011 tornado outbreak. Mon. Weather Rev. 144, 4373–4393. doi: 10.1175/mwr-d-16-0053.1
Fierro, A. O., Gao, J., Ziegler, C. L., Mansell, E. R., MacGorman, D. R., and Dembek, S. R. (2014). Evaluation of a cloud-scale lightning data assimilation technique and a 3dvar method for the analysis and short-term forecast of the 29 June 2012 Derecho Event. Mon. Weather Rev. 142, 183–202. doi: 10.1175/mwr-d-13-00142.1
Fierro, A. O., Gilmore, M. S., Mansell, E. R., Wicker, L. J., and Straka, J. M. (2006). Electrification and lightning in an idealized boundary-crossing supercell simulation of 2 June 1995∗. Mon. Weather Rev. 134, 3149–3172. doi: 10.1175/mwr3231.1
Fierro, A. O., Mansell, E. R., Ziegler, C. L., and MacGorman, D. R. (2012). Application of a lightning data assimilation technique in the WRF-ARW model at cloud-resolving scales for the tornado outbreak of 24 May 2011. Mon. Weather Rev. 140, 2609–2627. doi: 10.1175/mwr-d-11-00299.1
Fierro, A. O., and Reisner, J. M. (2011). High-resolution simulation of the electrification and lightning of hurricane rita during the period of rapid intensification. J. Atmos. Sci. 68, 477–494. doi: 10.1175/2010jas3659.1
Gao, J., Smith, T. M., Stensrud, D. J., Fu, C., Calhoun, K., Manross, K. L., et al. (2013). A Real-time weather-adaptive 3DVAR analysis system for severe weather detections and warnings. Weather Forecast. 28, 727–745. doi: 10.1175/waf-d-12-00093.1
Gao, J., and Stensrud, D. J. (2012). Assimilation of reflectivity data in a convective-scale, cycled 3DVAR framework with hydrometeor classification. J. Atmos. Sci. 69, 1054–1065. doi: 10.1175/jas-d-11-0162.1
Gao, J., Xue, M., Brewster, K., and Droegemeier, K. K. (2004). A three-dimensional variational data analysis method with recursive filter for doppler radars. J. Atmos. Oceanic Technol. 21, 457–469. doi: 10.1175/1520-04262004021<0457:atvdam<2.0.co;2
Ge, G., Gao, J., and Xue, M. (2013). Impacts of assimilating measurements of different state variables with a simulated supercell storm and three-dimensional variational Method. Mon. Weather Rev. 141, 2759–2777. doi: 10.1175/MWR-D-12-00193.1
Giannaros, T. M., Kotroni, V., and Lagouvardos, K. (2016). WRF-LTNGDA: a lightning data assimilation technique implemented in the WRF model for improving precipitation forecasts. Environ. Model. Softw. 76, 54–68. doi: 10.1016/j.envsoft.2015.11.017
Goodman, S. J., Buechler, D. E., Wright, P. D., and Rust, W. D. (1988). Lightning and precipitation history of a microburst-producing storm. Geophys. Res. Lett. 15, 1185–1188. doi: 10.1029/gl015i011p01185
Hong, S., and Lim, J. O. J. (2006). The WRF single-moment 6 class microphysics scheme (WSM6). J. Korean Meteorol. Soc. 42, 129–151.
Hong, S., Noh, Y., and Dudhia, J. (2006). A new vertical diffusion package with an explicit treatment of entrainment processes. Mon. Weather Rev. 134, 2318–2341. doi: 10.1175/mwr3199.1
Iacono, M. J., Delamere, J. S., Mlawer, E. J., Shephard, M. W., Clough, S. A., and Collins, W. D. (2008). Radiative forcing by long-lived greenhouse gases: calculations with the AER radiative transfer models. J. Geophys. Res. 113:D13103. doi: 10.1029/2008jd009944
Ide, K., Courtier, P., Ghil, M., and Lorenc, A. C. (1997). Unified notation for data assimilation: operational, sequential and variational. J. Meteorol. Soc. Jpn Ser. II 75, 181–189. doi: 10.2151/jmsj1965.75.1b_181
Kain, J. S. (2004). The Kain–Fritsch convective parameterization: an update. J. Appl. Meteorol. 43, 170–181. doi: 10.1175/1520-04502004043<0170:tkcpau<2.0.co;2
Kain, J. S., and Fritsch, J. M. (1990). A one-dimensional entraining/detraining plume model and its application in convective parameterization. J. Atmos. Sci. 47, 2784–2802. doi: 10.1175/1520-0469(1990)047<2784:aodepm>2.0.co;2
Lagouvardos, K., Kotroni, V., Defer, E., and Bousquet, O. (2013). Study of a heavy precipitation event over southern France, in the frame of HYMEX project: observational analysis and model results using assimilation of lightning. Atmos. Res. 134, 45–55. doi: 10.1016/j.atmosres.2013.07.003
