Abstract
Extreme heat events are associated with spikes in mortality, yet death rates are on average highest during the coldest months of the year. Under the assumption that most winter excess mortality is due to cold temperature, many previous studies have concluded that winter mortality will substantially decline in a warming climate. We analyzed whether and to what extent cold temperatures are associated with excess winter mortality across multiple cities and over multiple years within individual cities, using daily temperature and mortality data from 36 US cities (1985–2006) and 3 French cities (1971–2007). Comparing across cities, we found that excess winter mortality did not depend on seasonal temperature range, and was no lower in warmer vs. colder cities, suggesting that temperature is not a key driver of winter excess mortality. Using regression models within monthly strata, we found that variability in daily mortality within cities was not strongly influenced by winter temperature. Finally we found that inadequate control for seasonality in analyses of the effects of cold temperatures led to spuriously large assumed cold effects, and erroneous attribution of winter mortality to cold temperatures. Our findings suggest that reductions in cold-related mortality under warming climate may be much smaller than some have assumed. This should be of interest to researchers and policy makers concerned with projecting future health effects of climate change and developing relevant adaptation strategies.
Export citation and abstract BibTeX RIS

Content from this work may be used under the terms of the Creative Commons Attribution 3.0 licence. Any further distribution of this work must maintain attribution to the author(s) and the title of the work, journal citation and DOI.
Introduction
While the importance of seasonal patterns for human health has been recognized since antiquity (Hippocrates, 400 BCE), understanding of the causal role of ambient temperature in these associations remains incomplete. It is well established that extreme heat can lead to increases in daily death counts in cities, with little doubt as to causality (Semenza et al 1996, Basu and Samet 2002, Vandentorren et al 2004, Anderson and Bell 2009). There is also evidence that extreme cold spells can lead to adverse cardiac events and death (Teng and Heyer 1955, Huynen et al 2001). What remains unclear, however, is whether cold temperature is a direct, proximal cause for the general elevation in death rates during the winter season in developed countries where heating is largely available. Clarifying whether temperature is a proximal cause of winter season excess mortality is key for understanding future impacts of climate change. If cold is responsible for a substantial fraction of winter mortality, then future warming would be expected to lead to substantial reductions in winter mortality. However, if seasonal factors other than temperature are mainly responsible for winter excess mortality, then climate warming might have little benefit.
There is a large and growing body of research literature and government reports which projects future temperature-related deaths under climate change scenarios, with a range of findings (Langford and Bentham 1995, Martens 1998, Donaldson et al 2001, Keatinge 2002, Hayashi et al 2009, Huang et al 2012, Martin et al 2012, Vardoulakis and Heaviside 2012, Li et al 2013). Some analyses have predicted that increasing heat-related deaths will outweigh cold-related decreases, leading to increasing net mortality effects in future climates (Doyon et al 2008, Dear 2011, Li et al 2013). Other studies report the opposite, i.e. that decreases in cold-related deaths will outweigh heat-related increases (Langford and Bentham 1995, Martens 1998, Hayashi et al 2009, Martin et al 2012, Vardoulakis and Heaviside 2012).
A common approach to quantifying the effects of temperature on mortality is to analyze daily observations over time in a given city. A key challenge faced by these studies is separating temperature effects from seasonal effects, since temperature and season are very highly correlated across seasons. This latter challenge is crucial to studies that seek to fit exposure-response functions (ERFs) linking temperature with mortality, independent of seasonal effects. Such studies contribute substantially to our current understanding of the health effects of both hot and cold temperatures.
We suggest that underlying the wide range of findings regarding future climate impacts is a fundamental difference in implicit assumptions regarding the causal role of cold temperatures in winter season mortality, assumptions that derive in turn from subtle but critical differences in the statistical models used to estimate cold temperature effects in the presence of seasonal confounding. Studies that attribute large numbers of deaths to cold temperatures are based on statistical models that fit cold temperature effects using some form of multi-week moving average of lagged temperature (usually 21 days) (Anderson and Bell 2009, Gasparrini et al 2015). Studies that attribute small numbers of deaths to cold temperatures are based on statistical models that fit cold temperature effects using short lags (1–7 days) (Medina-Ramón and Schwartz 2007, Li et al 2013). Because long moving averages of temperature are highly correlated with seasonal patterns of mortality, estimating the mortality effects of long temperature lags can lead to multi-colinearity and residual confounding by season (Huang and Barnett 2014, Woodward 2014), even with seasonal variables included simultaneously in the model.
If cold temperatures were directly responsible for winter mortality, one might expect a more pronounced relative winter mortality excess in cities where winter to summer temperature differences are larger, or where winter temperatures are colder. We recently examined this issue by comparing Honolulu, HA and Detroit, MI and found a similar relative seasonal variation in mortality in Honolulu despite an order of magnitude smaller seasonal temperature difference (von Klot et al 2012). This observation implies either that seasonal variation of mortality is not primarily driven by temperature variations or that residents of Honolulu are highly adapted to their climate and are at risk of death when temperatures dip below 20°C. Indeed, the issue of adaptation to local climate is intimately connected to how one thinks about future mortality effects of changing temperatures.
Recent studies have questioned the potential future winter mortality benefits of warming temperatures (Ebi and Mills 2013, Staddon et al 2014). Using data from England and Wales spanning the period 1951–2011, Staddon and colleagues showed that cold temperature effects have largely disappeared in recent decades, and that there will not be future mortality benefits of climate warming. They suggest that improved housing and overall standards of living may have been responsible for the declining impact of cold. Based on a literature review, Ebi and Mills conclude that climate change is unlikely to result in a substantial reduction of winter mortality.
In the present paper we evaluate observational evidence for direct temperature effects on winter-season mortality by analyzing patterns over space and time of mortality and temperatures in a large set of cities in both the US and France. Our objective was to determine whether and to what extent cold temperatures are associated with excess winter mortality when examined across multiple cities and over multiple years within individual cities. Our focus here is only on direct effects of temperatures on mortality risk. We don't consider other pathways, such as storms, snowfall or ice, by which climate change could alter human health in winter.
Methods
This study emerged from a collaboration between environmental health researchers in France and the US and thus focused on readily available data from cities in those two countries.
Data Sources
Total mortality for all age groups were obtained from the French National Institute for Statistics and Economics Studies (INSEE) for Paris, Lyon and Marseille for the period 1971–2007.
Mortality data for 36 US cities (listed in supplemental table 1) were obtained from the US National Center for Health Statistics, and cover all non-accidental deaths (consistent with most previous studies).
For French cities, daily mean temperature data were obtained from Météo-France for a reference station (near city center in Paris and Lyon, and at the airport in Marseilles) for the period 1971–2007. US daily temperature data covering the years 1985–2006 were obtained from the National Climatic Data Center.
Data Analysis
We computed winter and summer seasonal mean temperatures and deaths in 39 cities and examined whether there were associations between winter/summer mortality ratios and seasonal temperature differences using graphical displays and ordinary linear regression. We compared winter season excess deaths (the ratio of December, January and February deaths to June, July and August deaths) to the difference in mean temperature between these two seasons and to mean winter season temperature across the 39 cities. To examine the importance of controlling for season when testing for temperature effects on mortality over time, we fit a penalized spline function to the relation between same day temperature and mortality in four representative cities: two with substantial seasonal temperature swings (Paris and New York City), one with a hot, humid climate (Miami), and one with intermediate climate (Marseille).
To examine temporal associations between temperature and mortality with or without control for season, first we aggregated data into time series of daily deaths and daily weather for each of the four cities noted above. To compare the effects of average daily mean temperature (Tmean) on mortality (Y) with different levels of control for season, we fit the Poisson regression model:
where k was set to 0, 6, or 8 respectively. For the smooth function of time, natural splines with k*(years of study) degrees of freedom were used. Yt is the time series of daily death counts for a city. Temperature was modeled using a 10 df spline function. The objective of these analyses was to explore the sensitivity of the fitted temperature function to differing amounts of seasonal adjustment (i.e., using different of freedom on the spline of time).
To further control of season, we used a similar Poisson regression model as above but stratified by month. The model took the form:
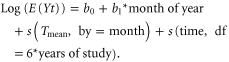
Yt is again the time series of daily death counts, but now stratified within months. In the first set of analyses, we used Tmean on the day of death. In sensitivity analyses, we used Tmean = average temperature over the five days ending on the day of death.
All analyses were carried out in R. A similar modeling framework was described in a previous study (Lee et al 2014).
Results
If warmer climate leads to less winter mortality, we would expect to see less winter excess mortality in cities that have less extreme winter–summer temperature variations, or that have warmer winters. Figure 1 plots the winter to summer mortality ratio against the seasonal temperature difference across the 39 cities, with each data point representing one city. Supplemental figure 1 plots the same mortality ratio against mean winter season temperature. A similar level of winter excess mortality was observed for all cities (mean 1.16; IQR: 1.14–1.18), while seasonal temperature differences spanned a four-fold range, and winter mean temperatures ranging from −5 to over 20 °C. There was no statistical correlation between the magnitude of winter excess mortality and the seasonal range of temperature (R2 = 0.01, p = 0.47).
Figure 1. Scatter plot of winter excess mortality (the ratio of December, January and February deaths to June, July, August deaths) versus seasonal temperature difference for the same months over 22 years in 39 cities. See supplemental file, table 1 for a list of cities and input data.