Lai, A., Gao, J., Koch, S. E., Wang, Y., Pan, S., Fierro, A. O., et al. (2019). Assimilation of radar radial velocity, reflectivity and pseudo water vapor for convective-scale nwp in a variational framework. Mon. Weather Rev. 147, 2877–2900. doi: 10.1175/mwr-d-18-0403.1
Liu, D., Qie, X., and Feng, G. (2010). Evolution characteristics of the lightning and the relation with dynamical structure in a mesoscale convective system over north china. Chin. J. Atmos. Sci. 34, 95–104. doi: 10.3724/SP.J.1037.2010.00186
Liu, J. G., Shi, C. X., and Sun, S. (2019). Improving land surface hydrological simulations in China using CLDAS meteorological forcing data. J. Meteorol. Res. 33, 1194–1206. doi: 10.1007/s13351-019-9067-0
MacGorman, D. R., Burgess, D. W., Mazur, V., Rust, W. D., Taylor, W. L., and Johnson, B. C. (1989). Lightning rates relative to tornadic storm evolution on 22 May 1981. J. Atmos. Sci. 46, 221–251. doi: 10.1175/1520-04691989046<0221:lrrtts<2.0.co;2
Mansell, E. R. (2014). Storm-scale ensemble kalman filter assimilation of total lightning flash-extent data. Mon. Weather Rev. 142, 3683–3695. doi: 10.1175/mwr-d-14-00061.1
Mansell, E. R., Ziegler, C. L., and MacGorman, D. R. (2007). A lightning data assimilation technique for mesoscale forecast models. Mon. Weather Rev. 135, 1732–1748. doi: 10.1175/mwr3387.1
Marchand, M. R., and Fuelberg, H. E. (2014). Assimilation of Lightning data using a nudging method involving low-level warming. Mon. Weather Rev. 142, 4850–4871. doi: 10.1175/mwr-d-14-00076.1
Papadopoulos, A., Chronis, T. G., and Anagnostou, E. N. (2005). improving convective precipitation forecasting through assimilation of regional lightning measurements in a mesoscale model. Mon. Weather Rev. 133, 1961–1977. doi: 10.1175/mwr2957.1
Papadopoulos, A., Serpetzoglou, E., and Anagnostou, E. N. (2009). Evaluating the impact of lightning data assimilation on mesoscale model simulations of a flash flood inducing storm. Atmos. Res. 94, 715–725. doi: 10.1016/j.atmosres.2009.05.008
Parrish, D. F., and Derber, J. C. (1992). The national meteorological center’s spectral statistical-interpolation analysis system. Mon. Weather Rev. 120, 1747–1763. doi: 10.1175/1520-04931992120<1747:tnmcss<2.0.co;2
Pessi, A. T., and Businger, S. (2009). The impact of lightning data assimilation on a winter storm simulation over the north pacific ocean. Mon. Weather Rev. 137, 3177–3195. doi: 10.1175/2009mwr2765.1
Petersen, W. A., and Rutledge, S. A. (1998). On the relationship between cloud-to-ground lightning and convective rainfall. J. Geophys. Res. Atmos. 103, 14025–14040. doi: 10.1029/97jd02064
Price, C., and Rind, D. (1992). A simple lightning parameterization for calculating global lightning distributions. J. Geophys. Res. Atmos. 97, 9919–9933. doi: 10.1029/92jd00719
Pu, Z., Li, X., and Sun, J. (2009). Impact of airborne doppler radar data assimilation on the numerical simulation of intensity changes of hurricane dennis near a landfall. J. Atmos. Sci. 66, 3351–3365. doi: 10.1175/2009jas3121.1
Qie, X., Guo, C., Yan, M., and Zhang, G. (1993). Lightning data and study of thunderstorm nowcasting. Acta Meteorol. Sin. 7, 244–256.
Qie, X., Zhu, R., Yuan, T., Wu, X., Li, W., and Liu, D. (2014). Application of total-lightning data assimilation in a mesoscale convective system based on the WRF model. Atmos. Res. 14, 255–266. doi: 10.1016/j.atmosres.2014.04.012
Schultz, C. J., Petersen, W. A., and Carey, L. D. (2011). Lightning and severe weather: a comparison between total and cloud-to-ground lightning trends. Weather Forecast. 26, 744–755. doi: 10.1175/waf-d-10-05026.1
Shi, C. X., Jiang, L. P., and Zhang, T. (2014). Status and plans of CMA land data assimilation system (CLDAS) project. Geophys. Res. Abstracts. 16:EGU2014–EGU5671.
Skamarock, W. C., Klemp, J. B., Dudhia, J., Gill, D. O., Barker, D., Duda, M. G., et al. (2008). A Description of the Advanced Research WRF Version 3 (No. NCAR/TN-475+STR). Boulder, CO: University Corporation for Atmospheric Research.