Download figure:
Standard image High-resolution imageIn figure 2 we plot penalized spline functions relating mortality with same day temperature in Paris, New York City, Miami, and Marseille. When season is left uncontrolled (black circles), cold effects appear much larger, and heat effects much smaller, than when season is controlled. (fitting splines of time with 6 and 8 degrees of freedom per year, as per (Peng et al 2006)).
Figure 2. Penalized spline functions fit to the relation between temperature and mortality in Paris and New York City (top), and Marseille and Miami (bottom). The black circles show the relationship when season is left uncontrolled. The red triangles show the relationship when season is controlled using a natural spline with six degrees of freedom per year, and the green crosses show the relation with eight degrees of freedom per year of seasonal control. The French data are for 1971–2007 and the US data are for 1985–2006.
Download figure:
Standard image High-resolution imagePenalized splines linking mortality with same-day temperature within calendar months for New York City, Paris, Miami and Marseille are shown in figure 3. There was little relationship evident between mortality and cold temperatures, except in January in Paris and Marseilles. Moreover, the increased mortality with cold weather occurred on the relatively small number of days below 0 °C in Paris, while more substantial increase in mortality for hot weather occurred on far more days when the mean temperature exceeded 20 °C. In sensitivity analyses, we repeated the above analyses using the average of temperature over the five days preceding death. The results (supplement figure 3) showed a larger effect of cold temperature, but still smaller, and spread over fewer days, than the heat effects in the prior figure.
Figure 3. Penalized spline functions relating mortality with temperature on the day of death by calendar month in Paris and New York City (top) and Marseille and Miami (bottom). The Paris and Marseille data are for 1971–2007 and the NYC and Miami data are for 1985–2006.
Download figure:
Standard image High-resolution imageDiscussion
Our results suggest that most winter-excess mortality is not largely driven by cold temperature. Among 39 cities in the US and France, there was no evidence that cities that have acclimatized to warm temperatures experience any less winter mortality than do cooler cities. These cities vary widely in demography, urban design, and socio-cultural background, all of which might influence exposure to outdoor temperature and related mortality risks. This is consistent with prior literature on winter excess mortality at the country level, which showed that warmer countries experience as much or more winter excess mortality as do colder countries (Healy 2003, Laake and Sverre 1996). Though cross-sectional in nature, this evidence is inconsistent with the notion that future warming would lead to diminished winter season mortality. The lack of correlation between seasonal temperature differences and winter season excess mortality suggests that winter excess mortality is driven mainly by seasonal factors other than temperature.
Our time series analyses of daily deaths in multiple cities demonstrated that exposure-response findings for cold temperatures were sensitive to analytical methods to control for season, an issue well illustrated in the published literature, where studies that used little or no seasonal control reported large cold temperature effects (Martens 1998, Gómez-Acebo et al 2010, Martin et al 2012), and those that used extensive seasonal control reported smaller cold temperature effects (Medina-Ramón and Schwartz 2007, Doyon et al 2008). Lack of, or inadequate, control for season leads to spuriously large estimated cold effects due to confounding by season.
A standard epidemiologic approach to control of confounding is to analyze associations of interest within narrow strata of the confounder, in this case season. Surprisingly, this concept has rarely been applied in the temperature-mortality literature. There was relatively little relationship evident between mortality and cold temperatures after stratifying by month, except on a small number of very cold days. These analyses confirmed a pattern of modest to no increase in mortality on cold days, more substantial increases in mortality on warm days, and a larger range and number of days for which heat related mortality occurs. Because cold effects may be spread over multiple days (Braga et al 2001), we repeated the above analyses using the average of temperature over the five days preceding death. The results (supplement figure 3) show a larger effect of cold temperature, but still smaller, and spread over fewer days, than the heat effects in the prior figure. While a 21 day distributed lag might show greater effects, that would obviously not be possible in an analysis stratified by month, which illustrates the difficulty of resolving such long lagged temperature effects from season.
In the more recent literature, the issue of confounding by season has re-emerged as a key challenge (Kinney et al 2012, Huang and Barnett 2014, Woodward 2014). While most time series studies include splines of time to control for seasonality, the estimated effects of temperature in the cold season can still be confounded by season if long lags are assumed. A long moving average of temperature is highly co-linear with seasonal variations in mortality, and the slope on distributed lagged temperature may capture a part of that seasonality, even when a separate spline of time is included simultaneously in the model. This is a classic problem of multi-colinearity in regression. Studies that report large cold temperature effects invariably have fit long distributed lags, e.g., 21 days (Analitis et al 2008, McMichael et al 2008, Anderson and Bell 2009, Guo et al 2012).
There is a growing need for projections of future mortality impacts of temperature under scenarios of climate change, both for economic assessments and for adaptation planning. These analyses usually follow a health impact assessment methodology which first fits an ERF to observed data in a given locale (expressing the functional relationship between temperature and mortality in a baseline period) and then applies the ERF to future temperature scenarios for that locale to compute future deaths. As noted, some assessments of this kind have projected very large reductions in winter deaths (Langford and Bentham 1995, Martens 1998, Hayashi et al 2009, Martin et al 2012, Vardoulakis and Heaviside 2012). We suggest that this is the case largely because the ERFs for cold temperatures used in these studies were estimated using methodologies that did not adequately control for seasonal confounding. We argue that fitting time series regression models using long distributed lags of temperature leads to spuriously large associations with cold temperatures and that estimated cold effects diminish markedly when season is controlled for completely.
If cold temperature per se is not directly responsible for most winter excess mortality, what is? Influenza is an important direct risk factor for winter mortality and also increases the risk of death due to subsequent cardiovascular events (Bainton et al 1978, Reichert et al 2004, Steinvil et al 2009, von Klot et al 2012). Notably, in an analysis of 40 years of mortality statistics in the US, Reichert and colleagues found a remarkable correspondence between temporal and age-specific patterns in influenza mortality and other death causes including ischemic heart disease (Reichert et al 2004), a finding supported in more recent work (von Klot et al 2012). These studies imply that influenza infections may underlie much of the winter excess in general mortality risk. One study hypothesized that observed winter season increases in plasma fibrinogen and other coagulation factors may be induced by respiratory infections via activation of the acute phase response (Woodhouse et al 1994). Studies have shown elevations in a range of cardiac risk factors during winter, including vasoconstriction and blood pressure, platelet counts, blood cholesterol levels, heart rate, plasma fibrinogen concentrations, platelet viscosity and peripheral vasoconstriction (Donaldson and Keatinge 1997, Mercer et al 1999, Pell and Cobbe 1999, Halonen et al 2010, Hong et al 2012, Schauble et al 2012). The extent to which these findings reflect direct effects of exposure to cold temperatures vs. infectious pathways remains unclear at present. Changes over time in seasonal patterns of cardiac mortality in the US have been hypothesized to reflect increased prevalence of winter heating and summer air conditioning (Seretakis et al 1997). Sorting out the role of temperature exposure in the range of factors influencing winter mortality risks is a major area for future research.
A range of seasonal factors other than cold, including overweight, lack of exercise, time spent indoors, school schedules, holiday gatherings, and air moisture content may lead to increased risks of respiratory infections during winter months (Pell and Cobbe 1999, Phillips et al 2004). Absolute humidity is one potential explanation for seasonality of respiratory infections because it varies substantially by season, with much lower levels in winter than summer both indoors and outdoors (Nguyen et al 2013). It has been shown that dry air increases the risk of influenza infection and probably other respiratory viruses (Shaman and Kohn 2009). Dry air exposure has been associated with cardiac arrhythmias in an adult cohort (Nguyen et al 2015). Another recent study showed that indoor and outdoor absolute humidity were highly correlated in winter in Boston (Nguyen et al 2013). In contrast, indoor and outdoor temperatures showed little correlation in winter. Since adults in developed countries spend more than 90% of their time indoors, and are largely protected in their daily lives from cold exposure via a range of infrastructure and personal adaptations, humidity may be a more plausible meteorological risk factor for winter season respiratory infections and related cardiovascular mortality. However, seasonal patterns of human exposure to dry air and respiratory viruses remain largely unexplored.
Several limitations should be kept in mind regarding our study. We did not disaggregate deaths into different causes, nor did we stratify by age, sex, ethnicity or economic status. Thus we were unable to assess the extent to which cold temperatures may differentially affect certain causes or population subgroups that may be more vulnerable. Also, temperatures were measured at only a single outdoor site in each city, which ignores spatial patterns of temperature exposure that might occur due to land surface characteristics including vegetation density.
Whatever the causal pathways that lead to winter excess mortality, in our view the evidence suggests that cold plays a limited direct role. Two recent studies have reached similar conclusions using different methods (Ebi and Mills 2013, Staddon et al 2014). The implications for assessing future health impacts of climate change are substantial. If winter-season excess mortality is not primarily caused by cold temperatures, future winter warming is likely to have far smaller health benefits than many previous studies have assumed.
Acknowledgments
PK and EP were supported by grants #NA10OAR4310212 from the US National Oceanic and Atmospheric Administration and from grant #P30 ES09089 from the US National Institute of Environmental Health Sciences. Additional support was provided by the Earth Institute at Columbia University.
Author Contribution
PK, JS, MP, and RV contributed to the research design, data analysis and paper writing. EP contributed data analysis. AT and SM provided input and edits on early drafts.
Competing Financial Interests
The authors declare no competing financial interests.