Sun, J. (2005). Convective-scale assimilation of radar data: progress and challenges. Q. J. R. Meteorol. Soc. 131, 3439–3463. doi: 10.1256/qj.05.149
Sun, J., and Wang, H. (2013). Radar data assimilation with WRF 4D-Var. Part II: comparison with 3D-Var for a squall line over the U.S. Great plains. Mon. Weather Rev. 141, 2245–2264. doi: 10.1175/mwr-d-12-00169.1
Wang, H., Liu, Y., Cheng, W. Y. Y., Zhao, T., Xu, M., Liu, Y., et al. (2017). Improving lightning and precipitation prediction of severe convection using lightning data Assimilation with NCAR WRF-RTFDDA. J. Geophys. Res. Atmos. 122, 12,296–12,316. doi: 10.1002/2017jd027340
Wang, Y., Yang, Y., Liu, D., Zhang, D., Yao, W., and Wang, C. (2017). A case study of assimilating lightning-proxy relative humidity with WRF-3DVAR. Atmosphere 8:55. doi: 10.3390/atmos8030055
Wang, H., Liu, Y., Zhao, T., Liu, Y., Xu, M., Shen, S., et al. (2018). Continuous assimilation of lightning data using time-lagged ensembles for a convection-allowing numerical weather prediction model. J. Geophys. Res. Atmos.. 123, 9652–9673. doi: 10.1029/2018jd028494
Wang, H., Sun, J., Fan, S., and Huang, X.-Y. (2013). Indirect assimilation of radar reflectivity with wrf 3d-var and its impact on prediction of four summertime convective events. J. Appl. Meteorol. Climatol. 52, 889–902. doi: 10.1175/jamc-d-12-0120.1
Wang, Y., Yang, Y., Zhang, F. M., and Yang, L. L. (2013). Improve the forecast of surface-layer wind in wind power farm with WRF-3DVAR. Adv. Mater. Res. 724-725, 480–484. doi: 10.4028/www.scientific.net/AMR.724-725.480
Wang, Y., Yang, Y., and Wang, C. (2014). Improving forecasting of strong convection by assimilating cloud-to-ground lightning data using the physical initialization method. Atmos. Res. 150, 31–41. doi: 10.1016/j.atmosres.2014.06.017
Wiens, K. C., Rutledge, S. A., and Tessendorf, S. A. (2005). The 29 June 2000 supercell observed during STEPS. Part II: lightning and charge structure. J. Atmos. Sci. 62, 4151–4177. doi: 10.1175/jas3615.1
Xiao, X., Wang, Y., Chen, M., and Gao, F. (2013). A mechanism analysis of the thermo-dynamical field of a suddenly intensifying storm from mountains in the Beijing area with the radar data 4DVar. Acta Meteorol. Sin. 71, 797–816. doi: 10.11676/qxxb2013.077
Yang, J., Zhang, Z., Wei, C., Lu, F., and Guo, Q. (2017). Introducing the new generation of chinese geostationary weather satellites, fengyun-4. Bull. Am. Meteorol. Soc. 98, 1637–1658. doi: 10.1175/bams-d-16-0065.1
Yang, Y., Wang, Y., and Zhu, K. (2015). Assimilation of chinese doppler radar and lightning data using WRF-GSI: a Case study of mesoscale convective system. Adv. Meteorol. 2015, 1–17. doi: 10.1155/2015/763919
Yi, X., Li, Z., Yao, X., Wang, H., and Sun, X. (2011). An analysis of the multi scale structure and evolution of a meso-scale occluding convective system. Acta Meteorol. Sin. 69, 249–262. doi: 10.3724/SP.J.1146.2006.01085
Keywords: FY4 lightning data, cloud top height, data assimilation, 3DVAR, humidity adjustment
Citation: Liu P, Yang Y, Gao J, Wang Y and Wang C (2020) An Approach for Assimilating FY4 Lightning and Cloud Top Height Data Using 3DVAR. Front. Earth Sci. 8:288. doi: 10.3389/feart.2020.00288
Received: 15 April 2020; Accepted: 22 June 2020;
Published: 10 July 2020.
Edited by:
Stefano Dietrich, National Research Council (CNR), ItalyReviewed by:
Theodore Giannaros, National Observatory of Athens, GreeceRoss Bannister, University of Reading, United Kingdom
Copyright © 2020 Liu, Yang, Gao, Wang and Wang. This is an open-access article distributed under the terms of the Creative Commons Attribution License (CC BY). The use, distribution or reproduction in other forums is permitted, provided the original author(s) and the copyright owner(s) are credited and that the original publication in this journal is cited, in accordance with accepted academic practice. No use, distribution or reproduction is permitted which does not comply with these terms.
*Correspondence: Yi Yang, yangyi@lzu.edu.cn